Track_genes_sig <- cds_subset_pt_res %>%top_n(n=10, morans_I) %>% pull(gene_short_name) %>% as.character()这句代码指定的是取前十,可以生成一句代码,我可以在一定区间内取值吗
时间: 2024-09-19 19:02:12 浏览: 36
该段R语言代码是用来从数据框`cds_subset_pt_res`中选择Moran's I指数最高的前十个基因(`morans_I`列),然后提取出对应的基因简名(`gene_short_name`),并转化为字符类型。如果你想在某个特定区间内选取基因,你可以稍微修改一下代码。例如,如果你想要选取I指数在某个范围内的所有基因,可以这样做:
```r
start_range <- 0.5 # 起始I指数值
end_range <- 0.8 # 结束I指数值
genes_in_range <- cds_subset_pt_res %>%
filter(morans_I >= start_range & morans_I <= end_range) %>%
select(gene_short_name) %>%
as.character()
# genes_in_range现在包含了在指定范围内的基因名称列表
```
这里,我们首先过滤出I指数在`start_range`和`end_range`之间的行,然后再提取基因名。请注意替换`start_range`和`end_range`为你实际需要的区间。
相关问题
> genes <- read.table("genes.tsv", header = TRUE) > row.names(genes) <- genes$ENSGeneID > genes <- genes[,-1] > barcodes <- read.table("barcodes.tsv") > colnames(barcodes) <- "cell_id" > mtx <- readMM("matrix.mtx") > exp_mtx <- as.data.frame(as.matrix(mtx)) > rownames(exp_mtx) <- rownames(genes[match(rownames(exp_mtx), rownames(genes)),]) Error in genes[match(rownames(exp_mtx), rownames(genes)), ] : 量度数目不对,是什么错误
这个错误可能是由于 `genes` 和 `exp_mtx` 的行数不匹配导致的。可以尝试检查一下 `genes.tsv` 文件中的基因数量是否与 `matrix.mtx` 文件中的基因数量一致,或者检查一下是否存在重复的基因。如果有重复的基因,需要对其进行去重操作。另外,还可以查看一下 `matrix.mtx` 文件中的基因是否都在 `genes.tsv` 文件中出现。
PCA_Plot_3=function (data,Annotation,VAR,Color) { # logcountdata row:genes,column: samples pca <- prcomp(data) pca_out<-as.data.frame(pca$x) df_out<- pca_out %>%tibble::rownames_to_column(var=VAR) %>% left_join(., Annotation) #df_out<- merge (pca_out,Annotation,by.x=0,by.y=0) # label_color<- factor(df_out[,group]) ggplot(df_out,aes_string(x="PC1",y="PC2")) +geom_point(aes_string(colour = Color)) } Deseq2_Deseq_function_2=function (Countdata,Coldata) { dds_fil <- DESeq2:: DESeqDataSetFromMatrix(countData =Countdata, colData = Coldata, design = ~Group) dds_fil_Deg<- DESeq2::DESeq(dds_fil) return(dds_fil_Deg) } pheatmap_singscore=function (pathways,data,Annotation) { Gene_select_anno= data[,colnames(data) %in% pathways] %>%t()%>%.[,rownames(Annotation)] # return(Gene_select_anno) # Anno_expression_data=Gene_select_anno[,c("SYMBOL",Group_select)] %>% as.data.frame() %>% distinct() %>% na.omit() # rownames(Anno_expression_data)=Anno_expression_data[,"SYMBOL"] # Annotation=group_anno["Gene_type"] # input= Anno_expression_data[,Group_select] # F2_pheatmap <- pheatmap::pheatmap(input, cellwigermline calling GATKdth = 10, cellheight = 12, scale = "row", # treeheight_row = 5, # show_rownames = T,show_colnames = T, # annotation_col= Annotation, # # annotation_row=Annotation, # annotation_legend=Label_def, # cluster_rows = T, cluster_cols = F,clustering_distance_rows = "euclidean") pheatmap::pheatmap(Gene_select_anno, cellwigermline=5, cellheight = 10,cellwidth = 10, scale = "row", treeheight_row = 5, show_rownames = T,show_colnames = F, annotation_col= Annotation, # annotation_row=Annotation, #annotation_legend=Label_def, cluster_rows = T, cluster_cols = F,clustering_distance_rows = "euclidean") } matrix.please<-function(x) { m<-as.matrix(x[,-1]) rownames(m)<-x[,1] m } 这是r语言的代码,告诉我每一条代码的作用和意义
PCA_Plot_3: 这个函数用来绘制主成分分析(PCA)的散点图。它接受四个参数:data(数据矩阵),Annotation(注释信息),VAR(行名),Color(颜色)。首先,它对数据进行主成分分析(prcomp函数),然后将主成分分析的结果转换为数据帧(pca_out)。接下来,它将行名转换为指定的VAR变量名,并将注释信息与主成分分析结果进行连接(left_join函数)。最后,它使用ggplot库绘制散点图,其中x轴表示PC1,y轴表示PC2,点的颜色由Color参数指定。
Deseq2_Deseq_function_2: 这个函数用于进行差异表达分析。它接受两个参数:Countdata(基因计数数据)和Coldata(样本注释信息)。首先,它使用DESeq2包中的DESeqDataSetFromMatrix函数将Countdata和Coldata转换为差异表达分析所需的数据集对象。然后,它使用DESeq2包中的DESeq函数进行差异表达分析,并返回结果。
pheatmap_singscore: 这个函数用于绘制热图。它接受三个参数:pathways(基因通路信息),data(基因表达数据),Annotation(注释信息)。首先,它从data中选择pathways对应的基因,并根据Annotation的行名对选定的基因进行筛选。然后,它使用pheatmap包中的pheatmap函数绘制热图,其中Gene_select_anno是待绘制的基因数据,Annotation用于注释列,cluster_rows参数表示是否对行进行聚类,clustering_distance_rows参数表示行聚类所使用的距离度量。
matrix.please: 这个函数用于将数据框转换为矩阵。它接受一个参数x(数据框),并将x的第一列作为行名,将x的其余列转换为矩阵。最后,它返回该矩阵。
阅读全文
相关推荐
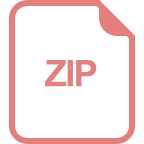
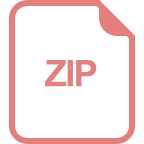












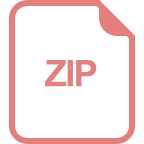
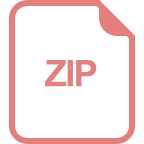
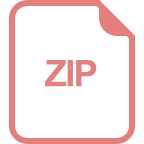