img_array = np.asarray(cropped_image) img = Image.fromarray(img_array)
时间: 2023-06-18 21:06:54 浏览: 190
这段代码的作用是将一个被裁剪后的图像转换为 numpy 数组,然后再将该数组转换为 PIL(Python Imaging Library)中的 Image 对象。
首先,`cropped_image` 可能是一个 PIL Image 对象,也可能是一个 numpy 数组,但无论哪种类型,都可以使用 `np.asarray()` 函数将其转换为 numpy 数组。这个数组可以在 NumPy 中进行处理和操作,例如对像素值进行数学运算或平滑处理等。
然后,使用 `Image.fromarray()` 函数将 numpy 数组转换为 PIL Image 对象。这允许我们使用 PIL 库中的其他函数和方法,例如调整图像大小、应用滤镜或保存到磁盘等。
相关问题
from PIL import Image, ImageDraw # 将图片平移并旋转 gray2 = Image.fromarray(src) width, height = gray2.size # 计算中心点和X轴角度 center = (max_point[0], max_point[1]) angle = np.arctan2(point2[1] - max_point[1], point2[0] - max_point[0]) * 180 / np.pi img_translated = gray2.transform((width, height), Image.AFFINE, (1, 0, center[0] - width/2, 0, 1, center[1] - height/2), resample=Image.BICUBIC) img_translated_rotated = img_translated.rotate(angle, resample=Image.BICUBIC, expand=True) #img_translated_rotated.show() #裁剪 img4 = Image.fromarray(src) width1, height1 = img4.size width2, height2 = img_translated_rotated.size left = (width2 - width1 )/2 top = (height2 - height1 )/2 right = (width2 - width1 )/2 + width1 bottom = (height2 - height1 )/2 + height1 cropped_image = img_translated_rotated.crop((left, top, right, bottom )) import cv2 GRID_STEP = distance/2 # 设置1010栅格(暂时尝试) grid_num_x = 10 grid_num_y = 10 def transform_point_set(points, max_point, distance, angle): # 平移向量 translation_vector = np.array([distance * np.cos(anglenp.pi/180), distance * np.sin(anglenp.pi/180)]) # 旋转矩阵 rotation_matrix = np.array([[np.cos(anglenp.pi/180), -np.sin(anglenp.pi/180)], [np.sin(anglenp.pi/180), np.cos(angle*np.pi/180)]]) # 将点集转换为 numpy 数组 point_array = np.array(points) max_point_array = np.array(max_point) # 对点集进行平移和旋转 point_array = (point_array - max_point_array) @ rotation_matrix + max_point_array + translation_vector # 将 numpy 数组转换为列表 points2 = point_array.tolist() return points2 points2 = transform_point_set(points, max_point, distance, angle) print(points2) #第2.5部分(用作确认检验) from PIL import Image, ImageDraw #裁剪 img4 = Image.fromarray(src) width1, height1 = img4.size width2, height2 = img_translated_rotated.size left = (width2 - width1 )/2 top = (height2 - height1 )/2 right = (width2 - width1 )/2 + width1 bottom = (height2 - height1 )/2 + height1 cropped_image = img_translated_rotated.crop((left, top, right, bottom )) # 导入图片() img_array = np.asarray(cropped_image) img = Image.fromarray(img_array) draw = ImageDraw.Draw(img) for point in point
这是一段 Python 代码,主要是对图片进行平移和旋转,并进行裁剪,然后将一组点坐标进行平移和旋转后输出。具体实现过程中,代码使用了 PIL 库和 OpenCV 库。首先通过 PIL 库中的 Image 类将源图片转换为灰度图像 gray2,然后计算出中心点和旋转角度,通过 transform 和 rotate 方法对图片进行平移和旋转操作。接着通过 crop 方法进行裁剪,将裁剪后的图片转换为 numpy 数组 img_array。最后使用 PIL 库中的 ImageDraw 类和 for 循环对一组点坐标进行平移和旋转,并输出平移和旋转后的点坐标。
from bm3d import bm3d_rgb from experiment_funcs1 import get_experiment_noise, get_psnr, get_cropped_psnr from PIL import Image import argparse import os import torch import numpy as np from torchvision.utils import save_image def main(): imagename = './test_image1/(1271).jpg' save_dir = 'test_result' save_path = 'noise' y = np.array(Image.open(imagename)) / 255 noise_type = 'g3' noise_var = 0.02 seed = 0 noise, psd, kernel = get_experiment_noise(noise_type, noise_var, seed, y.shape) z = np.atleast_3d(y) + np.atleast_3d(noise) y_est = bm3d_rgb(z, psd) psnr = get_psnr(y, y_est) print("PSNR:", psnr) y_est = np.minimum(np.maximum(y_est, 0), 1) z_rang = np.minimum(np.maximum(z, 0), 1) z_rang = torch.from_numpy(np.transpose(z_rang, (2, 0, 1))).float() y_est = torch.from_numpy(np.transpose(y_est, (2, 0, 1))).float() denoise_img_path = os.path.join(save_dir, 'denoised.jpg') save_image(y_est, denoise_img_path) noise_img_path = os.path.join(save_path, 'noise.jpg') save_image(z_rang, noise_img_path) if __name__ == '__main__': main()改为对灰度图处理
可以将代码中的`bm3d_rgb`函数改为`bm3d`函数,同时将读入图像的方式改为读入灰度图像即可。具体代码如下:
```
from bm3d import bm3d
from experiment_funcs1 import get_experiment_noise, get_psnr, get_cropped_psnr
from PIL import Image
import argparse
import os
import torch
import numpy as np
from torchvision.utils import save_image
def main():
imagename = './test_image1/(1271).jpg'
save_dir = 'test_result'
save_path = 'noise'
y = np.array(Image.open(imagename).convert('L')) / 255
noise_type = 'g3'
noise_var = 0.02
seed = 0
noise, psd, kernel = get_experiment_noise(noise_type, noise_var, seed, y.shape)
z = y + noise
y_est = bm3d(z, psd)
psnr = get_psnr(y, y_est)
print("PSNR:", psnr)
y_est = np.minimum(np.maximum(y_est, 0), 1)
z_rang = np.minimum(np.maximum(z, 0), 1)
z_rang = torch.from_numpy(z_rang).unsqueeze(0).float()
y_est = torch.from_numpy(y_est).unsqueeze(0).float()
denoise_img_path = os.path.join(save_dir, 'denoised.jpg')
save_image(y_est, denoise_img_path)
noise_img_path = os.path.join(save_path, 'noise.jpg')
save_image(z_rang, noise_img_path)
if __name__ == '__main__':
main()
```
阅读全文
相关推荐

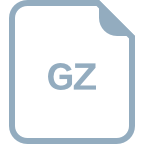















