解释这段代码# Def X and Y X = data.drop('Outcome', axis=1) y = data['Outcome'] X_train, X_test, y_train, y_test = train_test_split(X, y, train_size=0.8, shuffle=True, random_state=1) y_train = to_categorical(y_train) y_test = to_categorical(y_test)
时间: 2023-12-24 20:12:47 浏览: 20
这段代码用于对数据集进行处理,其中:
- `data` 是原始的数据集;
- `X` 是特征数据,通过 `drop` 方法删除了 `data` 中的 `Outcome` 列;
- `y` 是目标数据,即 `Outcome` 列;
- `train_test_split` 方法将数据集随机分割成训练集和测试集,其中 `train_size=0.8` 表示训练集占原始数据集的80%;
- `shuffle=True` 表示在分割数据集之前要先打乱数据集的顺序,`random_state=1` 表示打乱顺序的随机种子,可以保证每次运行程序时得到的结果是一样的;
- `to_categorical` 方法将目标数据集转化为多分类格式,以适应某些机器学习算法的要求。
相关问题
# Def X and Y X = data.drop('Outcome', axis=1) y = data['Outcome'] X_train, X_test, y_train, y_test = train_test_split(X, y, train_size=0.8, shuffle=True, random_state=1) y_train = to_categorical(y_train) y_test = to_categorical(y_test)这段代码是什么意思
这段代码主要是将数据集按照一定比例(这里是 80% 的训练集和 20% 的测试集)随机划分为训练集(X_train 和 y_train)和测试集(X_test 和 y_test),并对 y_train 和 y_test 进行独热编码。
具体来说,第一行代码通过 `drop()` 函数将数据集中的标签列(即 y 列)从数据集中去除,得到只包含特征的数据集 X。第二行代码则将数据集 X 和标签 y 按照 80:20 的比例随机划分成训练集和测试集,其中 `train_size=0.8` 表示训练集占总数据集的 80%,`shuffle=True` 表示在划分数据集时进行随机打乱,`random_state=1` 则是为了保证每次划分数据集的随机结果一致。
第三行代码使用 `to_categorical()` 函数将训练集的标签 y_train 进行独热编码,将其从原来的类别值转换为了一个长度为类别数目的向量,向量中只有一个元素为 1,其余均为 0,表示该样本属于这个类别。同理,第四行代码对测试集的标签 y_test 进行独热编码。
将这些代码转换为伪代码 # 确定目标变量和特征变量 target_col = ["Outcome"] cat_cols = data.nunique()[data.nunique() < 12].keys().tolist() cat_cols = [x for x in cat_cols] # numerical columns num_cols = [x for x in data.columns if x not in cat_cols + target_col] # Binary columns with 2 values bin_cols = data.nunique()[data.nunique() == 2].keys().tolist() # Columns more than 2 values multi_cols = [i for i in cat_cols if i not in bin_cols] # Label encoding Binary columns le = LabelEncoder() for i in bin_cols: data[i] = le.fit_transform(data[i]) # Duplicating columns for multi value columns data = pd.get_dummies(data=data, columns=multi_cols) # Scaling Numerical columns std = StandardScaler() scaled = std.fit_transform(data[num_cols]) scaled = pd.DataFrame(scaled, columns=num_cols) # dropping original values merging scaled values for numerical columns df_data_og = data.copy() data = data.drop(columns=num_cols, axis=1) data = data.merge(scaled, left_index=True, right_index=True, how="left") # 输出预处理后的数据集 print(data.head())
以下是转换为伪代码后的代码:
```
# 确定目标变量和特征变量
target_col = ["Outcome"]
cat_cols = 对数据集进行唯一值计数并筛选小于12的特征
cat_cols = [x for x in cat_cols]
# 数值型特征
num_cols = [x for x in 数据集的列名 if x not in cat_cols + target_col]
# 二分类特征
bin_cols = 对数据集进行唯一值计数并筛选等于2的特征
# 多分类特征
multi_cols = [i for i in cat_cols if i not in bin_cols]
# Label encoding 二分类特征
le = LabelEncoder()
for i in bin_cols:
对二分类特征进行标签编码
# 独热编码 多分类特征
data = pd.get_dummies(data=data, columns=multi_cols)
# 标准化 数值型特征
std = StandardScaler()
scaled = std.fit_transform(data[num_cols])
scaled = pd.DataFrame(scaled, columns=num_cols)
# 合并特征
df_data_og = data.copy()
data = data.drop(columns=num_cols, axis=1)
data = data.merge(scaled, left_index=True, right_index=True, how="left")
# 输出预处理后的数据集
print(data.head())
```
以上伪代码是对原 Python 代码的简化和抽象,将其转化为了一系列的操作和方法调用。
相关推荐
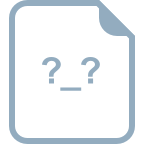
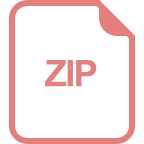
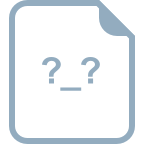












