将下列代码变为伪代码def median_target(var): temp = data[data[var].notnull()] temp = temp[[var, 'Outcome']].groupby(['Outcome'])[[var]].median().reset_index() return temp data.loc[(data['Outcome'] == 0 ) & (data['Insulin'].isnull()), 'Insulin'] = 102.5 data.loc[(data['Result'] == 1 ) & (data['Insulin'].isnull()), 'Insulin'] = 169.5 data.loc[(data['Result'] == 0 ) & (data['Glucose'].isnull()), 'Glucose'] = 107 data.loc[(data['Result'] == 1 ) & (data['Glucose'].isnull()), 'Glucose'] = 1 data.loc[(data['Result'] == 0 ) & (data['SkinThickness'].isnull()), 'SkinThickness'] = 27 data.loc[(data['Result'] == 1 ) & (data['SkinThickness'].isnull()), 'SkinThickness'] = 32 data.loc[(data['Result'] == 0 ) & (data['BloodPressure'].isnull()), 'BloodPressure'] = 70 data.loc[(data['Result'] == 1 ) & (data['BloodPressure'].isnull()), 'BloodPressure'] = 74.5 data.loc[(data['Result'] == 0 ) & (data['BMI'].isnull()), 'BMI'] = 30.1 data.loc[(data['Result'] == 1 ) & (data['BMI'].isnull()), 'BMI'] = 34.3 target_col = [“Outcome”] cat_cols = data.nunique()[data.nunique() < 12].keys().tolist() cat_cols = [x for x in cat_cols ] #numerical列 num_cols = [x for x in data.columns if x 不在 cat_cols + target_col] #Binary列有 2 个值 bin_cols = data.nunique()[data.nunique() == 2].keys().tolist() #Columns 2 个以上的值 multi_cols = [i 表示 i in cat_cols if i in bin_cols] #Label编码二进制列 le = LabelEncoder() for i in bin_cols : data[i] = le.fit_transform(data[i]) #Duplicating列用于多值列 data = pd.get_dummies(data = data,columns = multi_cols ) #Scaling 数字列 std = StandardScaler() 缩放 = std.fit_transform(数据[num_cols]) 缩放 = pd。数据帧(缩放,列=num_cols) #dropping原始值合并数字列的缩放值 df_data_og = 数据.copy() 数据 = 数据.drop(列 = num_cols,轴 = 1) 数据 = 数据.合并(缩放,left_index=真,right_index=真,如何 = “左”) # 定义 X 和 Y X = 数据.drop('结果', 轴=1) y = 数据['结果'] X_train, X_test, y_train, y_test = train_test_split(X, y, train_size=0.8, shuffle=True, random_state=1) y_train = to_categorical(y_train) y_test = to_categorical(y_test)
时间: 2024-02-26 11:51:43 浏览: 166
function median_target(var) {
temp = data[data[var].notnull()];
temp = temp[[var, 'Outcome']].groupby(['Outcome'])[[var]].median().reset_index();
return temp;
}
data.loc[(data['Outcome'] == 0) & (data['Insulin'].isnull()), 'Insulin'] = 102.5;
data.loc[(data['Outcome'] == 1) & (data['Insulin'].isnull()), 'Insulin'] = 169.5;
data.loc[(data['Outcome'] == 0) & (data['Glucose'].isnull()), 'Glucose'] = 107;
data.loc[(data['Outcome'] == 1) & (data['Glucose'].isnull()), 'Glucose'] = 1;
data.loc[(data['Outcome'] == 0) & (data['SkinThickness'].isnull()), 'SkinThickness'] = 27;
data.loc[(data['Outcome'] == 1) & (data['SkinThickness'].isnull()), 'SkinThickness'] = 32;
data.loc[(data['Outcome'] == 0) & (data['BloodPressure'].isnull()), 'BloodPressure'] = 70;
data.loc[(data['Outcome'] == 1) & (data['BloodPressure'].isnull()), 'BloodPressure'] = 74.5;
data.loc[(data['Outcome'] == 0) & (data['BMI'].isnull()), 'BMI'] = 30.1;
data.loc[(data['Outcome'] == 1) & (data['BMI'].isnull()), 'BMI'] = 34.3;
target_col = ["Outcome"];
cat_cols = data.nunique()[data.nunique() < 12].keys().tolist();
cat_cols = [x for x in cat_cols];
num_cols = [x for x in data.columns if x not in cat_cols + target_col];
bin_cols = data.nunique()[data.nunique() == 2].keys().tolist();
multi_cols = [i for i in cat_cols if i in bin_cols];
le = LabelEncoder();
for i in bin_cols:
data[i] = le.fit_transform(data[i]);
data = pd.get_dummies(data=data, columns=multi_cols);
std = StandardScaler();
scaled = std.fit_transform(data[num_cols]);
scaled = pd.DataFrame(scaled, columns=num_cols);
df_data_og = data.copy();
data = data.drop(columns=num_cols, axis=1);
data = data.merge(scaled, left_index=True, right_index=True, how='left');
X = data.drop('Outcome', axis=1);
y = data['Outcome'];
X_train, X_test, y_train, y_test = train_test_split(X, y, train_size=0.8, shuffle=True, random_state=1);
y_train = to_categorical(y_train);
y_test = to_categorical(y_test);
阅读全文
相关推荐
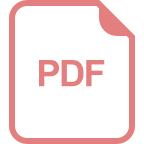















