python输出基于窗口的中文文本共词矩阵,并构建复杂网络,并构建可视化
时间: 2024-06-10 10:09:10 浏览: 8
抱歉,由于您的问题涉及到技术问题,我可以为您提供帮助。可以参考以下代码实现基于窗口的中文文本共词矩阵的构建和复杂网络的构建:
# 导入必要的库
import jieba
import numpy as np
import networkx as nx
import matplotlib.pyplot as plt
# 加载停用词
stop_words = set()
with open('stop_words.txt', 'r', encoding='utf-8') as f:
for line in f.readlines():
stop_words.add(line.strip())
# 加载语料
corpus = []
with open('corpus.txt', 'r', encoding='utf-8') as f:
for line in f.readlines():
corpus.append(line.strip())
# 分词
words_list = []
for c in corpus:
words = []
for w in jieba.cut(c):
if w not in stop_words and len(w) > 1:
words.append(w)
words_list.append(words)
# 构建共词矩阵
word_set = set()
for words in words_list:
word_set |= set(words)
word_list = list(word_set)
word_dict = {word: i for i, word in enumerate(word_list)}
co_matrix = np.zeros([len(word_list), len(word_list)])
for words in words_list:
for i in range(len(words)):
for j in range(i + 1, len(words)):
if words[i] in word_dict and words[j] in word_dict:
co_matrix[word_dict[words[i]], word_dict[words[j]]] += 1
co_matrix[word_dict[words[j]], word_dict[words[i]]] += 1
# 构建复杂网络
G = nx.Graph()
for i in range(len(word_list)):
for j in range(i + 1, len(word_list)):
if co_matrix[i, j] > 0:
G.add_edge(word_list[i], word_list[j], weight=co_matrix[i, j])
# 绘制可视化图像
pos = nx.spring_layout(G)
nx.draw(G, pos, with_labels=True, font_size=8, node_size=300, node_color='white', edge_color='gray', width=1)
edge_labels = nx.get_edge_attributes(G, 'weight')
nx.draw_networkx_edge_labels(G, pos, edge_labels=edge_labels, font_size=5)
plt.show()
相关推荐
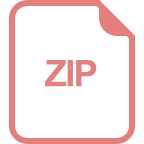
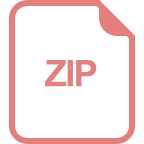
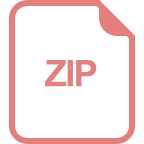














