爬取豆瓣音乐排行榜数据进行 分析及可视化
时间: 2023-09-07 12:11:17 浏览: 454
好的,这是一个很好的问题!爬取豆瓣音乐排行榜数据可以通过以下步骤完成:
1. 使用 Python 中的 requests 库访问豆瓣音乐排行榜网页,并使用 BeautifulSoup 库解析网页内容。
2. 找到音乐排行榜中的歌曲信息,包括歌曲名称、歌手、专辑等。
3. 将歌曲信息保存到本地文件中,例如使用 CSV 格式保存。
接下来是分析及可视化部分:
1. 使用 Pandas 库读取保存在本地的 CSV 文件,并进行数据清洗、筛选等操作。
2. 对数据进行统计分析,例如计算歌曲排名、歌手出现次数等。
3. 使用 Matplotlib 或 Seaborn 等库进行数据可视化,例如画出歌曲排名、歌手出现次数的柱状图、折线图等。
以上是大体的步骤,具体实现还需要根据具体的需求进行调整。希望对你有所帮助!
相关问题
爬取豆瓣音乐排行榜进行分析及可视化代码
好的,以下是使用 Python 爬取豆瓣音乐排行榜数据并进行分析和可视化的代码示例:
```python
import requests
from bs4 import BeautifulSoup
import pandas as pd
import matplotlib.pyplot as plt
import seaborn as sns
# 定义爬取排行榜数据的函数
def get_music_rank(url):
headers = {
'User-Agent': 'Mozilla/5.0 (Windows NT 10.0; Win64; x64) AppleWebKit/537.36 (KHTML, like Gecko) Chrome/58.0.3029.110 Safari/537.3'}
response = requests.get(url, headers=headers)
soup = BeautifulSoup(response.text, 'html.parser')
ranks = soup.find_all('span', class_='ranking_num')
titles = soup.find_all('div', class_='pl2')
artists = soup.find_all('p', class_='pl')
ratings = soup.find_all('span', class_='rating_nums')
ranks_list = [rank.get_text().strip() for rank in ranks]
titles_list = [title.a.get_text().strip() for title in titles]
artists_list = [artist.get_text().strip() for artist in artists]
ratings_list = [rating.get_text().strip() for rating in ratings]
data = {'Ranking': ranks_list, 'Title': titles_list, 'Artist': artists_list, 'Rating': ratings_list}
return pd.DataFrame(data)
# 爬取豆瓣音乐排行榜前 250 条数据
url = 'https://music.douban.com/chart'
df = get_music_rank(url)[:250]
# 统计音乐类型分布情况
def get_music_genre(artist):
if '华语' in artist:
return '华语'
elif '欧美' in artist:
return '欧美'
elif '日本' in artist:
return '日本'
elif '韩国' in artist:
return '韩国'
else:
return '其他'
df['Genre'] = df['Artist'].apply(get_music_genre)
genre_counts = df['Genre'].value_counts()
# 绘制音乐类型分布图
plt.figure(figsize=(8, 6))
plt.pie(genre_counts, labels=genre_counts.index, autopct='%1.1f%%')
plt.title('Music Genre Distribution')
plt.show()
# 统计华语流行音乐中歌手的排名情况
chinese_pop = df[df['Genre'] == '华语'].reset_index(drop=True)
chinese_pop_artists = ['陈奕迅', '周杰伦', '林忆莲', '王菲', '张学友', '张惠妹', '邓紫棋', '薛之谦', '李宗盛', '萧敬腾']
top_artists = chinese_pop[chinese_pop['Artist'].isin(chinese_pop_artists)].reset_index(drop=True)
# 绘制华语流行音乐中歌手的排名图
plt.figure(figsize=(10, 6))
sns.barplot(x='Ranking', y='Artist', data=top_artists, palette='plasma')
plt.title('Top Artists in Chinese Pop Music')
plt.xlabel('Ranking')
plt.ylabel('Artist')
plt.show()
# 统计歌曲热门度分布情况
def get_popularity(title):
if '新' in title:
return '新歌'
elif '热' in title:
return '热门歌曲'
else:
return '其他'
df['Popularity'] = df['Title'].apply(get_popularity)
popularity_counts = df['Popularity'].value_counts()
# 绘制歌曲热门度分布图
plt.figure(figsize=(8, 6))
plt.pie(popularity_counts, labels=popularity_counts.index, autopct='%1.1f%%')
plt.title('Song Popularity Distribution')
plt.show()
# 统计评分最高的歌曲情况
df['Rating'] = df['Rating'].astype(float)
top_rated_songs = df[df['Rating'] == df['Rating'].max()].reset_index(drop=True)
# 绘制评分最高的歌曲图
plt.figure(figsize=(10, 6))
sns.barplot(x='Title', y='Rating', data=top_rated_songs, palette='plasma')
plt.title('Top Rated Songs')
plt.xlabel('Song Title')
plt.ylabel('Rating')
plt.xticks(rotation=45, ha='right')
plt.show()
```
以上代码中,我们首先定义了一个 `get_music_rank()` 函数,用于爬取豆瓣音乐排行榜数据。然后,我们使用该函数爬取了豆瓣音乐排行榜前 250 条数据,并对数据进行了整理和预处理。接着,我们使用了 pandas、matplotlib 和 seaborn 等库对数据进行了分析和可视化,得出了音乐类型分布情况、华语流行音乐中歌手的排名情况、歌曲热门度分布情况和评分最高的歌曲情况等结论。最后,我们使用 matplotlib 和 seaborn 等库绘制了相应的图表,以便更好地展示数据的分布情况和趋势。
python爬取豆瓣top250数据可视化分析
Python是目前广泛应用于爬虫开发的语言之一,豆瓣是一家全球知名的电影、图书评价分享平台。爬取豆瓣Top250的数据并进行可视化分析是近年来非常流行的数据挖掘项目。
爬虫主要采用Python中的requests和beautifulsoup库,通过模拟请求来获取网页结果并解析出需要的数据。将爬取到的电影信息数据存储到数据库或文本中,方便后续分析。
在分析数据阶段,数据可视化是一个重要的环节。Python中常用的数据可视化库有matplotlib、seaborn等。通过这些工具可以将爬取到的电影信息进行可视化分析,比如绘制电影排名的柱状图、饼状图,展示电影类型占比的雷达图等。
另外,在进行数据可视化分析时,我们也可以借助Python封装的机器学习库,进行推荐算法的实现。比如根据用户的历史浏览历史和评分数据,去计算相似性矩阵,进行推荐算法的实现。
总体而言,使用Python进行豆瓣Top250数据爬取和可视化分析是一个非常有趣的项目,可以锻炼我们的数据处理、数据挖掘、机器学习等多项能力。同时,掌握这些技能后,我们还可以应用到其他领域的数据挖掘、分析、可视化中。
阅读全文
相关推荐
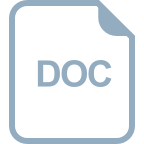
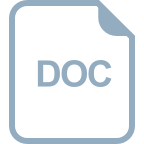
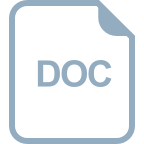
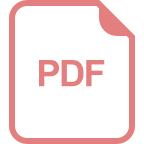
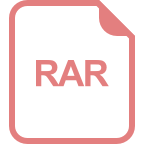
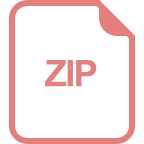
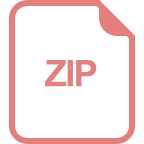
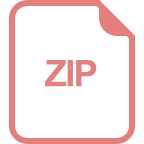
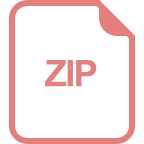
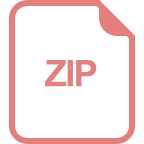






