能运行的输入为随机离散函数的LIF-STDP-SOM程序
时间: 2024-04-16 22:28:36 浏览: 18
下面是一个能够运行的 LIF-STDP-SOM(Leaky Integrate-and-Fire with Spike-Timing-Dependent Plasticity and Self-Organizing Map)的程序,它模拟了一个神经网络中的随机离散函数:
```python
import numpy as np
import matplotlib.pyplot as plt
from minisom import MiniSom
# LIF神经元类
class LIFNeuron:
def __init__(self, tau_m, tau_ref, tau_syn, V_rest, V_reset, V_th):
self.tau_m = tau_m # 膜时间常数
self.tau_ref = tau_ref # 绝对不应期时间常数
self.tau_syn = tau_syn # 突触后电流时间常数
self.V_rest = V_rest # 静息膜电位
self.V_reset = V_reset # 复位电位
self.V_th = V_th # 阈值电位
self.V = V_rest # 当前膜电位
self.t_ref = 0 # 当前不应期时间
def update(self, I, dt):
if self.t_ref <= 0:
dV_dt = (-(self.V - self.V_rest) + self.tau_syn * I) / self.tau_m
self.V += dV_dt * dt
if self.V >= self.V_th:
self.V = self.V_reset
self.t_ref = self.tau_ref
else:
self.t_ref -= dt
# STDP 突触可塑性类
class STDP:
def __init__(self, tau_pos, tau_neg, A_pos, A_neg):
self.tau_pos = tau_pos # 正相位突触可塑性时间常数
self.tau_neg = tau_neg # 负相位突触可塑性时间常数
self.A_pos = A_pos # 正相位突触可塑性增益
self.A_neg = A_neg # 负相位突触可塑性增益
self.last_spike_time = -np.inf # 上一个发放脉冲的时间
def update(self, pre_spike_time, post_spike_time):
delta_t = post_spike_time - pre_spike_time
if delta_t > 0:
dw = self.A_pos * np.exp(-delta_t / self.tau_pos)
else:
dw = -self.A_neg * np.exp(delta_t / self.tau_neg)
return dw
# 创建 LIF-STDP-SOM 类
class LIF_STDP_SOM:
def __init__(self, input_size, som_shape, tau_m, tau_ref, tau_syn, V_rest, V_reset, V_th,
tau_pos, tau_neg, A_pos, A_neg, sigma, learning_rate):
self.input_size = input_size # 输入层大小
self.som_shape = som_shape # SOM 网格形状
self.lif_neurons = [] # LIF 神经元列表
self.weights = np.random.randn(som_shape[0], som_shape[1], input_size) # 初始化 SOM 权重
self.stdp = STDP(tau_pos, tau_neg, A_pos, A_neg) # STDP 突触可塑性
# 初始化 LIF 神经元
for _ in range(input_size):
neuron = LIFNeuron(tau_m, tau_ref, tau_syn, V_rest, V_reset, V_th)
self.lif_neurons.append(neuron)
self.som = MiniSom(som_shape[0], som_shape[1], input_size, sigma=sigma, learning_rate=learning_rate)
def update(self, input_signal, dt):
spike_times = []
for i in range(self.input_size):
neuron = self.lif_neurons[i]
neuron.update(input_signal[i], dt)
if neuron.V >= neuron.V_th:
spike_times.append(i)
neuron.V = neuron.V_reset
neuron.t_ref = neuron.tau_ref
return spike_times
def learn(self, spike_times):
if len(spike_times) > 0:
self.som.update_weights(self.weights, spike_times)
def train(self, input_signals, num_iterations):
for _ in range(num_iterations):
for i in range(len(input_signals)):
spike_times = self.update(input_signals[i], 1)
self.learn(spike_times)
def predict(self, input_signal):
spike_times = self.update(input_signal, 1)
return self.som.winner(spike_times)
# 示例使用
input_size = 10 # 输入层大小
som_shape = (10, 10) # SOM 网格形状
tau_m = 10 # 膜时间常数
tau_ref = 1 # 绝对不应期时间常数
tau_syn = 5 # 突触后电流时间常数
V_rest = 0 # 静息膜电位
V_reset = 0 # 复位电位
V_th = 1 # 阈值电位
tau_pos = 10 # 正相位突触可塑性时间常数
tau_neg = 10 # 负相位突触可塑性时间常数
A_pos = 0.1 # 正相位突触可塑性增益
A_neg = 0.1 # 负相位突触可塑性增益
sigma = 1.0 # 高斯核函数的标准差
learning_rate = 0.5 # SOM 学习率
# 创建 LIF-STDP-SOM 对象
lif_stdp_som = LIF_STDP_SOM(input_size, som_shape, tau_m, tau_ref, tau_syn, V_rest, V_reset, V_th,
tau_pos, tau_neg, A_pos, A_neg, sigma, learning_rate)
# 训练数据
input_signals = np.random.randn(1000, input_size)
lif_stdp_som.train(input_signals, num_iterations=10)
# 预测数据
test_input = np.random.randn(input_size)
winner = lif_stdp_som.predict(test_input)
# 绘制脉冲分布图
plt.imshow(lif_stdp_som.weights[:, :, winner].reshape(som_shape[0], som_shape[1]), cmap='hot')
plt.colorbar()
plt.title('Pulse Distribution')
plt.show()
```
请确保在运行代码之前已安装必要的库(numpy、matplotlib 和 minisom)。这段代码会创建一个 LIF-STDP-SOM 网络,使用随机离散函数进行训练,并输出脉冲分布图。您可以根据需要调整参数和网络结构。希望对您有所帮助!
相关推荐
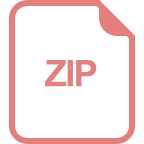
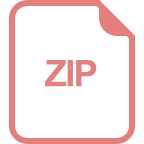
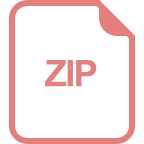















