LIF-STDP-SOM的python代码
时间: 2024-01-08 20:03:51 浏览: 37
LIF-STDP-SOM(Leaky Integrate and Fire - Spike-Timing Dependent Plasticity - Self-Organizing Map)是一种结合了LIF神经元模型、时序相关可塑性(Spike-Timing Dependent Plasticity, STDP)和自组织映射(Self-Organizing Map, SOM)的神经网络模型。以下是一个简单的LIF-STDP-SOM的Python代码示例:
```python
import numpy as np
class LIFSTDP:
def __init__(self, input_dim, map_dim, learning_rate, tau_pre, tau_post):
self.input_dim = input_dim
self.map_dim = map_dim
self.learning_rate = learning_rate
self.tau_pre = tau_pre
self.tau_post = tau_post
self.weights = np.random.rand(map_dim[0], map_dim[1], input_dim)
self.pre_trace = np.zeros((map_dim[0], map_dim[1]))
self.post_trace = np.zeros((map_dim[0], map_dim[1]))
def lif(self, inputs):
self.weights -= self.learning_rate * (self.weights - inputs)
def stdp(self, pre_spike, post_spike):
self.pre_trace = self.pre_trace * np.exp(-pre_spike / self.tau_pre) + pre_spike
self.post_trace = self.post_trace * np.exp(-post_spike / self.tau_post) + post_spike
delta_weights = np.outer(self.pre_trace, self.post_trace)
self.weights += self.learning_rate * delta_weights
def som(self, input_data, num_iterations):
for _ in range(num_iterations):
for input_vector in input_data:
distances = np.sum((self.weights - input_vector) ** 2, axis=2)
winner = np.unravel_index(np.argmin(distances), distances.shape)
self.lif(input_vector)
self.stdp(pre_spike=np.eye(self.map_dim[0], self.map_dim[1])[winner],
post_spike=np.eye(self.map_dim[0], self.map_dim[1]))
def get_map(self):
return self.weights
# 示例使用
input_data = np.array([[0.2, 0.4, 0.6], [0.1, 0.3, 0.5], [0.7, 0.8, 0.9]])
lif_stdp = LIFSTDP(input_dim=3, map_dim=(5, 5), learning_rate=0.1, tau_pre=20, tau_post=20)
lif_stdp.som(input_data, num_iterations=100)
map_weights = lif_stdp.get_map()
print(map_weights)
```
在这个示例代码中,`LIFSTDP` 类表示LIF-STDP-SOM模型。在初始化时,我们需要指定输入维度(`input_dim`)、映射维度(`map_dim`)、学习率(`learning_rate`)、前向时序相关可塑性时间常数(`tau_pre`)和后向时序相关可塑性时间常数(`tau_post`)。模型的权重是一个三维数组,其中前两个维度表示映射的坐标,第三个维度表示输入的特征维度。此外,我们还定义了用于跟踪前向和后向脉冲的追踪变量。
`lif()` 方法表示LIF神经元模型,它根据输入值更新权重。`stdp()` 方法表示STDP算法,它根据前向和后向脉冲更新权重。`som()` 方法用于执行SOM算法,遍历输入数据并进行LIF和STDP操作。`get_map()` 方法用于获取更新后的权重映射。
示例中的 `input_data` 是一个简单的3维输入数据的示例,然后创建一个 `LIFSTDP` 对象,并调用 `som()` 方法进行训练。最后,通过调用 `get_map()` 方法获取训练后的权重映射。
请注意,这只是一个简单的示例代码,实际应用中可能需要根据具体需求进行修改和扩展。
相关推荐
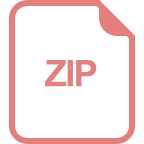
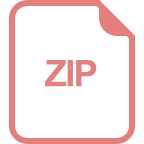














