class LogisticRegression(): def __init__(self, num_feature: int, learning_rate: float) -> None: ''' Constructor Parameters: num_features is the number of features. learning_rate is the learning rate. Return: there is no return value. ''' self.num_feature = num_feature self.w = np.random.randn(num_feature + 1) self.learning_rate = learning_rate这段是什么意思
时间: 2024-02-14 08:14:25 浏览: 76
这是一个 Logistic 回归模型的 Python 类的初始化方法。在初始化方法中:
- `num_feature` 表示特征的数量;
- `learning_rate` 表示学习率。
在初始化方法中,首先将特征的数量 `num_feature` 和学习率 `learning_rate` 存储到类的属性中。然后,使用 `np.random.randn` 方法初始化权重向量 `self.w`,其中 `num_feature + 1` 表示权重向量的长度,因为还需要添加一个偏置项。最后,将学习率也存储到类的属性中。
相关问题
代码为:class LogisticRegression(nn.Module): def __inti__(self,n_input_features): super(LogisticRegression,self).__inti() self.linea=nn.Linear(n_input_features,1) def forward(self,x): y_predicted=torch.sigmoid(self.linea(x)) return y_predicted model=LogisticRegression(n_feature)
这段代码定义了一个 LogisticRegression 类,继承了 nn.Module。构造函数 __init__() 中传入了输入特征的数量 n_input_features,然后调用了父类的构造函数,并定义了一个线性层 self.linea,其输入特征数量为 n_input_features,输出特征数量为 1。
forward() 方法定义了模型的前向传播过程。输入 x 经过线性层 self.linea 后,再经过 sigmoid 函数得到 y_predicted,即预测值。
最后,利用该类构造一个模型对象 model,传入特征数量 n_feature。
class LogisticRegression(object): def __init__(self, input_size, output_size, eta, max_epoch, eps):
def __init__(self, input_size, output_size, eta=0.01, max_epoch=1000, eps=1e-6):
"""
Initialize logistic regression model with hyperparameters
input_size: int - size of input features
output_size: int - number of output classes
eta: float - learning rate
max_epoch: int - maximum number of training epochs
eps: float - convergence threshold
"""
self.input_size = input_size
self.output_size = output_size
self.eta = eta
self.max_epoch = max_epoch
self.eps = eps
self.weights = np.zeros((input_size, output_size))
self.bias = np.zeros(output_size)
阅读全文
相关推荐
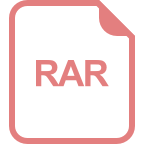
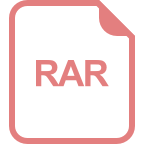
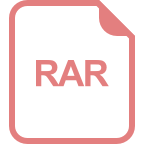
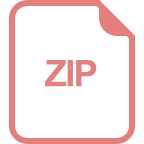
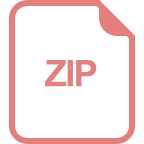
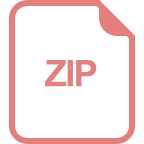
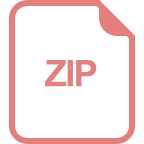
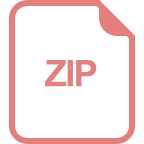


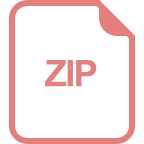
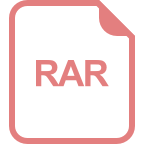
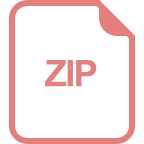

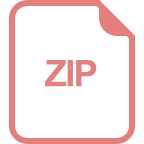