if ignore is not None:
时间: 2024-05-19 22:12:21 浏览: 12
This code snippet is checking whether the variable "ignore" is not equal to None. If it is not None, the code inside the if statement will be executed.
相关问题
解释下面这段代码,并说明哪些参数是可调整的:def adjust_predicts(score, label, threshold, pred=None, calc_latency=False): """ Calculate adjusted predict labels using given `score`, `threshold` (or given `pred`) and `label`. Args: score (np.ndarray): The anomaly score label (np.ndarray): The ground-truth label threshold (float): The threshold of anomaly score. A point is labeled as "anomaly" if its score is lower than the threshold. pred (np.ndarray or None): if not None, adjust `pred` and ignore `score` and `threshold`, calc_latency (bool): Returns: np.ndarray: predict labels Method from OmniAnomaly (https://github.com/NetManAIOps/OmniAnomaly) """ if label is None: predict = score > threshold return predict, None if pred is None: if len(score) != len(label): raise ValueError("score and label must have the same length") predict = score > threshold else: predict = pred actual = label > 0.1 anomaly_state = False anomaly_count = 0 latency = 0 for i in range(len(predict)): if any(actual[max(i, 0) : i + 1]) and predict[i] and not anomaly_state: anomaly_state = True anomaly_count += 1 for j in range(i, 0, -1): if not actual[j]: break else: if not predict[j]: predict[j] = True latency += 1 elif not actual[i]: anomaly_state = False if anomaly_state: predict[i] = True if calc_latency: return predict, latency / (anomaly_count + 1e-4) else: return
该函数用于调整预测标签,基于给定的分数 `score`、阈值 `threshold`(或给定的预测值 `pred`)和真实标签 `label`。 如果一个点的分数低于阈值,则将其标记为“异常”点。
参数 `score`、`label` 和 `threshold` 都是可调整的。
参数说明:
- `score`:np.ndarray 类型,表示每个时间步的异常分数;
- `label`:np.ndarray 类型,表示每个时间步的真实标签;
- `threshold`:float 类型,表示异常分数的阈值。如果一个点的分数低于阈值,则将其标记为“异常”点;
- `pred`:np.ndarray 类型或者 None,表示是否给定预测值,如果给定,会忽略 `score` 和 `threshold`;
- `calc_latency`:bool 类型,表示是否计算延迟。
该函数首先判断是否有真实标签 `label`,如果没有,则直接通过阈值 `threshold` 对异常分数 `score` 进行预测,并返回预测标签和延迟。如果有真实标签,则通过阈值 `threshold` 或预测值 `pred` 对异常分数 `score` 进行预测,并根据实际情况对预测标签进行调整,最终返回调整后的标签和延迟。
其中 `actual` 表示真实标签中大于 0.1 的标签,`anomaly_state` 表示当前是否处于异常状态,`anomaly_count` 表示异常的数量,`latency` 表示延迟的数量。
该函数中可调整的参数是 `score`、`label` 和 `threshold`。
def Focal_Loss(inputs, target, cls_weights, num_classes=21, alpha=0.5, gamma=2): n, c, h, w = inputs.size() nt, ht, wt = target.size() if h != ht and w != wt: inputs = F.interpolate(inputs, size=(ht, wt), mode="bilinear", align_corners=True) temp_inputs = inputs.transpose(1, 2).transpose(2, 3).contiguous().view(-1, c) temp_target = target.view(-1) logpt = -nn.CrossEntropyLoss(weight=cls_weights, ignore_index=num_classes, reduction='none')(temp_inputs, temp_target) pt = torch.exp(logpt) if alpha is not None: logpt *= alpha loss = -((1 - pt) ** gamma) * logpt loss = loss.mean() return loss 是什么意思
这段代码实现了 Focal Loss,是一种用于解决分类问题中,样本不平衡导致的分类精度下降的方法。其中,输入的参数包括:
- inputs:模型的输出,是一个大小为 (n, c, h, w) 的张量,其中 n 表示 batch size,c 表示类别数,h 和 w 表示输入图片的高和宽。
- target:真实标签,是一个大小为 (n, h, w) 的张量。
- cls_weights:各个类别的权重,是一个大小为 (c,) 的张量。
- num_classes:类别数,默认为 21。
- alpha:平衡因子,控制正负样本的权重比例,默认为 0.5。
- gamma:调节因子,控制易错样本的权重,默认为 2。
该函数的返回值是一个标量,表示 Focal Loss 的值。
相关推荐
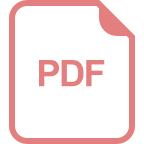
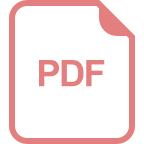
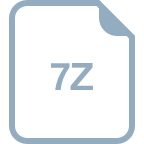













