t_obs = tensor([[ 3.9165e+00, -3.2818e-01, 3.9585e+00, -3.2877e-01, 2.5000e+00, 4.0000e+00, 2.9092e-04, -3.1356e-04, -5.0448e-02, -1.0185e-02, -2.6571e-01, 3.5682e-02, 3.2696e-01, 1.4884e-01, -2.9417e-01, 7.9636e+00, -2.0701e+00, -1.0490e+01, -5.4630e-01, 1.3817e+01, 9.7782e+00, 1.0266e-01, 7.3403e-02, 1.0498e-01]], device='cuda:0') 。actor: torch.nn.Module, t_obs = torch.FloatTensor((train_collector.data.obs)[0]).unsqueeze(0).to(args.device) t_act = torch.FloatTensor((train_collector.data.act)[0]).unsqueeze(0).to(args.device) print(t_obs) print(t_act) writer.add_graph(policy.actor, t_obs)出现RuntimeError: Only tensors, lists, tuples of tensors, or dictionary of tensors can be output from traced functions错误,怎么改正代码
时间: 2024-02-24 17:59:51 浏览: 16
这个错误通常是由于`add_graph`方法不能接受`torch.nn.Module`类型的参数,而应该是一个计算图(`torch.onnx.export`)或一组张量。要修复这个错误,可以使用`torch.jit.trace`函数来创建一个跟踪模块,然后将跟踪模块的输入张量作为参数传递给`add_graph`方法。
以下是可能的解决方案,可以将其添加到代码中尝试:
```
# 使用torch.jit.trace创建跟踪模块
traced_actor = torch.jit.trace(policy.actor, (t_obs,))
# 将输入张量传递给add_graph方法
writer.add_graph(traced_actor, (t_obs,))
```
这应该可以解决该错误,允许您将模型添加到TensorBoard中。
相关问题
t_obs = torch.FloatTensor((train_collector.data.obs)[0]).unsqueeze(0).to(args.device)作为输入 tensor([[ 3.8838e+00, 3.5745e+00, 3.8844e+00, 3.6165e+00, 2.5000e+00, 4.0000e+00, -2.8299e-03, 3.1723e-03, 1.4587e+00, -2.5189e-01, -2.4204e
抱歉,我之前的回答似乎部分内容丢失了,这里提供完整的回答:
`torch.FloatTensor((train_collector.data.obs)[0]).unsqueeze(0).to(args.device)` 的作用是将训练数据集中的第一个样本的 `obs` 数据转换为 `FloatTensor` 类型,并在第0维度上加了一个维度,最后将其移动到指定设备上(这里是 `cuda:0`)。这个张量可能是一个状态向量,用于输入到模型中进行推理或训练。
如果要将这个张量的计算图加到TensorBoard中,可以使用以下代码:
```python
from torch.utils.tensorboard import SummaryWriter
writer = SummaryWriter()
dummy_input = torch.randn(1, 24).to('cuda:0') # 这里输入张量的形状需要和模型输入的形状一致
writer.add_graph(model, (dummy_input,))
```
其中,`model` 是你的模型,`dummy_input` 是一个随机输入张量,用于推导模型的计算图。最后,运行TensorBoard时,可以在Graphs选项卡中查看模型的计算图。
转matlab: n_points_total = numpy.int(noisy_sensor_measured_total.shape[1]/(n_obs_in_sensor_array + 1)) intrinsic_process_total_reshaped = numpy.reshape(intrinsic_process_total, [dim_intrinsic, n_points_total, n_obs_in_sensor_array + 1], order='C') noisy_sensor_measured_total_reshaped = numpy.reshape(noisy_sensor_measured_total, [dim_measurement, n_points_total, n_obs_in_sensor_array + 1], order='C') intrinsic_process_base_total = intrinsic_process_total_reshaped[:, :, 0] intrinsic_process_step_total = intrinsic_process_total_reshaped[:, :, 1:] noisy_sensor_base_total = noisy_sensor_measured_total_reshaped[:, :, 0] n_obs_used_in_each_cluster = min(n_obs_used_in_cluster, n_obs_in_sensor_array) obs_used_in_each_cluster_indexes = numpy.random.choice(n_obs_in_sensor_array, size=n_obs_used_in_each_cluster, replace=False) sensor_array_matrix = sensor_array_matrix_dense[:, obs_used_in_each_cluster_indexes] noisy_sensor_step_total = noisy_sensor_measured_total_reshaped[:, :, 1:]
在Matlab中,您可以将以下Python代码转换为对应的Matlab代码:
```matlab
n_points_total = int(size(noisy_sensor_measured_total, 2) / (n_obs_in_sensor_array + 1));
intrinsic_process_total_reshaped = reshape(intrinsic_process_total, [dim_intrinsic, n_points_total, n_obs_in_sensor_array + 1]);
noisy_sensor_measured_total_reshaped = reshape(noisy_sensor_measured_total, [dim_measurement, n_points_total, n_obs_in_sensor_array + 1]);
intrinsic_process_base_total = intrinsic_process_total_reshaped(:, :, 1);
intrinsic_process_step_total = intrinsic_process_total_reshaped(:, :, 2:end);
noisy_sensor_base_total = noisy_sensor_measured_total_reshaped(:, :, 1);
n_obs_used_in_each_cluster = min(n_obs_used_in_cluster, n_obs_in_sensor_array);
obs_used_in_each_cluster_indexes = datasample(1:n_obs_in_sensor_array, n_obs_used_in_each_cluster, 'Replace', false);
sensor_array_matrix = sensor_array_matrix_dense(:, obs_used_in_each_cluster_indexes);
noisy_sensor_step_total = noisy_sensor_measured_total_reshaped(:, :, 2:end);
```
请注意,上述代码中的 `datasample` 函数用于在 `1:n_obs_in_sensor_array` 中随机选择 `n_obs_used_in_each_cluster` 个索引,并且不允许重复。如果您的Matlab版本不支持 `datasample` 函数,可以使用以下代码替代:
```matlab
obs_used_in_each_cluster_indexes = randsample(1:n_obs_in_sensor_array, n_obs_used_in_each_cluster, false);
```
这里使用了 `randsample` 函数来实现相同的功能。
相关推荐
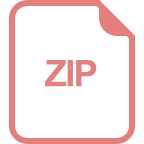












