import numpy as np import matplotlib.pyplot as plt from keras.models import Sequential from keras.layers import Dense from tensorflow.keras.utils import plot_model # 随机生成1000个二维坐标数据 X = np.random.rand(1000, 2) y = np.zeros((1000, 1)) # 根据y=x对数据进行分类 for i in range(1000): if X[i, 1] > X[i, 0]: y[i, 0] = 1 else: y[i, 0] = 0 # 定义神经网络模型,没有隐藏层 model = Sequential() model.add(Dense(1, input_dim=2, activation='sigmoid')) # 编译模型 model.compile(loss='binary_crossentropy', optimizer='adam', metrics=['accuracy']) # 训练模型 model.fit(X, y, epochs=50, batch_size=10) # 输出模型的权重数据 weights = model.get_weights() for i, weight in enumerate(weights): print(f'Layer {i} weights:\n{weight}\n') # 绘制随机生成的坐标数据图 plt.scatter(X[:, 0], X[:, 1], c=y[:, 0]) plt.xlabel('X') plt.ylabel('Y') plt.show()修改模型,使训练出的权重自身为整数
时间: 2024-04-12 14:31:56 浏览: 23
要使训练出的权重自身为整数,你可以在训练结束后对权重进行四舍五入,然后重新设置模型的权重。以下是修改后的代码示例:
```python
import numpy as np
import matplotlib.pyplot as plt
from keras.models import Sequential
from keras.layers import Dense
from tensorflow.keras.utils import plot_model
# 随机生成1000个二维坐标数据
X = np.random.rand(1000, 2)
y = np.zeros((1000, 1))
# 根据y=x对数据进行分类
for i in range(1000):
if X[i, 1] > X[i, 0]:
y[i, 0] = 1
else:
y[i, 0] = 0
# 定义神经网络模型,没有隐藏层
model = Sequential()
model.add(Dense(1, input_dim=2, activation='sigmoid'))
# 编译模型
model.compile(loss='binary_crossentropy', optimizer='adam', metrics=['accuracy'])
# 训练模型
model.fit(X, y, epochs=50, batch_size=10)
# 输出模型的权重数据
weights = model.get_weights()
for i, weight in enumerate(weights):
print(f'Layer {i} weights:\n{weight}\n')
# 四舍五入并设置整数权重
rounded_weights = [np.round(w) for w in weights]
model.set_weights(rounded_weights)
# 输出四舍五入后的整数权重数据
rounded_weights = model.get_weights()
for i, weight in enumerate(rounded_weights):
print(f'Layer {i} rounded weights:\n{weight}\n')
# 绘制随机生成的坐标数据图
plt.scatter(X[:, 0], X[:, 1], c=y[:, 0])
plt.xlabel('X')
plt.ylabel('Y')
plt.show()
```
在这个修改后的代码中,我使用`np.round()`函数对权重进行四舍五入,并将四舍五入后的整数权重重新设置到模型中。然后,我打印出四舍五入后的整数权重数据。请注意,四舍五入可能会对模型的性能产生影响,所以请根据实际需求和问题来决定是否进行四舍五入。
相关推荐
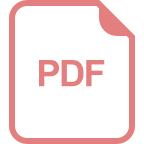
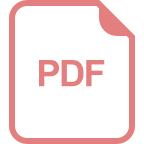








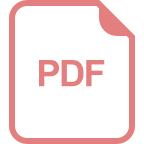
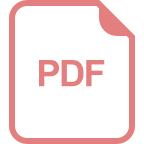
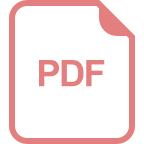
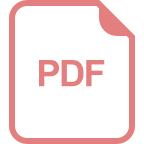
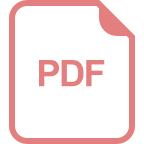
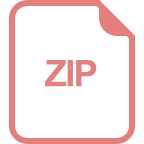
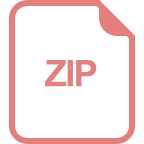