unet加入自注意力机制和交叉注意力机制
时间: 2024-12-26 13:17:45 浏览: 6
### 如何在UNet架构中引入Self-Attention和Cross-Attention
#### 添加 Self-Attention 到 UNet Encoder 部分
为了增强编码器部分捕捉全局上下文的能力,可以在每一层的卷积操作之后加入self-attention模块。具体来说:
1. **定义Self-Attention Layer**
使用线性变换来计算查询(Q)、键(K)以及值(V),接着应用缩放点乘法得到注意力权重矩阵,并将其应用于原始输入特征图。
```python
def self_attention_layer(x, d_model):
q = Conv2D(d_model, (1, 1), padding='same')(x)
k = Conv2D(d_model, (1, 1), padding='same')(x)
v = Conv2D(d_model, (1, 1), padding='same')(x)
attn_scores = tf.matmul(q, k, transpose_b=True) / np.sqrt(d_model)
attn_weights = Softmax()(attn_scores)
output = tf.matmul(attn_weights, v)
return Add()([output, x])
```
2. **集成到Encoder Blocks**
将上述`self_attention_layer()`函数嵌入至每一个下采样阶段后的残差连接之前[^1]。
#### 在Skip Connection处添加 Cross-Attention
为了让解码路径更好地利用来自不同尺度的信息流,在跳跃链接处实施cross-attention有助于过滤掉不必要的细节并保留重要的结构化特性。这可以通过以下方式完成:
1. **构建Cross-Attention Module**
设计一个接受两个输入张量——即低分辨率特征映射F_LowRes与高分辨率特征映射F_HighRes——作为参数的跨模态关注力组件。该模块负责生成加权组合形式的新表示G_CrossAtten。
```python
class CrossAttentionLayer(Layer):
def __init__(self, channels):
super(CrossAttentionLayer, self).__init__()
self.query_conv = Conv2D(channels//8, kernel_size=1)
self.key_conv = Conv2D(channels//8, kernel_size=1)
self.value_conv = Conv2D(channels, kernel_size=1)
self.gamma = Dense(1)
def call(self, low_res_feat, high_res_feat):
batch_size, height_low, width_low, chans_low = K.int_shape(low_res_feat)
_, height_high, width_high, _ = K.int_shape(high_res_feat)
proj_query = Reshape((height_low * width_low, chans_low))(low_res_feat)
proj_key = Permute((3, 1, 2))(high_res_feat)
energy = MatMul()(proj_query, proj_key)
attention = Activation('softmax')(energy)
proj_value = Reshape((chans_low, height_high * width_high))(Permute((3, 1, 2))(high_res_feat))
out = Dot(axes=[2])([attention, proj_value])
gamma = Lambda(lambda t: t * self.gamma)(out)
result = Add()([gamma, low_res_feat])
return result
```
2. **部署于Decoder Side 的 Skip Connections**
当从前一层传递过来的数据准备同对应的高层次特征相结合时,先经过此定制化的cross-attention处理单元再继续后续的操作流程[^3]。
---
阅读全文
相关推荐
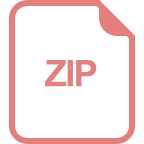
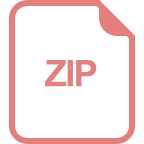
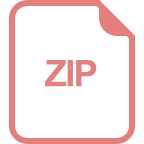
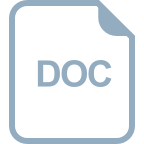
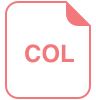
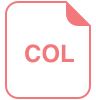
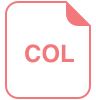
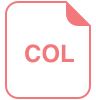

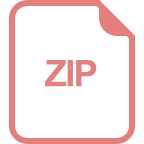
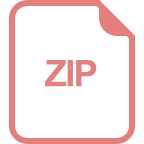
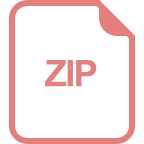
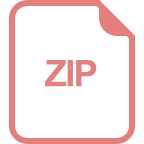
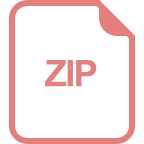