用C++部署yolov5模型
时间: 2023-08-10 18:07:17 浏览: 141
要在C++中部署yolov5模型,可以按照以下步骤进行:
1. 安装OpenCV库:需要安装OpenCV库来处理图像数据。
2. 下载yolov5模型文件:从yolov5官方网站上下载相应的模型文件。
3. 安装libtorch:yolov5使用了Pytorch作为框架,要在C++中使用yolov5,需要安装libtorch。
4. 加载模型:使用libtorch的API加载yolov5模型,例如:
```c++
torch::jit::script::Module module = torch::jit::load("path/to/model.pt");
```
5. 处理输入数据:将图像数据转换为模型输入所需的格式,例如使用OpenCV库读取图像并转换为Tensor类型。
6. 运行模型:将处理后的输入数据输入到模型中运行,例如:
```c++
at::Tensor output = module.forward({input}).toTensor();
```
7. 处理输出数据:将模型输出的Tensor类型结果转换为易于理解的格式,例如使用OpenCV库将结果绘制在图像上。
以上是部署yolov5模型的大致步骤,具体实现细节需要根据实际情况进行调整。
相关问题
C++ 部署yolov5
您好!对于C++部署Yolov5模型,您可以按照以下步骤进行:
1. 首先,您需要将Yolov5模型从PyTorch转换为ONNX格式。您可以使用以下命令将PyTorch模型转换为ONNX格式:
```python
import torch
from models.experimental import attempt_load
# Load Yolov5 model
model = attempt_load('yolov5.pt', map_location=torch.device('cpu'))
# Export the model to ONNX format
torch.onnx.export(model, (torch.zeros(1, 3, 640, 640),), 'yolov5.onnx', opset_version=11)
```
2. 接下来,您需要使用C++编写代码来加载和运行ONNX模型。您可以使用OpenCV库来处理图像。以下是一个简单的示例代码,用于加载并运行Yolov5模型:
```cpp
#include <iostream>
#include <opencv2/opencv.hpp>
#include <onnxruntime/core/providers/cpu/cpu_provider_factory.h>
#include <onnxruntime/core/providers/cuda/cuda_provider_factory.h>
#include <onnxruntime/core/providers/cpu/tensor/concat_cpu.h>
#include <onnxruntime/core/providers/cpu/tensor/split_cpu.h>
#include <onnxruntime/core/providers/cpu/tensor/transpose_cpu.h>
#include <onnxruntime/core/providers/cpu/tensor/unsqueeze_cpu.h>
#include <onnxruntime/core/providers/cpu/tensor/slice_cpu.h>
#include <onnxruntime/core/providers/cpu/tensor/resize_cpu.h>
int main() {
// Load the ONNX model
Ort::Env env(ORT_LOGGING_LEVEL_WARNING, "Yolov5");
Ort::SessionOptions session_options;
session_options.SetIntraOpNumThreads(1);
session_options.SetGraphOptimizationLevel(ORT_ENABLE_EXTENDED);
Ort::Session session(env, "yolov5.onnx", session_options);
// Prepare input image
cv::Mat image = cv::imread("image.jpg");
cv::cvtColor(image, image, cv::COLOR_BGR2RGB);
cv::resize(image, image, cv::Size(640, 640));
std::vector<cv::Mat> inputImages{image};
// Preprocess input image
std::vector<float> inputData(3 * 640 * 640);
for (int c = 0; c < 3; ++c) {
for (int i = 0; i < 640 * 640; ++i) {
inputData[c * 640 * 640 + i] = (float)inputImages[0].data[i * 3 + c] / 255.0;
}
}
// Create ONNX runtime input tensor
std::vector<int64_t> inputShape{1, 3, 640, 640};
Ort::MemoryInfo memory_info = Ort::MemoryInfo::CreateCpu(OrtAllocatorType::OrtArenaAllocator, OrtMemType::OrtMemTypeDefault);
// Bind input tensor to ONNX runtime session
size_t inputTensorSize = sizeof(float) * inputData.size();
Ort::Value inputTensor = Ort::Value::CreateTensor<float>(memory_info, inputData.data(), inputTensorSize, inputShape.data(), inputShape.size());
const char* inputNames[] = { "input" };
const char* outputNames[] = { "output" };
Ort::RunOptions run_options;
Ort::AllocatorWithDefaultOptions allocator;
// Run inference
std::vector<Ort::Value> outputTensors = session.Run(run_options, inputNames, &inputTensor, 1, outputNames, 1);
// Postprocess output tensors
// ...
return 0;
}
```
请注意,上述示例代码仅用于演示目的,并且可能需要根据您的实际情况进行调整。您可能还需要根据模型的输出进行后处理,以获取检测到的对象的边界框和类别等信息。
3. 在编译代码之前,请确保已正确安装OpenCV和ONNX Runtime库,并将相关头文件和库文件正确链接到您的项目中。
希望以上信息能对您有所帮助!如果您有任何疑问,请随时提问。
c++部署yolov5
TensorRT是一个高性能的深度学习推理库,可以帮助您在 NVIDIA GPU 上加速深度学习推理。要在C++中部署YOLOv5,您需要进行以下步骤:
1.安装 TensorRT:在系统上安装 TensorRT 库和配套的驱动程序。
2.导出权重:在训练模型之后,您需要将权重导出到一个可以被 TensorRT 读取的格式,如 ONNX 或 TensorRT 格式。
3.构建网络:使用 TensorRT 的 C++ API 构建网络,并将权重加载到网络中。
4.配置推理:设置网络的运行参数,如批量大小和精度。
5.运行推理:使用 TensorRT 运行网络,并得到结果。
6.解码结果:最后,您需要对结果进行解码,以便更好地理解结果。
希望这能帮到你。
阅读全文
相关推荐
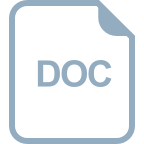
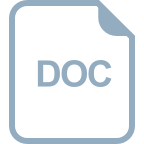
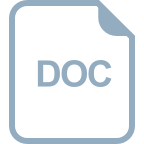
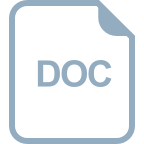
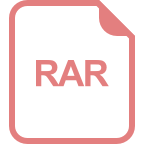
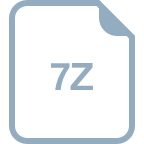


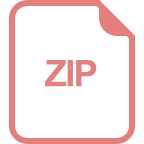
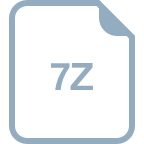
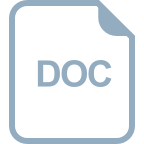
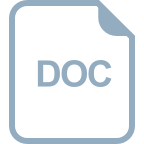
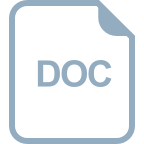

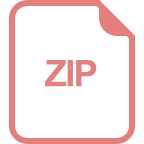