f,ax=plt.subplots(1,2,figsize=(12,5)) sns.set_palette(["#9b59b6","#3498db",]) #设置所有图的颜色,使用hls色彩空间 sns.distplot(ruesult_df['fav'],bins=30,kde=True,label='123',ax=ax[0]) sns.distplot(ruesult_df['cart'],bins=30,kde=True,label='12',ax=ax[1])代码解释
时间: 2023-06-07 15:08:56 浏览: 45
f,ax=plt.subplots(1,2,figsize=(12,5)) sns.set_palette(["..."]) 这行代码的作用是创建一个包含两个子图的画布,大小为12*5,同时设置 seaborn(一个常用的可视化库)的调色板为指定颜色。
相关问题
import numpy as np import scipy.stats as ss import pandas as pd import matplotlib.pyplot as plt import seaborn as sns df=pd.read_excel("./tmp_apply.xlsx") sns.set_context(font_scale=20) plt.rcParams['font.family'] = 'FangSong' plv_tb=pd.pivot_table(df,values="sale",index=["FactoryName","JiJXH"],columns=["Xian"],aggfunc=np.mean) fig, ax = plt.subplots(figsize=(200,10)) sns.heatmap(plv_tb,vmin=plv_tb.min().min(), vmax=plv_tb.max().max(),cmap=sns.color_palette("RdYlBu",n_colors=10),annot=True, fmt='g', annot_kws={"size": 20}, ax=ax) plt.subplots_adjust(left=0, bottom=0) # 调整左边和底部的距离 plt.show()热力图和色阶图之间距离还是没有变小
你可以尝试调整 `figsize` 参数来改变热力图的大小,也可以使用 `plt.tight_layout()` 函数来自动调整子图间距离。例如:
```
fig, ax = plt.subplots(figsize=(15,10))
sns.heatmap(plv_tb,vmin=plv_tb.min().min(), vmax=plv_tb.max().max(),cmap=sns.color_palette("RdYlBu",n_colors=10),annot=True, fmt='g', annot_kws={"size": 10}, ax=ax)
plt.tight_layout()
plt.show()
```
你可以根据需要调整 `figsize` 和 `annot_kws` 参数来适配你的数据和字体大小。
fig,ax=plt.subplots(1,2,figsize=(14,5)) sns.countplot(x='cp',data=data,hue='target',palette='Set3',ax=ax[0]) ax[0].set_xlabel("胸痛类型") data.cp.value_counts().plot.pie(ax=ax[1],autopct='%1.1f%%',explode=[0.01,0.01,0.01,0.01],shadow=True, cmap='Blues') ax[1].set_title("胸痛类型")这段代码有错吗?怎么改
这段代码也有一点小错误,需要在两个地方做出修改。应该将两个代码行分开写,并将第一个代码行的输出作为第二个代码行的输入,如下所示:
```
import matplotlib.pyplot as plt
import seaborn as sns
# 修改第一行代码
fig, ax = plt.subplots(1, 2, figsize=(14, 5))
sns.countplot(x='cp', data=data, hue='target', palette='Set3', ax=ax[0])
ax[0].set_xlabel("胸痛类型")
# 修改第二行代码
value_counts = data.cp.value_counts()
value_counts.plot.pie(ax=ax[1], autopct='%1.1f%%', explode=[0.01, 0.01, 0.01, 0.01], shadow=True, cmap='Blues')
ax[1].set_title("胸痛类型")
```
这样就可以正确地绘制胸痛类型的计数图和饼图了。
相关推荐
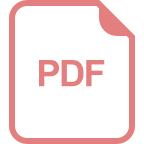
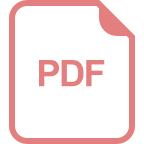
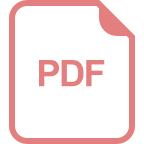












