错误使用 fitdist (line 126) X 必须为数值列向量。 出错 QX (line 5) pd = fitdist(log(data), 'Lognormal', 'Start', [mu, sigma]);% 绘制概率密度函数曲线
时间: 2024-03-25 07:38:46 浏览: 283
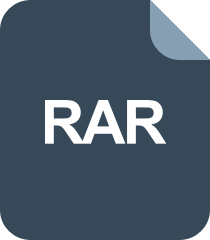
log_normal.rar_clutter matlab_gaussian clutter_lognormal_产生相关序列_
这个错误提示是由于`fitdist()`函数要求输入的数据必须是数值列向量,而`data`是一个矩阵。你需要将`data`转化为数值列向量。
下面是修改后的代码:
```matlab
% 准备数据
x = [1, 2, 3, 4, 5];
y = [0.1, 0.3, 0.4, 0.15, 0.05];
data = log([x', y']); % 将x和y组成一个矩阵,并将其转化为对数数据
% 拟合对数正态分布曲线
mu = mean(log(x)); % 对数正态分布的均值
sigma = std(log(x)); % 对数正态分布的标准差
pd = fitdist(data, 'Lognormal', 'Start', [mu, sigma]);
% 绘制概率密度函数曲线
x_vals = linspace(min(x), max(x), 100);
y_vals = pdf(pd, log(x_vals));
plot(x_vals, y_vals);
```
运行上述代码,就可以得到一条拟合好的对数正态分布曲线。
阅读全文
相关推荐
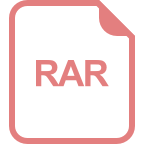
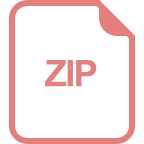
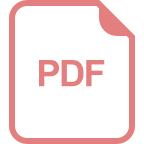
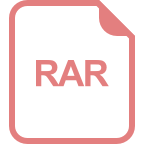











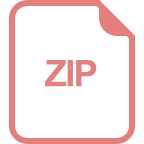