在R软件,怎样将group_df的数据横向合并到数据库data中
时间: 2024-05-02 19:20:25 浏览: 30
可以使用dplyr包中的left_join()函数将group_df的数据横向合并到数据库data中,具体操作如下:
1. 确认group_df和data中用于合并的变量名相同。
2. 使用left_join()函数将group_df的数据横向合并到data中,例如:
```{r}
library(dplyr)
data <- left_join(data, group_df, by = "变量名")
```
其中,"变量名"是group_df和data中用于合并的变量名。
3. 合并后的数据保存在data中。
相关问题
R语言中用group_by将同一个月同一天的数据汇总到一起怎么写代码
在R语言中,可以使用`dplyr`包中的`group_by`和`summarize`函数来实现按月、按日汇总数据。具体代码如下:
```R
library(dplyr)
# 假设数据框为df,其中有一个名为date的日期字段,一个名为value的数值字段
# 首先将日期字段转换为年月日格式
df$date <- as.Date(df$date)
# 按月份汇总数据
df_monthly <- df %>%
group_by(year_month = format(date, "%Y-%m")) %>%
summarize(total_value = sum(value))
# 按日期汇总数据
df_daily <- df %>%
group_by(date) %>%
summarize(total_value = sum(value))
```
其中,`format(date, "%Y-%m")`将日期字段转换为年月格式,`summarize(total_value = sum(value))`计算每个月或每天的数据总和。
将groupby中两列数据合并
可以使用`apply`方法和lambda函数来实现将groupby中的两列数据合并。假设有一个DataFrame `df`,其中有两列`col1`和`col2`,需要根据`col1`进行分组,然后将每组中的`col2`列合并成一个字符串。
代码示例:
```
df.groupby('col1')['col2'].apply(lambda x: ','.join(x)).reset_index()
```
其中,`groupby('col1')`表示根据`col1`列进行分组,`['col2']`表示对`col2`列进行操作,`lambda x: ','.join(x)`表示将每组中的`col2`列合并成一个以逗号分隔的字符串,`reset_index()`表示将结果重新设置索引。
相关推荐
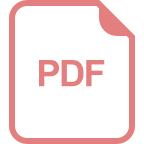
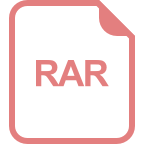
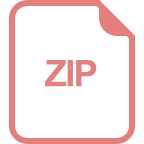












