rate.reset_index
时间: 2024-05-06 19:15:12 浏览: 11
`reset_index()` is a method in pandas library in Python that resets the index of a dataframe to the default integer index. It is useful when the index of a dataframe needs to be reset after some operations that may have changed the index.
For example, if you have a dataframe `df` with a custom index and you want to reset it to the default integer index, you can use the `reset_index()` method as follows:
```
df = df.reset_index()
```
This will reset the index of the dataframe `df` to the default integer index and create a new column called "index" with the old index values.
Now, coming to your code `rate.reset_index`, it seems that you are calling the `reset_index()` method on a dataframe called `rate`. However, since there is no assignment to a variable or any other operation being performed on the resulting dataframe, this code will not have any effect.
To actually reset the index of the dataframe `rate`, you need to assign the result of the `reset_index()` method to a variable or use the `inplace=True` parameter to modify the dataframe in place. For example:
```
rate = rate.reset_index()
```
Or,
```
rate.reset_index(inplace=True)
```
This will actually reset the index of the dataframe `rate` to the default integer index.
相关推荐
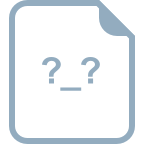
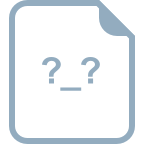
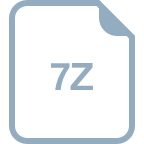














