val survived_sex_count=df2.groupBy("Sex","Survived").count() val survived_sex_percent=survived_sex_count.withColumn("percent",format_number(col("count").divide(sum("count").over()).multiply(100),5)); survived_sex_percent.show() survived_sex_percent.coalesce(1).write.option("header", "true").csv("/home/hadoop/titanic_output/survived_sex_percent.csv")
时间: 2024-03-31 22:35:10 浏览: 27
这是一个使用Spark SQL进行数据处理和分析的代码片段。它首先对一个名为df2的DataFrame按照“Sex”和“Survived”两列进行分组计数,然后使用Spark SQL中的窗口函数sum()计算出count列的总和。最后,使用withColumn()方法添加一个名为“percent”的列,计算每个组的占比,并使用format_number()方法将结果格式化为小数点后5位。最终结果会展示出来。接着,使用coalesce()方法将结果合并成一个分区,使用write()方法将结果写入指定路径下的CSV文件,文件名为“survived_sex_percent.csv”。同时,设置选项“header”为“true”,表示将列名写入CSV文件的第一行作为头部。这样可以方便地在其他工具中打开和使用这些数据。
相关问题
val survived_sex_count = df2.groupBy("Sex", "Survived").count() val survived_sex_percent = survived_sex_count.withColumn("percent", format_number(col("count") .divide(sum("count").over()) .multiply(100), 5)); survived_sex_percent.show() 中sum("count").over() 存在问题
对于`sum("count").over()`的问题,这是因为`over()`函数需要指定窗口范围,表示对哪些数据进行求和,而在这里未指定窗口范围,因此报错。
如果想要对每个不同的`Sex`进行比例计算,则需要在`over()`函数中指定分区窗口,以便对每个窗口内的数据进行求和。
可以尝试修改成以下代码:
```
import org.apache.spark.sql.expressions.Window
val windowSpec = Window.partitionBy("Sex")
val survived_sex_percent = survived_sex_count.withColumn("percent", format_number(col("count").divide(sum("count").over(windowSpec)).multiply(100), 5));
survived_sex_percent.show()
```
在这个修改后的代码中,`Window.partitionBy("Sex")`表示按照`Sex`字段进行分区,然后在`over()`函数中指定了窗口范围,表示对每个分区内的数据进行求和。这样就可以正确计算每个分区内存活者的性别分布所占比例,并输出结果。
val survived_sex_count=df2.groupBy("Sex","Survived").count() val survived_sex_percent=survived_sex_count.withColumn("percent", format_number(col("count").divide(sum("count").over()).multiply(100),5)); survived_sex_percent.show()
这是一个使用Spark SQL进行数据处理和分析的代码片段。它首先对一个名为df2的DataFrame按照“Sex”和“Survived”两列进行分组计数,然后使用Spark SQL中的窗口函数sum()计算出count列的总和。最后,使用withColumn()方法添加一个名为“percent”的列,计算每个组的占比,并使用format_number()方法将结果格式化为小数点后5位。最终结果会展示出来。
相关推荐
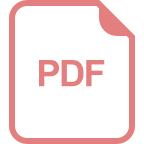










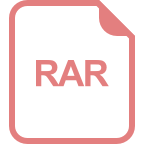