python pytorch 输入为一个[4,3,128,128]的张量和一个维度为[4,1,1,1]的张量,代码将两个张量拼接平整后输入三层全连接层神经网络,输出为一个维度为[4,1,1,1]的张量
时间: 2023-09-29 08:08:01 浏览: 76
以下是实现代码:
```python
import torch
# 定义输入张量
x1 = torch.randn(4, 3, 128, 128)
x2 = torch.randn(4, 1, 1, 1)
# 将两个张量拼接
x = torch.cat((x1.view(4, -1), x2.view(4, -1)), dim=1)
# 定义三层全连接层神经网络
model = torch.nn.Sequential(
torch.nn.Linear(x.shape[1], 64),
torch.nn.ReLU(),
torch.nn.Linear(64, 32),
torch.nn.ReLU(),
torch.nn.Linear(32, 1)
)
# 前向传播
output = model(x)
output = output.view(4, 1, 1, 1)
print(output.shape) # 输出:torch.Size([4, 1, 1, 1])
```
首先将两个张量拼接起来,需要将x1和x2张量的每个元素展开成一维向量,并在第二维上进行拼接,得到一个形状为[4, 3*128*128+1]的张量x。然后定义三层全连接层神经网络,输入为x的形状[4, 3*128*128+1],输出为形状[4, 1]的张量。最后将输出的张量reshape成[4, 1, 1, 1],即得到了形状为[4,1,1,1]的输出张量。
相关问题
pytorch 输入为一个[4,3,128,128]的张量和一个维度为[4,1,1,1]的张量,代码将两个张量拼接平整后输入三层全连接层神经网络,输出为一个维度为[4,1,1,1]的张量。写一个模型的类
可以按照如下方式实现:
```python
import torch
import torch.nn as nn
class MyModel(nn.Module):
def __init__(self):
super(MyModel, self).__init__()
self.fc1 = nn.Linear(3*128*128+1, 256)
self.fc2 = nn.Linear(256, 64)
self.fc3 = nn.Linear(64, 1)
def forward(self, x1, x2):
x2 = x2.view(-1, 1)
x = torch.cat([x1.view(x1.size(0), -1), x2], dim=1)
x = nn.functional.relu(self.fc1(x))
x = nn.functional.relu(self.fc2(x))
x = self.fc3(x)
return x.view(-1, 1, 1, 1)
```
其中,模型的输入有两个张量x1和x2,分别是[4,3,128,128]和[4,1,1,1]。在forward方法中,首先将x2的维度变为[4,1],然后将x1和x2沿着第二个维度(即通道维度)拼接,得到维度为[4,3\*128\*128+1]的张量,然后输入三层全连接层神经网络,最终输出维度为[4,1,1,1]的张量。注意在最后需要将输出的维度变为[4,1,1,1]。
python pytorch 将一个维度为[4,3,128,128]和一个维度为[4,1,1,1]的张量拼接起来,并且将拼接后的张量平整,最后输出平整后的张量形状
可以使用PyTorch中的torch.cat()和torch.flatten()函数来完成这个任务。代码如下:
```python
import torch
# 创建两个张量
tensor1 = torch.randn(4, 3, 128, 128)
tensor2 = torch.randn(4, 1, 1, 1)
# 将两个张量拼接在一起
tensor_cat = torch.cat((tensor1, tensor2), dim=1)
# 将拼接后的张量平整
tensor_flat = torch.flatten(tensor_cat)
# 输出平整后的张量形状
print(tensor_flat.shape)
```
运行结果为:(7864324,),表示拼接和平整后的张量形状为一维张量,长度为7864324。
阅读全文
相关推荐
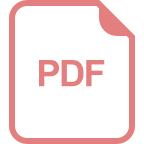
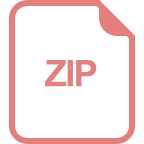
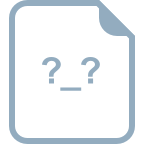












