Recall that an exponential random variable 𝑋~exp (𝜆) has mean 𝜆 1 and pdf given by: 𝑓(𝑥) = 𝜆𝑒 −𝜆𝑥 on 𝑥 ≥ 0. (b) Suppose that 𝑋1 and 𝑋2 are independent exponential random variables with mean 𝜆 1 . Let 𝑇 = min (𝑋1 ,𝑋2 ). Find the cdf of 𝑇. (Hint: what is 𝑃(𝑇 ≥ 𝑡)?)教我怎么做
时间: 2023-06-13 19:07:53 浏览: 111
首先,我们可以使用下面的公式计算 $P(T \geq t)$:
$$P(T \geq t) = P(X_1 \geq t, X_2 \geq t)$$
因为 $X_1$ 和 $X_2$ 是独立的,所以我们可以将概率分解为:
$$P(X_1 \geq t, X_2 \geq t) = P(X_1 \geq t) \cdot P(X_2 \geq t)$$
由于 $X_1$ 和 $X_2$ 都是指数分布,所以:
$$P(X_i \geq t) = e^{-\lambda_i t}, \ i = 1,2$$
因此,我们有:
$$P(T \geq t) = P(X_1 \geq t) \cdot P(X_2 \geq t) = e^{-\lambda_1 t} \cdot e^{-\lambda_2 t} = e^{-(\lambda_1 + \lambda_2) t}$$
现在我们可以使用 $P(T \geq t)$ 来计算 $T$ 的累积分布函数 (CDF):
$$F_T(t) = P(T \leq t) = 1 - P(T \geq t) = 1 - e^{-(\lambda_1 + \lambda_2) t}$$
因此,$T$ 的 CDF 为 $F_T(t) = 1 - e^{-(\lambda_1 + \lambda_2) t}$。
相关问题
Recall that an exponential random variable 𝑋~exp (𝜆) has mean 𝜆 1 and pdf given by: 𝑓(𝑥) = 𝜆𝑒 −𝜆𝑥 on 𝑥 ≥ 0. (a) Compute 𝑃(𝑋 ≥ 𝑥). (b) Suppose that 𝑋1 and 𝑋2 are independent exponential random variables with mean 𝜆 1 . Let 𝑇 = min (𝑋1 ,𝑋2 ). Find the cdf of 𝑇. (Hint: what is 𝑃(𝑇 ≥ 𝑡)?)
(a) 𝑃(𝑋 ≥ 𝑥) = ∫𝑥∞ 𝑓(𝑥) d𝑥 = ∫𝑥∞ 𝜆𝑒^−𝜆𝑥 d𝑥 = e^−𝜆𝑥
(b) 𝑃(𝑇 ≥ 𝑡) is the probability that both 𝑋1 and 𝑋2 are greater than or equal to 𝑡, which is given by:
𝑃(𝑇 ≥ 𝑡) = 𝑃(𝑋1 ≥ 𝑡)𝑃(𝑋2 ≥ 𝑡) = e^−𝜆1𝑡 * e^−𝜆1𝑡 = e^(−2𝜆1𝑡)
To find the cdf of 𝑇, we can use the fact that the cdf is the integral of the pdf:
𝐹𝑇(𝑡) = 𝑃(𝑇 ≤ 𝑡) = 1 − 𝑃(𝑇 ≥ 𝑡) = 1 − e^(−2𝜆1𝑡)
Therefore, the cdf of 𝑇 is 𝐹𝑇(𝑡) = 1 − e^(−2𝜆1𝑡).
autoanchor: thr=0.09: 1.0000 best possible recall, 9.00 anchors past thr
这段文字的意思是在进行目标检测时,使用了一种名为"autoanchor"的算法,设置了一个阈值(thr=0.09),并且在该阈值下获得了最佳的召回率(1.0000),超过了该阈值的9个anchors被认为是可行的。通常情况下,目标检测算法会将输入图像分割成多个较小的区域,这些区域被称为"anchors",然后算法会尝试在每个anchor中寻找目标物体。该算法使用"autoanchor"的目的是为了自适应地确定合适的anchor大小和数量,以提高检测精度。
阅读全文
相关推荐
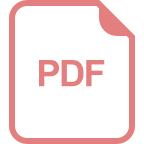
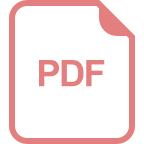
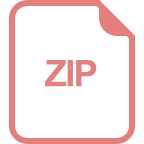













