Understanding Accuracy and Recall: Key Metrics in Machine Learning
发布时间: 2024-09-15 14:00:25 阅读量: 8 订阅数: 14 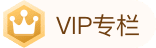
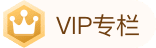
# 1. Fundamental Concepts of Precision and Recall
When discussing the performance of any machine learning model, two basic evaluation metrics are often mentioned: accuracy and recall. Accuracy is the ratio of the number of correctly predicted samples to the total number of samples, reflecting the overall extent to which the model predicts correctly. Recall measures the ability of the model to correctly identify positive class samples, that is, the proportion of true positives in all actual positive samples. For many application areas, such as medical diagnosis, fraud detection, and recommendation systems, accuracy and recall play a vital role. Understanding the basic concepts of these indicators is the first step in evaluating and optimizing the performance of machine learning models.
# 2. Theoretical Basis and Mathematical Principles
In the field of machine learning and data science, it is crucial to correctly understand the mathematical basis of classification problems and performance indicators. Precision and recall are two key indicators for evaluating the performance of classification models, which help us measure the model's performance in handling data classification tasks from different perspectives. This chapter will discuss these theoretical foundations and mathematical principles in detail and clarify how these concepts are applied in real-world situations through examples.
## 2.1 Classification Problems and Performance Indicators
### 2.1.1 Types of Classification Problems
Classification problems can be divided into two categories: binary classification problems and multi-class classification problems. In a binary classification problem, there are only two categories for the target variable, such as "spam" or "non-spam". In a multi-class classification problem, the target variable has three or more categories, such as the animal identification problem of "dog," "cat," and "horse."
### 2.1.2 Definitions and Importance of Performance Indicators
Performance indicators are used to measure the degree of fit between the model's predictions and the true situation. Accuracy and recall are among the most critical indicators.
Accuracy measures the proportion of true positives predicted by the model, while recall measures the proportion of positive cases identified by the model (actual positive samples). Understanding these two indicators is crucial for selecting an appropriate model to solve specific problems.
## 2.2 Mathematical Definitions of Precision and Recall
### 2.2.1 Formula for Calculating Precision
The formula for calculating precision is:
```
Precision = (True Positives TP + True Negatives TN) / (True Positives TP + False Positives FP + True Negatives TN + False Negatives FN)
```
Where TP (True Positive) represents true positives, FP (False Positive) represents false positives, TN (True Negative) represents true negatives, and FN (False Negative) represents false negatives.
### 2.2.2 Formula for Calculating Recall
The formula for calculating recall is:
```
Recall = True Positives TP / (True Positives TP + False Negatives FN)
```
This formula reflects the proportion of positive cases identified by the model in all actual positive cases.
### 2.2.3 Balance between the Two
In practical applications, there is often a trade-off between precision and recall. Improving one metric may lead to a decrease in the other. For example, in spam filtering, if we want to reduce false positives (i.e., marking real emails as spam), we might lower the threshold to increase recall, which also increases the risk of misclassifying non-spam emails as spam, thus lowering accuracy.
## 2.3 Confusion Matrix: Role and Application
### 2.3.1 Introduction to Confusion Matrix
A confusion matrix is a table used to visualize the performance of a classification model. In the confusion matrix, each row represents the true class of the instance, and each column represents the class predicted by the model. For a binary classification problem, a confusion matrix looks like this:
```
| | Predicted Positive | Predicted Negative |
|--------|--------------------|--------------------|
| Actual Positive | TP | FN |
| Actual Negative | FP | TN |
```
### 2.3.2 Relationship between Confusion Matrix and Performance Indicators
Each element in the confusion matrix is related to the performance indicators. For example, accuracy can be calculated by the ratio of the sum of TP and FP to the sum of the entire matrix.
### 2.3.3 Case Study Analysis of Confusion Matrix Interpretation
Consider a disease detection model where TP is the patients correctly identified as having the disease, TN is the non-patients correctly identified as healthy, FP is the healthy non-patients misdiagnosed as having the disease, and FN is the true patients who were not diagnosed.
If we have a confusion matrix:
```
| | Predicted Disease | Predicted Healthy |
|--------|-------------------|-------------------|
| Actual Disease | 80 | 20 |
| Actual Healthy | 10 | 90 |
```
Based on the above formula, we can calculate the accuracy and recall:
```
Accuracy = (80 + 90) / (80 + 20 + 10 + 90) = 0.875
Recall = 80 / (80 + 20) = 0.8
```
This section introduces the theoretical foundations of classification problems and their performance indicators. In the next chapter, we will further demonstrate how to use these concepts to evaluate and optimize model performance through examples in real-world applications.
# 3. Practical Application of Precision and Recall
After understanding the theoretical foundations of precision and recall, practical application becomes crucial. This chapter will delve into how to use these indicators to evaluate model performance, adjust models to optimize performance metrics, and analyze the application of precision and recall in different scenarios.
## 3.1 Evaluating Model Performance
Precision and recall provide important perspectives on the accuracy and completeness of model predictions. In practice, we need to evaluate the model's performance to determine its performance on specific tasks.
### 3.1.1 Model Selection and Performance Comparison
When selecting a model, we should look not only at its performance on the training set but more importantly on the validation and test sets. Typically, we build multiple models and compare their precision and recall to choose the best one.
For example, suppose we have three different classifiers A, B, and C, and we compare their performance on the test set:
- Classifier A has an accuracy of 85% and a recall of 70%.
- Classifier B has an accuracy of 80% and a recall of 85%.
- Classifier C has an accuracy of 75% and a recall of 90%.
By comparing, we can see that no model is the best in all aspects. Classifier A performs better in accuracy but is slightly inferior in recall compared to the other two. Classifier C has the highest recall but is not the best in accuracy. The choice of model depends on specific application requirements. If high accuracy is more important, classifier A might be chosen; if the priority is not to miss any positive sample, classifier C might be preferred.
### 3.1.2 Performance Evaluation in Real-World Cases
Performance evaluation in real-world cases usually requires more complex methods. We can use cross-validation to reduce the risk of overfitting and obtain a more accurate estimate of the model's generalization ability.
Suppose we are building a spam filter with a large amount of data marked as "spam" or "non-spam." Using cross-validation, we divide the data into K subsets and repeatedly train the model with K-1 subsets and evaluate it with the remaining subset. In this way, we can obtain the model's average performance on unseen data.
```python
from sklearn.model_selection import cross_val_score
from sklearn.feature_extraction.text import TfidfVectorizer
from sklearn.naive_bayes import MultinomialNB
# Assuming 'data' is a DataFrame containing email content and labels
X = data['email_text']
y = data['label']
# Convert text to TF-IDF feature vectors
vectorizer = TfidfVectorizer()
X_vectorized = vectorizer.fit_transform(X)
# Perform cross-validation with a Multinomial Naive Bayes classifier
clf = MultinomialNB()
scores = cross_val_score(clf, X_vectorized, y, cv=5)
print("Accuracy scores for each fold: ", scores)
print("Average accuracy: ", scores.mean())
```
In the above Python code, we first convert email text into TF-IDF feature vectors, then perform 5-fold cross-validation with a Naive Bayes classifier. Finally, we obtain the accuracy for each fold and the average accuracy.
Through this method, we can gain a more comprehensive understanding of the model's performance and optimize it further if necessary.
## 3.2 Adjusting the Model to Optimize Indicators
After understanding how to evaluate the model's performance, the next step is to adjust the model to optimize precision and recall.
### 3.2.1 Strategies for Model Parameter Adjustment
Model parameter adjustment is an important step in improving model performance. Different algorithms have different parameters, and these parameters affect accuracy and recall differently.
Taking logistic regression as an example, we would typically adjust the regularization strength (C parameter) and the type of regularization (penalty parameter, such as L1 or L2). A smaller C value increases the strength of regularization, which may lead the model to reduce overfitting, thereby increasing the model's recall, but it may sacrifice some accuracy.
```python
from sklearn.linear_model import LogisticRegression
# Use a logistic regression classifier and set different C values for comparison
clf1 = LogisticRegression(C=1.0, penalty='l2')
clf2 = LogisticRegression(C=0.1, penalty='l2')
# Compare the performance of the model under different C values
scores1 = cross_val_score(clf1, X_vectorized, y, cv=5)
scores2 = cross_val_score(clf2, X_vectorized, y, cv=5)
print("Accuracy and recall for model 1: ", scores1.mean(), ", ", scores1.std())
print("Accuracy and recall for model 2: ", scores2.mean(), ", ", scores2.std())
```
### 3.2.2 Hyperparameter Optimization Methods
Hyperparameter optimization is an advanced topic for improving model performance. Here, we can use methods such as Grid Search (GridSearchCV) or Randomized Search (RandomizedSearchCV) to automatically find the best combination of parameters.
```python
from sklearn.model_selection import GridSearchCV
# Set the parameter space for logistic regression
param_grid = {'C': [0.1, 1, 10], 'penalty': ['l1', 'l2']}
# Build a GridSearchCV object
grid_search = GridSearchCV(LogisticRegression(), param_grid, cv=5)
grid_search.fit(X_vectorized, y)
print("Best parameters: ", grid_search.best_params_)
```
With grid search, we can try every possible combination of parameters in the preset parameter space and choose the best combination based on the results of cross-validation.
### 3.2.3 Tuning Cases in Practical Operations
In practical operations, we may need to fine-tune multiple hyperparameters. For example, if we use a Support Vector Machine (SVM) classifier, we may need to adjust both the C parameter and the type of kernel function.
```python
from sklearn.svm import SVC
# Set the parameter space for SVM
param_grid = {'C': [0.1, 1, 10], 'kernel': ['linear', 'rbf']}
# Build a GridSearchCV object
grid_search = GridSearchCV(SVC(), param_grid, cv=5)
grid_search.fit(X_vectorized, y)
print("Best parameters: ", grid_search.best_params_)
```
After running this code, we would use the model with the best parameter combination for the final evaluation based on the output of the best parameters. This often yields better model performance than the default parameters.
## 3.3 Application Scenario Analysis
The application of precision and recall is not limited to a single scenario. Understanding how to apply these indicators in different fields is crucial for the deployment of models in practice.
### 3.3.1 Application of Precision and Recall in Different Fields
In the field of medical diagnosis, recall may be more important because missing a diagnosis can lead to serious consequences. In contrast, in spam filtering, accuracy may be more important because users would rather see a spam email than miss an important one.
### 3.3.2 Adjusting Performance Indicators for Specific Scenarios
Adjusting performance indicators according to specific scenarios is key to enhancing the practical utility of the model. For example, in credit scoring, we can give a higher weight to accuracy to reduce the risk of bad debt.
### 3.3.3 Discussion of Real-World Cases
Let's take the shopping basket analysis of an online retail website as an example. Precision (predicting whether a user will purchase a particular item) and recall (recalling all the items a user actually wants to purchase) are both very important in personalized recommendation systems.
By analyzing users' purchase histories, we can build a model to predict items a user may be interested in. We can use precision to evaluate the accuracy of recommendations and use recall to evaluate the completeness of recommendations. By optimizing these two indicators, we can increase user satisfaction and boost sales.
The practical application of precision and recall is an important step in transforming theory into practical results. In the following chapters, we will further explore advanced applications of precision and recall and future trends.
# 4. Advanced Discussion on Precision and Recall
In the previous chapters, we introduced the basic concepts, theoretical foundations, and practical applications of precision and recall, along with case analyses. With a deeper understanding of machine learning model performance evaluation, this chapter will lead readers into a more advanced discussion of performance indicators and potential challenges and solutions in practical applications.
## 4.1 Other Related Performance Indicators
While precision and recall are the basic indicators for evaluating classification models, in complex models and diverse application scenarios, we often need to consider more dimensions of performance indicators to comprehensively evaluate model performance.
### 4.1.1 Introduction and Calculation of the F1 Score
The F1 score is the harmonic mean of precision and recall, taking into account the importance of both. The F1 score is defined as:
```
F1 = 2 * (precision * recall) / (precision + recall)
```
Where `precision` represents precision, and `recall` represents recall. The F1 score ranges from [0, 1], and the closer the value is to 1, the better the performance. The introduction of the F1 score is particularly useful when dealing with imbalanced data.
### 4.1.2 Relationship between Precision, Recall, and F1 Score
There is a close relationship between precision, recall, and the F1 score. In some cases, we need to balance these three to achieve the best model performance. For example, in applications sensitive to false positives, we may value precision more; in applications sensitive to false negatives, recall is more important. The F1 score offers a middle ground solution, giving a lower score when both precision and recall are low, encouraging the model to find a balance between them.
### 4.1.3 Analysis of ROC Curve and AUC Value
The ROC curve (Receiver Operating Characteristic) is a powerful tool that displays model performance through the true positive rate (TPR) and false positive rate (FPR) at different thresholds. The area under the ROC curve (AUC value) is an important indicator for evaluating the model, with a value closer to 1 indicating better classification performance.
```
AUC = 0.5 for a random model
AUC > 0.7 indicates that the model has some predictive ability
AUC > 0.9 indicates that the model has very good predictive ability
```
### Code Block and Parameter Explanation
The following is an example Python code that draws the ROC curve and calculates the AUC value.
```python
from sklearn.metrics import roc_curve, auc
from sklearn import datasets
import numpy as np
import matplotlib.pyplot as plt
# Load the example dataset
iris = datasets.load_iris()
X = iris.data[:, 2] # Only use petal length
y = iris.target
# Use only the binary classification problem
X, y = X[:, np.newaxis], y
y = y == 2
# Predict probabilities
rf = RandomForestClassifier(n_estimators=100)
proba = rf.fit(X, y).predict_proba(X)
# Calculate ROC curve and AUC value
fpr, tpr, thresholds = roc_curve(y, proba[:, 1])
roc_auc = auc(fpr, tpr)
# Plotting
plt.figure()
lw = 2
plt.plot(fpr, tpr, color='darkorange', lw=lw, label='ROC curve (area = %0.2f)' % roc_auc)
plt.plot([0, 1], [0, 1], color='navy', lw=lw, linestyle='--')
plt.xlim([0.0, 1.0])
plt.ylim([0.0, 1.05])
plt.xlabel('False Positive Rate')
plt.ylabel('True Positive Rate')
plt.title('Receiver Operating Characteristic')
plt.legend(loc="lower right")
plt.show()
```
Logical Analysis: The code first loads the iris dataset and performs simple data preprocessing. Then, using the Random Forest classifier for model training, it obtains the model's predicted probabilities. The roc_curve function is used to calculate the true positive rate and false positive rate, and the auc function is used to calculate the AUC value. Finally, matplotlib is used to plot the ROC curve and display the AUC value.
## 4.2 Advanced Strategies for Indicator Optimization
When dealing with complex datasets, we often need to adopt some advanced strategies to optimize performance indicators.
### 4.2.1 Considerations for Multi-Label Classification Problems
In multi-label classification problems, an instance may belong to multiple classes. In such problems, the definitions of precision and recall need to be extended. For each label, we can calculate its precision and recall separately, and then average or weighted average all the labels.
### 4.2.2 Model Integration and Performance Indicators
Model integration methods, such as bagging, boosting, stacking, etc., can improve prediction performance by combining multiple models. When evaluating integrated models, in addition to precision and recall, we also need to consider the impact of the integration strategy on the overall model's generalization ability.
### 4.2.3 Methods for Handling Imbalanced Datasets
When faced with imbalanced datasets, accuracy may be misleading due to the presence of majority classes. In such cases, we can adopt different strategies, such as changing evaluation criteria, adjusting class weights, and using different types of sampling methods.
## 4.3 Challenges and Solutions in Real-World Applications
When applying precision, recall, and related indicators to real-world problems, we often encounter various challenges. This section will propose possible solutions to these challenges.
### 4.3.1 Handling Bias and Noise in Real Data
In the real world, data often contains bias and noise, which can affect the performance evaluation of the model. Coping strategies include data cleaning, feature engineering, and using robust algorithms.
### 4.3.2 Challenges in the Indicator Optimization Process
Indicator optimization may lead to a decrease in the model's generalization ability. We need to find a balance between optimizing indicators and maintaining the model's generalization ability. This requires a deep understanding of business needs and careful tuning of parameters during model training.
### 4.3.3 Indicator Adjustment Strategies Based on Business Logic
The selection and optimization strategy for indicators should be closely related to business logic. Different business needs require different methods for model performance evaluation. For example, in medical diagnosis applications, the importance of recall may far outweigh accuracy.
In the process of understanding and addressing these challenges, we continuously gain deeper insights into model performance evaluation and improve the accuracy and practicality of models in practice.
# ***prehensive Case Studies and Future Prospects
## 5.1 Comprehensive Case Studies
After gaining an in-depth understanding of the theoretical foundations and practical applications of precision and recall, we will further explore how these two indicators function in real-world problems through a comprehensive case study.
### 5.1.1 In-depth Analysis of Industry Cases
Consider a typical e-commerce scenario where we need to build a recommendation system that can predict products that users may be interested in. In this example, the degree of match between the recommendation list output by the recommendation system (i.e., the model's prediction results) and the actual list of products purchased by users (i.e., the true results) can be evaluated using precision and recall.
When building the recommendation system model, we may encounter the problem of data imbalance, where the number of products purchased by users compared to those not purchased is a smaller proportion. In such cases, using accuracy as the sole evaluation criterion may lead to misleading results because the model may predict that all users will not purchase any products, resulting in high accuracy but low recall.
### 5.1.2 Analysis of the Application of Precision and Recall in the Case
In this recommendation system case, precision (Precision) is the proportion of products actually purchased in the recommended list, and recall (Recall) is the proportion of products purchased that are recommended by the model out of all the products purchased. Using these indicators, we can understand the model's performance in identifying products that users may be interested in.
```python
# The following is a pseudo-example of building a recommendation system:
# Assuming we have the following dataset:
# User purchase data (userId, productId)
# Recommendation system output data (userId, recommended product list)
# User actual purchase data (userId, actual product purchase list)
# Precision calculation
def calculate_precision(recommended, actual):
true_positives = len(set(recommended).intersection(set(actual)))
return true_positives / len(recommended) if recommended else 0
# Recall calculation
def calculate_recall(recommended, actual):
true_positives = len(set(recommended).intersection(set(actual)))
return true_positives / len(actual) if actual else 0
recommended_list = [...] # Recommended products list generated by the recommendation system for a user
actual_purchase_list = [...] # Actual product purchase list for a user
precision = calculate_precision(recommended_list, actual_purchase_list)
recall = calculate_recall(recommended_list, actual_purchase_list)
```
In real-world applications, the recommendation system may adopt more complex algorithms and a large amount of user behavior data to improve the accuracy and relevance of recommendations. However, the goal remains to improve precision and recall and find a balance between the two, thereby enhancing user experience and sales performance for merchants.
## 5.2 Technological Development Trends and Challenges
### 5.2.1 Current Trends in Machine Learning Technology Development
With the development of deep learning, the measurement of performance indicators such as precision and recall has become more complex. Current trends include using neural networks to solve complex pattern recognition problems, such as natural language processing and computer vision, which require more advanced evaluation techniques to measure model performance.
### 5.2.2 Application of Precision and Recall in New Technologies
In these emerging fields, precision and recall still play a vital role, but they come with additional challenges. For example, when dealing with natural language rich in semantics and context dependence, simple classification accuracy may not capture the subtle differences in the model's understanding of semantics.
### 5.2.3 Future Technical Challenges in Machine Learning
In the future, researchers in the field of machine learning will face challenges in dealing with larger datasets, more complex models, and adapting to ever-changing environments. In this process, traditional indicators such as precision and recall may be combined with new indicators to form a more comprehensive performance evaluation system. At the same time, how to optimize these indicators in the constantly changing business environment is also a concern that needs to be addressed in future development.
0
0