Avoiding the Accuracy Pitfall: Evaluating Indicators with Support Vector Machines
发布时间: 2024-09-15 14:09:13 阅读量: 30 订阅数: 41 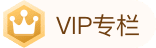
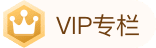
# 1. Support Vector Machine Fundamentals
Support Vector Machine (SVM) is a machine learning method developed on the basis of statistical learning theory. It is widely used in classification and regression analysis. The core idea of SVM is to find an optimal hyperplane to correctly classify data points of different categories, maximizing the margin between different categories. It can handle both linearly separable and nonlinearly separable data and has shown superior performance in many practical applications.
In the first chapter, we first introduce the basic concepts of SVM, then explore its unique advantages and basic working principles in data classification. We will use simple examples to explain the core idea of SVM, building a preliminary understanding of SVM for readers.
## 1.1 Basic Concepts of SVM
Support Vector Machine (SVM) is a supervised learning model used to solve classification problems. It separates datasets into two categories by finding a hyperplane. The choice of hyperplane needs to maximize the margin between two categories of data, that is, the "maximum margin" principle. In the ideal case, the classification margin is the largest, meaning that the hyperplane can be as far away from the nearest data points as possible, thereby improving the model's generalization ability.
## 1.2 Core Advantages of SVM
A significant advantage of SVM is its excellent generalization ability, especially outstanding when the feature space dimension is much larger than the number of samples. In addition, SVM introduces the kernel trick, which allows SVM to effectively deal with nonlinearly separable problems. By nonlinearly mapping the data, SVM can find a linear decision boundary in a high-dimensional space, thereby achieving nonlinear classification in the original space.
On this basis, we will delve into the principles and applications of SVM, laying a solid theoretical foundation for the in-depth analysis of SVM theory, discussion of evaluation metrics, and introduction of practical applications in subsequent chapters.
# 2. Theoretical Foundations and Mathematical Principles of Support Vector Machines
## 2.1 Linearly Separable Support Vector Machines
### 2.1.1 Linearly Separable Problems and Hyperplanes
Linearly separable problems are a special case of classification problems in machine learning. In such cases, samples of two categories can be completely separated by a hyperplane. Mathematically, if we have an n-dimensional feature space, then the hyperplane can be represented as an (n-1)-dimensional subspace. For example, in two-dimensional space, the hyperplane is a straight line; in three-dimensional space, the hyperplane is a plane.
In Support Vector Machines (SVM), finding this hyperplane is crucial. We hope to find a hyperplane that not only correctly separates the two types of data but also has the largest margin (the distance from the hyperplane to the nearest data points, support vectors, is as large as possible). The purpose of doing this is to obtain better generalization ability, that is, to perform better on unseen data.
### 2.1.2 Definition and Solution of Support Vectors
Support vectors are the training data points closest to the decision boundary. They directly determine the position and direction of the hyperplane and are the most critical factors in forming the optimal decision boundary. When solving linearly separable SVMs, the goal is to maximize the margin between the two categories.
The solution to support vector machines can be accomplished through an optimization problem. Specifically, we need to solve the following optimization problem:
\begin{aligned}
& \text{minimize} \quad \frac{1}{2} \|\mathbf{w}\|^2 \\
& \text{subject to} \quad y_i (\mathbf{w} \cdot \mathbf{x}_i + b) \geq 1, \quad i = 1, \ldots, m
\end{aligned}
Where $\mathbf{w}$ is the normal vector of the hyperplane, $b$ is the bias term, $y_i$ is the class label, $\mathbf{x}_i$ is the sample point, and $m$ is the number of samples. The constraints of this optimization problem ensure that all sample points are correctly classified and that the distance from the hyperplane is at least 1.
The above optimization problem is typically solved using the Lagrange multiplier method, transforming it into a dual problem for solution. The solution will give a model determined by the support vectors and their corresponding weights.
## 2.2 Kernel Trick and Non-Linear Support Vector Machines
### 2.2.1 Concept and Types of Kernel Functions
The concept of kernel functions is the core of SVM's ability to handle nonlinear problems. Kernel functions can map the original feature space to a higher-dimensional feature space, making data that is not linearly separable in the original space linearly separable in the new space.
An important property of kernel functions is that they do not need to explicitly calculate the high-dimensional feature vectors after mapping, but achieve im***mon types of kernel functions include linear kernel, polynomial kernel, Gaussian Radial Basis Function (RBF) kernel, and sigmoid kernel, among others.
Taking the Gaussian RBF kernel as an example, its mathematical expression is as follows:
K(\mathbf{x}, \mathbf{z}) = \exp\left(-\gamma \|\mathbf{x} - \mathbf{z}\|^2\right)
Where $\mathbf{x}$ and $\mathbf{z}$ are two sample points, and $\gamma$ is the parameter of the kernel function. The RBF kernel can control the distribution of the mapped data by adjusting the value of $\gamma$ to control the "influence range" of sample points.
### 2.2.2 Application of the Kernel Trick in Non-Linear Problems
By introducing kernel functions, support vector machines can be extended from linear classifiers to nonlinear classifiers. When dealing with nonlinear problems, SVM uses the kernel trick to implicitly construct hyperplanes in high-dimensional spaces.
The application of the kernel trick in nonlinear SVMs can be summarized in the following steps:
1. Select an appropriate kernel function and its corresponding parameters.
2. Use the kernel function to calculate the inner product between sample points in the high-dimensional space.
3. Construct an optimization problem in the high-dimensional space and solve it to obtain the hyperplane.
4. Define the final classification decision function using support vectors and weights.
The effectiveness of the kernel trick depends on whether the selected kernel function can map to a feature space in which the sample points become linearly separable. Through the kernel trick, SVM has shown strong capabilities in dealing with complex nonlinear classification problems in image recognition, text classification, and other fields.
## 2.3 Support Vector Machine Optimization Problems
### 2.3.1 Introduction to Lagrange Multiplier Method
The Lagrange multiplier method is an effective method for solving optimization problems with constraint conditions. In support vector machines, by introducing Lagrange multipliers (also known as Lagrange dual variables), the original problem can be transformed into a dual problem, which is easier to solve.
The original optimization problem can be written in the following form:
\begin{aligned}
& \text{minimize} \quad \frac{1}{2} \|\mathbf{w}\|^2 \\
& \text{subject to} \quad y_i (\mathbf{w} \cdot \mathbf{x}_i + b) \geq 1, \quad i = 1, \ldots, m
\end{aligned}
Using the Lagrange multiplier method, we construct the Lagrange function:
L(\mathbf{w}, b, \alpha) = \frac{1}{2} \|\mathbf{w}\|^2 - \sum_{i=1}^{m} \alpha_i \left( y_i (\mathbf{w} \cdot \mathbf{x}_i + b) - 1 \right)
Where $\alpha_i \geq 0$ are Lagrange multipliers. Next, by taking the partial derivative of $L$ with respect to $\mathbf{w}$ and $b$ and setting the derivative to zero, we can obtain the expressions for $\mathbf{w}$ and $b$.
### 2.3.2 Dual Problem and KKT Conditions
The dual problem obtained by the Lagrange multiplier method is the equivalent form of the original problem and is usually easier to solve. The goal of the dual problem is to maximize the expression of the Lagrange function with respect to the Lagrange multipliers, while satisfying certain conditions.
\begin{aligned}
& \text{maximize} \quad \sum_{i=1}^{m} \alpha_i - \frac{1}{2} \sum_{i, j=1}^{m} y_i y_j \alpha_i \alpha_j \mathbf{x}_i \cdot \mathbf{x}_j \\
& \text{subject to} \quad \alpha_i \geq 0, \quad i = 1, \ldots, m \\
& \quad \quad \sum_{i=1}^{m} y_i \alpha_i = 0
\end{aligned}
This problem is a quadratic programming problem about the Lagrange multipliers $\alpha_i$ and can be solved by existing optimization algorithms.
After solving the dual problem, we also need to check whether the Karush-Kuhn-Tucker (KKT) conditions are met. The KKT conditions are the necessary conditions for the optimization problem of support vector machines, including:
- Smoothness conditions
- Stationarity conditions
- Dual feasibility conditions
- Primal feasibility conditions
If all KKT conditions are met, then the optimal solution to the original problem is found.
### 2.3.3 Code Implementation for Solving the Dual Problem
Below is a simple example code using Python's `cvxopt` library to solve the SVM dual problem:
```python
import numpy as np
from cvxopt import matrix, solvers
# Training data, X is the feature matrix, y is the label vector
X = np.array([[1, 2], [2, 3], [3, 3]])
y = np.array([-1, -1, 1])
# Calculate the kernel matrix
def kernel_matrix(X, gamma=0.5):
K = np.zeros((X.shape[0], X.shape[0]))
for i in range(X.shape[0]):
for j in range(X.shape[0]):
K[i, j] = np.exp(-gamma * np.linalg.norm(X[i] - X[j]) ** 2)
return K
# Construct Lagrange multipliers
K = kernel_matrix(X)
P = matrix(np.outer(y, y) * K)
```
0
0
相关推荐








