Statistical Tests for Model Evaluation: Using Hypothesis Testing to Compare Models
发布时间: 2024-09-15 14:40:58 阅读量: 47 订阅数: 31 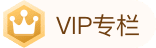
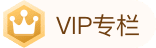
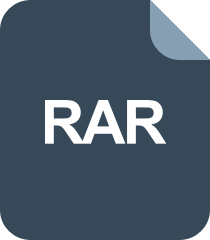
Applied_Linear_Statistical_Models.rar_Statistical Models_site:ww

# Basic Concepts of Model Evaluation and Hypothesis Testing
## 1.1 The Importance of Model Evaluation
In the fields of data science and machine learning, model evaluation is a critical step to ensure the predictive performance of a model. Model evaluation involves not only the production of accurate predictions but also a profound understanding of the model's stability and generalization capabilities. Hypothesis testing, as a core concept in statistics, plays a key role in model evaluation. It allows us to infer model parameters based on existing data and test their statistical significance, thereby quantifying the reliability and predictive power of the model.
## 1.2 Introduction to Hypothesis Testing
Hypothesis testing is a statistical method used to infer population parameters based on sample data. In the context of model evaluation, it often involves constructing statistical hypotheses about model parameters and using data to decide whether to reject these hypotheses. This process typically includes setting up a null hypothesis (H0) and an alternative hypothesis (H1), and then calculating a p-value to determine whether to reject the null hypothesis. The p-value is the probability of observing the statistical result or something more extreme, and if the p-value is less than the significance level (usually 0.05), then the null hypothesis is rejected, considering the observed effect to be statistically significant.
## 1.3 The Relationship between Model Evaluation and Hypothesis Testing
In model evaluation, hypothesis testing is often used to verify whether the model's assumptions are met, such as linear relationships and normally distributed residuals. Additionally, hypothesis testing can be used to compare the predictive performance of different models, such as using cross-validation methods to test if there is a significant performance difference between two models. Ultimately, the goal of model evaluation and hypothesis testing is to ensure that the model performs well not only on sample data but also maintains consistent performance on new datasets, thereby achieving effective prediction and decision-making.
# Theoretical Basis of Statistical Tests
## 2.1 Concepts and Types of Statistical Hypotheses
A statistical hypothesis is the starting point for inferring population parameters in statistics. They are typically divided into two types: null and alternative hypotheses.
### 2.1.1 Definitions of Null and Alternative Hypotheses
The **null hypothesis** (H0) generally represents a state of no effect, no difference, or no association. It is the default state of the test, meaning that we assume there is no effect or difference until the evidence is sufficiently strong.
The **alternative hypothesis** (H1 or Ha) is opposite to the null hypothesis, indicating the presence of an effect, a difference, or some association. The alternative hypothesis is accepted after the null hypothesis has been rejected.
### 2.1.2 Differences between Two-Sided and One-Sided Tests
When conducting statistical tests, different test methods are used according to the needs of the research design:
The **two-sided test** is used to test whether sample data significantly differ from the population parameters, without considering the direction of the difference (i.e., larger or smaller).
The **one-sided test** is used to test whether sample data significantly greater or less than the population parameters, thus it is concerned with the direction of the difference.
## 2.2 Common Statistical Test Methods
### 2.2.1 Parametric and Nonparametric Tests
**Parametric tests** require that the data meet certain assumptions (e.g., normal distribution) and use sample data distribution parameters (such as mean and variance) for inference.
**Nonparametric tests** do not rely on the specific form of the population distribution and are suitable for situations that do not meet the conditions of parametric tests, such as unknown data distributions or those that significantly deviate from normal distribution.
### 2.2.2 Determining the Rejection and Acceptance Regions
When performing statistical tests, a **rejection region** (critical region) must be determined. If the test statistic falls into the rejection region, the null hypothesis is rejected. Otherwise, the null hypothesis is accepted.
The **acceptance region** is the area where the null hypothesis is not rejected, and it, along with the rejection region, constitutes all possibilities for decision-making.
## 2.3 Probability and Decision-Making Process of Statistical Tests
### 2.3.1 Types of Errors: Type I and Type II
A **Type I error** occurs when the null hypothesis is actually true but is incorrectly rejected, falsely assuming a significant difference or association. The probability of a Type I error is usually denoted by α.
A **Type II error** occurs when the null hypothesis is actually false, but not rejected. The probability of a Type II error is denoted by β, and 1-β represents **power**, which is the probability of correctly rejecting a false null hypothesis.
### 2.3.2 Significance Level and Power Analysis
The **significance level** (α level) is a predetermined threshold used to determine whether the results of a statistical test are statistically significant. Typically, α is set to 0.05 or 0.01.
**Power analysis** (power analysis) is used to evaluate the probability of correctly rejecting a false null hypothesis under specific effect sizes, α levels, and sample sizes. It helps determine the appropriate sample size and the power of statistical tests.
### 2.3.3 Calculation of Test Statistics and p-Values
Test statistics are calculated based on sample data and are used to test the null hypothesis. The calculation method of test statistics depends on the type of test selected and the distribution of the data.
A **p-value** (probability value) is the probability of observing the statistic or something more extreme under the condition that the null hypothesis is true. A small p-value means that the observed data are unlikely to be produced by random fluctuations alone, thereby providing evidence to reject the null hypothesis.
In practical applications, a threshold is usually set (e.g., 0.05), and if the p-value is less than this threshold, we reject the null hypothesis, considering the observed effect to be statistically significant.
# Hypothesis Testing Methods in Model Evaluation
### 3.1 Hypothesis Testing for Model Accuracy Evaluation
Accuracy is a key indicator of a model's predictive performance, measuring the correctness of the model's predictions. To scientifically evaluate a model's accuracy, hypothesis testing methods are often used to determine whether the model's predictive results are significantly better than random guessing.
#### 3.1.1 Applications of F-tests and t-tests in Model Evaluation
F-tests and t-tests are common parametric tests in statistics, used to assess the statistical significance of a model.
- The **t-test** is typically used to compare the mean differences between two independent samples or between a single sample mean and a known value. In model evaluation, we may use a one-sample t-test to determine if the model's predicted mean significantly differs from the actual values.
- The **F-test** is primarily used to compare the differences in variances between two or more samples and is commonly used in regression analysis to test the significance of the overall fit of a regression model. For example, in a multiple linear regression model, the F-test can help us determine if at least one explanatory variable has a statistically significant effect on the response variable.
```r
# R code example: One-sample t-test
# Assuming 'data' is a data frame containing model predicted values and actual values, where the Actual column contains actual values
t_test_result <- t.test(data$Predicted, mu=data$Actual, alternative="two.sided")
print(t_test_result)
```
In the above code, the `t.test` function is used for the one-sample t-test. The `mu` parameter is set to the mean of the actual values, and `alternative="two.sided"` indicates that we are interested in a two-tailed test.
- The application of the **F-test** is broader and can be used to determine if all explanatory variables as a whole significantly explain the model.
```r
# R code example: F-test
# Assuming 'lm_model' is a linear model fitted using the lm function
f_test_result <- anova(lm_model)
print(f_test_result)
```
In R, the `anova` function is used to perform variance analysis on linear models, and the results of the F-test will provide statistical evidence of whether the explanatory variables have a significant effect on the response variable.
#### 3.1.2 Applications of Chi-Square Test in Classification Models
In the evaluation of classification models, the Chi-square test is often used to examine whether there is an independent relationship between the predicted results of the classification model and the actual values. When the model predicts categorical variables, the Chi-square test is particularly useful.
```python
# Python code example: Chi-square test
from scipy.stats import chi2_contingency
# Assuming 'observed' is a two-dimensional array containing the frequency table of model predicted values and actual values
chi2, p, dof, expected = chi2_contingency(observed)
print(f"Chi-square statistic: {chi2}")
print(f"P-value: {p}")
```
In this example, the `chi2_contingency` function from the scipy library is used to perform the Chi-square test. The given observed frequency table (`observed`) is used to calculate the Chi-square statistic and the corresponding P-value.
### 3.2 Hypothesis Testing for Model Stability Evaluation
The stability of a model refers to its consistency and reliability under differ
0
0
相关推荐
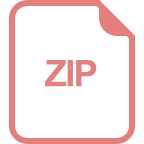
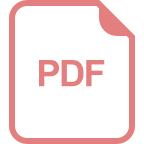
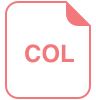
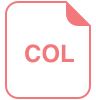
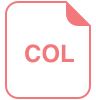
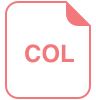
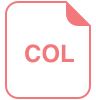
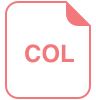