Feature Selection: Master These 5 Methodologies to Revolutionize Your Models
发布时间: 2024-09-15 11:15:15 阅读量: 10 订阅数: 17 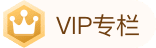
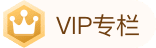
# Feature Selection: Master These 5 Methodologies to Transform Your Models
## 1. Theoretical Foundations of Feature Selection
### 1.1 Importance of Feature Selection
Feature selection is a critical step in machine learning and data analysis, aimed at choosing a subset of features from the original dataset that most aid in the construction of predictive models. In this process, we not only eliminate irrelevant or redundant features to reduce model complexity but also retain those that have predictive power for the target variable, thereby enhancing model performance.
### 1.2 Objectives of Feature Selection
Effective feature selection can reduce data dimensions, decrease model training time, enhance model interpretability, prevent overfitting, and improve the generalization ability of the model. It helps us find an optimal balance point in the vast feature space.
### 1.3 Challenges of Feature Selection
Despite the many benefits of feature selection, challenges arise in practical operations. Determining the relationship between features and the target variable, evaluating the importance of features, and handling inter-feature dependencies are all issues that need to be addressed during feature selection.
In this chapter, we will explore the theoretical foundations of feature selection, providing the necessary theoretical support for specific feature selection methods in subsequent chapters.
# 2. Feature Selection Methods Based on Statistical Tests
## 2.1 Univariate Statistical Tests
In feature selection, univariate statistical tests are a simple yet effective method that evaluates the relationship between a single feature and the target variable. This method assumes that features are independent and attempts to identify those with significant statistical relationships to the target variable.
### 2.1.1 Chi-Square Test
The Chi-square test is a commonly used hypothesis testing method in statistics, used to determine if there is a statistically significant correlation between two categorical variables. In feature selection, the Chi-square test can be used to select categorical features.
#### Applying the Chi-square Test for Feature Selection
```python
from sklearn.feature_selection import SelectKBest, chi2
from sklearn.datasets import load_iris
# Load dataset
iris = load_iris()
X, y = iris.data, iris.target
# Select top 5 features using Chi-square test
select = SelectKBest(chi2, k=5)
X_kbest = select.fit_transform(X, y)
# Output selected features
selected_features = iris.feature_names[select.get_support()]
print(selected_features)
```
In the above code, we use the `SelectKBest` class, select the Chi-square test (`chi2`) as the scoring function, and specify selecting the top 5 features. The `fit_transform` method performs feature selection, and the `get_support` method returns a boolean array indicating which features are selected.
### 2.1.2 T-test
The T-test is used to compare the mean differences of two independent samples. In feature selection, the T-test is commonly used for continuous features to identify which features have a significant difference from the target variable's mean.
#### Applying the T-test for Feature Selection
```python
from sklearn.feature_selection import SelectKBest, f_classif
# Select top 5 features using ANOVA F-value
select = SelectKBest(f_classif, k=5)
X_kbest = select.fit_transform(X, y)
# Output selected features
selected_features = iris.feature_names[select.get_support()]
print(selected_features)
```
Here, we use ANOVA F-value (`f_classif`) as the scoring function, which is applicable to classification tasks and can identify features that impact the target variable.
### 2.1.3 ANOVA
Analysis of variance (ANOVA) is a statistical technique used to test if there are statistically significant differences between the means of three or more samples. In feature selection, ANOVA can be used to identify features that show different means across different categories.
#### Applying ANOVA for Feature Selection
```python
from scipy.stats import f_oneway
# Assume X and y are the features and labels obtained from the dataset
feature_groups = []
for feature in range(len(iris.feature_names)):
f_value, p_value = f_oneway(X[:, feature], y)
feature_groups.append((iris.feature_names[feature], f_value, p_value))
# Sort features by ANOVA F-values
feature_groups = sorted(feature_groups, key=lambda x: x[1], reverse=True)
print("Top features by ANOVA F-values:")
for feature in feature_groups[:5]:
print(f"{feature[0]} F-value: {feature[1]} P-value: {feature[2]}")
```
With the above code, we perform ANOVA testing on each feature and sort them by F-values. Note that ANOVA is a more complex statistical testing method used in feature selection to identify features that differentiate between different categories.
## 2.2 Multivariate Statistical Tests
Multivariate statistical tests differ from univariate tests as they evaluate the relationship between multiple features and the target variable. These methods are better suited to address issues of inter-feature dependencies.
### 2.2.1 Correlation Analysis
Correlation analysis is a statistical tool used to study the linear relationship between two continuous variables. In feature selection, common correlation coefficients include the Pearson correlation coefficient and the Spearman's rank correlation coefficient.
#### Applying Pearson Correlation Coefficient for Feature Selection
```python
import pandas as pd
import seaborn as sns
# Convert data to DataFrame for correlation analysis
df = pd.DataFrame(X, columns=iris.feature_names)
corr_matrix = df.corr()
# Plot heatmap of the correlation matrix
plt.figure(figsize=(10, 8))
sns.heatmap(corr_matrix, annot=True, cmap='coolwarm')
plt.title("Correlation Matrix Heatmap")
plt.show()
```
By plotting the heatmap of the correlation matrix, we can visually see the correlations between different features. In feature selection, we tend to remove features that are highly correlated with others to avoid multicollinearity issues.
### 2.2.2 Partial Correlation Analysis
Partial correlation analysis measures the linear relationship between two variables while controlling for the influence of other variables. This is particularly useful in feature selection as it helps identify features that are still related to the target variable after eliminating the effects of other variables.
#### Steps of Partial Correlation Analysis
1. Calculate the correlation of all features with the target variable.
2. For each pair of features, compute a conditional correlation, i.e., the correlation between the two variables when controlling for a third variable.
3. Perform feature selection based on conditional correlations.
Due to the complexity of partial correlation analysis, specialized statistical software or packages are often required. In Python, the advanced functions of the `numpy` and `scipy` libraries can be used to calculate it.
### 2.2.3 Path Analysis
Path analysis is an extended regression analysis method aimed at evaluating causal relationships between variables. In feature selection, path analysis can help us identify features that have a direct impact on the target variable.
#### Steps of Path Analysis
1. Determine potential causal relationship models.
2. Fit the model using structural equation modeling (SEM).
3. Assess the paths between variables through model fit goodness.
In Python, the `sem` module in the `statsmodels` library can be used to perform path analysis. However, path analysis usually requires domain knowledge to design a reasonable model structure.
The above introduces feature selection methods based on statistical tests, including univariate and multivariate statistical tests. In the next chapter, we will explore feature selection methods based on machine learning, a more proactive approach that utilizes the predictive power of machine learning models for feature selection.
# 3. Feature Selection Methods Based on Machine Learning
In machine learning, feature selection plays a significant role as it not only reduces model complexity and avoids overfitting but also improves the predictive performance of models. This chapter will detail feature selection methods based on machine learning, including model-based and penalty-based feature selection.
## 3.1 Model-Based Feature Selection
Model-based feature selection methods rely on the inherent feature selection capabilities of algorithms. These algorithms can evaluate the importance of features while building the model. A primary advantage of this method is that it takes into account the correlations between features, thus identifying and retaining more useful feature combinations.
### 3.1.1 Decision Tree Methods
Decision trees are one of the commonly used machine learning methods, classifying data through a series of judgment rules. Decision tree models not only provide an intuitive explanation of the data but also automatically perform feature selection.
```python
from sklearn.datasets import load_iris
from sklearn.tree import DecisionTreeClassifier
from sklearn.model_selection import train_test_split
# Load Iris dataset
iris = load_iris()
X = iris.data
y = iris.target
# Split the dataset into training and testing sets
X_train, X_test, y_train, y_test = train_test_split(X, y, test_size=0.2, random_state=42)
# Build decision tree model
clf = DecisionTreeClassifier(random_state=42)
clf.fit(X_train, y_train)
# Print feature importance
importances = clf.feature_importances_
indices = np.argsort(importances)[::-1]
# Output feature importance
for f in range(X_train.shape[1]):
```
0
0
相关推荐
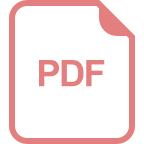
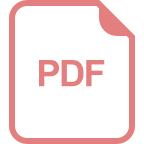





