Model Monitoring and Maintenance: 7 Key Steps to Ensure Long-Term Model Effectiveness
发布时间: 2024-09-15 11:43:14 阅读量: 28 订阅数: 31 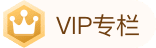
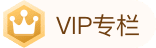
## The Importance of Model Selection and Validation in Machine Learning
Model monitoring and maintenance are key factors in ensuring the long-term, stable operation of machine learning models. With changes in business requirements, evolution in the data environment, and gradual aging of models, monitoring mechanisms can help us promptly detect a decline in model performance and take necessary maintenance measures. Moreover, monitoring and maintenance can effectively identify data drift and concept drift, which are major causes for a decline in model accuracy and reliability. Additionally, continuous monitoring and timely maintenance can enhance the transparency and interpretability of models, boosting stakeholders' confidence in the models, thus maintaining a competitive edge in fierce market competition.
In the following chapters, we will delve into the theoretical foundations of model monitoring, operational strategies for monitoring and maintenance, as well as the automation of monitoring processes, to ensure that models in the IT industry can adapt to environmental changes and maintain optimal performance.
## The Theoretical Foundations of Model Monitoring
### 2.1 Model Performance Evaluation Metrics
Model performance evaluation is the first step in model monitoring, with the key being how to accurately and objectively measure the predictive power of the model. Choosing the appropriate evaluation metrics can help us better understand the performance of the model and provide guidance for subsequent optimization work.
#### 2.1.1 Accuracy and Precision
Accuracy refers to the proportion of the model's correct predictions, which directly reflects the overall predictive effect of the model. However, in certain application scenarios, such as medical diagnosis, the tolerance for different types of prediction errors is not the same. In such cases, precision and recall become particularly important.
Precision measures the proportion of true positives among the samples predicted as positive by the model, which reflects the reliability of the model in positive predictions. Its formula is:
```math
Precision = \frac{True Positive}{True Positive + False Positive}
```
Where True Positive (the true positive class) is the number of samples correctly predicted as positive by the model, and False Positive (the false positive class) is the number of samples incorrectly predicted as positive by the model. Therefore, if the precision is high, it means that when the model says "yes," it is almost always correct.
#### 2.1.2 Recall and F1 Score
Recall, also known as true positive rate, focuses on the proportion of positive samples identified by the model out of all actual positive samples. Recall measures the inclusiveness of the model, with its formula being:
```math
Recall = \frac{True Positive}{True Positive + False Negative}
```
Where False Negative (the false negative class) represents the number of positive samples that the model incorrectly predicts as negative. If a model has a high recall, it means it rarely misses true positives.
The F1 score is the harmonic mean of precision and recall, serving as a comprehensive indicator for both, especially applicable when there is an extreme imbalance between positive and negative samples, and its formula is:
```math
F1 Score = 2 * \frac{Precision * Recall}{Precision + Recall}
```
The F1 score considers both the precision and the coverage of the model's predictions, providing a more balanced performance evaluation metric.
### 2.2 Identifying and Handling Model Drift
After deployment, as time goes on, the predictive capability of a model may gradually decrease due to changes in the external environment or alterations in data distribution, a phenomenon known as model drift. Model drift is an important issue that requires ongoing attention in model monitoring.
#### 2.2.1 Methods for Detecting Data Drift
Data drift refers to changes in the distribution of input features, which can lead to a decline in model performance. One common method for detecting data drift is to calculate statistical information for features, such as mean, variance, etc., and compare these with historical data. For example, Kullback-Leibler divergence (KL divergence) can be used to measure the difference between data probability distributions:
```python
from scipy.stats import entropy as kl_divergence
def compute_kl_divergence(P, Q):
"""Compute the KL divergence between two probability distributions P and Q"""
return kl_divergence(P, Q)
# Suppose P and Q represent the probability distributions of feature distributions in historical data and the latest collected data, respectively
P = [0.2, 0.3, 0.5]
Q = [0.1, 0.4, 0.5]
# Compute KL divergence
kl_div = compute_kl_divergence(P, Q)
print(f"The KL Divergence between P and Q is {kl_div}")
```
#### 2.2.2 Impact of Concept Drift
Concept drift refers to changes in the distribution of target variables within the data. Unlike data drift, concept drift can occur even when there is no significant change in the feature distribution. Concept drift may be caused by external environmental changes, changes in user behavior, and other factors, which directly affect the accuracy of model predictions.
Methods for identifying concept drift can be divided into unsupervised and supervised categories. Unsupervised methods can use distribution similarity measures, such as Earth Mover's Distance (EMD) or statistical distribution testing methods like Kolmogorov-Smirnov tests. Supervised methods detect concept drift by continuously tracking changes in the accuracy of model predictions and various indicators.
#### 2.2.3 Drift Response Strategies
Once model drift is identified, the next step is to adopt appropriate response strategies. Strategies can be categorized into two types: passive and active.
Passive strategies involve retraining or fine-tuning the model to adapt to the new data distribution. For example, using a sliding window of data to retrain the model, or only updating the model upon detecting drift. Active strategies focus on continuously adjusting the model to adapt to data changes. For example, implementing online learning or continuously integrating new data to continuously improve the model. Additionally, one can design more adaptable models, such as ensemble methods or robust feature selection.
### 2.3 Model Monitoring Tools and Platforms
The choice of monitoring tools and platforms greatly affects the efficiency and effectiveness of model monitoring. This section will introduce some commonly used monitoring tools and platforms and compare them.
#### 2.3.1 Introduction to Open-Source Monitoring Tools
Open-source monitoring tools are widely adopted due to their flexibility and cost-effectiveness. For example, Prometheus is an open-source monitoring solution that provides powerful data collection and querying capabilities, and manages alerts through Alertmanager. Although Prometheus is mainly used for system monitoring, its strong customization capabilities also make it suitable for model monitoring. By defining appropriate query statements, one can regularly check whether model performance metrics meet expectations.
Another popular open-source monitoring tool is the ELK Stack (Elasticsearch, Logstash, and Kibana), which is mainly used for collecting, analyzing, and visualizing log data. ELK can be used to monitor the real-time behavior of models, such as abnormal predictive behavior in log files.
#### 2.3.2 Comparison of Commercial Monitoring Platforms
Compared to open-source tools, commercial monitoring platforms typically offer more comprehensive services, user interfaces, and automation features. For example, DataDog is a comprehensive cloud monitoring platform that offers a full suite of monitoring, alerting, and data analysis tools. DataDog provides excellent support for data analysis and visualization, making monitoring the performance and stability of models more manageable.
Seldon Core is another open-source platform used for deploying and monitoring machine learning models. It seamlessly integrates with Kubernetes and offers real-time monitoring and logging features, making it an ideal choice for machine learning model operations.
#### 2.3.3 Automated Monitoring Processes
Automated monitoring processes are essential for improving the efficiency and accuracy of model monitoring. Automated monitoring processes include not only data collection and performance metric calculation but should also include real-time alerts and automatic model repair mechanisms. For instance, CI/CD pipelines can be used to automate the model update process, only deploying new models after they pass all performance tests.
Below is a simple example of an automated monitoring process written in Python:
```python
import requests
def monitor_model_performance(model_id):
"""Monitor the performance indicators of a specified model and automatically send alerts when issues are detected"""
# Assuming there is an API to obtain model performance metrics
performance_url = f'***{model_id}'
```
0
0
相关推荐
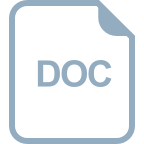
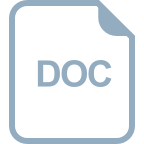
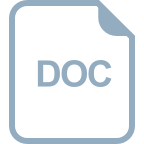
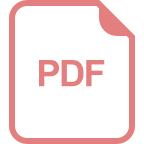
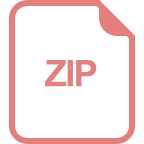
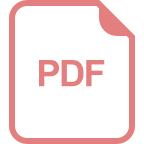
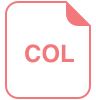
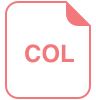
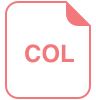