Why Explainability in Models Matters: 4 Methods to Achieve Interpretable AI
发布时间: 2024-09-15 11:36:35 阅读量: 27 订阅数: 26 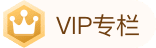
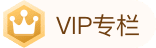
# The Importance of Model Interpretability: Achieving Explainable AI with 4 Methods
## 1. The Necessity of Model Interpretability
The importance of model interpretability has been a widely discussed topic in the construction and deployment of artificial intelligence systems in recent years. This is not only because algorithms and models have become more complex, but also because for many applications, transparency and interpretability are crucial for building user trust, ensuring fairness, and complying with regulations. Understanding the decision-making process of a model enables us to diagnose and improve the model more effectively, reduce biases, and promote interdisciplinary collaboration. This chapter will discuss the necessity of model interpretability in the AI field and lay the theoretical and practical groundwork for subsequent discussions.
Model interpretability is not just a technical issue; it also involves legal, ethical, and moral aspects. For example, in the financial services industry, model interpretability helps prevent unfair credit scoring; in the medical field, explainable AI can enhance doctors' trust in disease predictions and treatment plans. Therefore, exploring interpretability is not only an aspect of improving AI technology; it also involves how we better apply these technologies in society. With the development of explainable AI, we can look forward to smarter, more transparent, and more morally responsible AI systems.
## 2. Fundamental Theories of Model Interpretability
### 2.1 Interpretability Issues in Machine Learning
Interpretability in machine learning models refers to the degree to which a model's predictions or decision-making process can be understood and explained by humans. This is a complex and challenging issue in machine learning, especially deep learning, as these models are often considered "black boxes" because their internal mechanisms are opaque.
#### 2.1.1 The Relationship Between Interpretability and Complex Models
Deep learning models are often criticized for lacking interpretability due to their complexity. These models typically contain billions of parameters and learn data representations through multiple levels of abstraction. Despite this, the black-box nature of these models may be acceptable in some cases, such as image recognition tasks where models can accurately identify objects in an image without necessarily knowing how they do so. However, as models are applied in decision support, medical diagnosis, legal judgments, and other critical areas, understanding their internal logic becomes increasingly important.
In some cases, interpretability can be traded off with model performance. For example, simplified models may have an advantage in interpretability but may sacrifice some predictive accuracy. Therefore, finding a balance between model complexity and interpretability is a key issue faced by researchers and practitioners.
#### 2.1.2 The Application of Interpretability in Different Fields
In various fields, interpretability is not just a technical issue but also an important legal and ethical one. For example, in the financial services industry, regulatory agencies may require that the decision-making process of models must be interpretable so that errors can be traced and corrected when they occur. In the medical field, doctors and patients need to understand how models arrive at specific treatment recommendations to promote better decision-making and trust.
Furthermore, the interpretability of models can help researchers and engineers identify and correct biases in models, which is crucial for enhancing the fairness and transparency of the models. Through model interpretability, we can better understand how models handle data from different groups and ensure that models do not unintentionally amplify existing inequalities.
### 2.2 A Comparison Between Interpretable Models and Black-Box Models
Compared to black-box models, the key advantage of interpretable models is that they can provide insights into their predictions, which is crucial for promoting user trust and model transparency.
#### 2.2.1 The Limitations of Black-Box Models
Black-box models are difficult to interpret because their decision-making processes are not intuitive. For example, deep neural networks model data by learning complex nonlinear functions, but these functions are usually difficult to explain intuitively.
The limitations of black-box models are evident in several aspects. First, their prediction results often lack transparency, making it difficult to assess the reliability of their predictions, especially in high-risk decision-making situations. Second, black-box models may contain biases that are difficult to detect because their decisions are based on complex pattern recognition, which may be inconsistent with human intuition and social values. Additionally, when models make errors, it is challenging to identify the root cause and make corrections due to a lack of transparency.
#### 2.2.2 The Advantages of Interpretable Models
Interpretable models, or white-box models, such as decision trees or linear regression, provide a clearer prediction process. The decision-making processes of these models can be described through simple rules or weight coefficients, making it easier for users to understand the model's prediction results.
A significant advantage of interpretable models is that they provide insights into how data affects model decisions, which is crucial for debugging, improving, and verifying models. For example, in medical diagnosis, doctors may need to know how a disease prediction model arrives at its predictions based on various patient indicators so that they can trust and use the model to make decisions.
Moreover, interpretable models help ensure that models do not unintentionally amplify social biases or unfair phenomena. By examining the internal workings of a model, researchers can identify and adjust features or decision rules that may cause bias, thus improving the fairness and accuracy of the model.
In the next chapter, we will delve into four methods to achieve AI interpretability, including feature importance analysis, model visualization techniques, local vs. global interpretation, and model simplification and surrogate models, to deeply understand how to overcome the limitations of black-box models in practical applications and leverage the advantages of interpretable models.
# 3. Four Methods to Achieve AI Interpretability
## 3.1 Feature Importance Analysis
### 3.1.1 Feature Importance Evaluation Methods
Being able to identify which features significantly impact a model's predictions is crucial when building machine learning models. Feature importance evaluation methods typically include the following:
1. **Model-based methods**: These methods usually incorporate feature importance evaluation during the model training process. For example, in the Random Forest algorithm, the importance of features is determined by calculating the average value of feature split information gain.
2. **Permutation-based methods**: For instance, Permutation Feature Importance, which evaluates the importance of features by observing the decline in model performance when a feature value is permuted.
3. **Model explainer-based methods**: Using model explainers such as LIME and SHAP, which can provide local or global explanations for black-box models.
### 3.1.2 The Application of Feature Importance in Decision Making
Feature importance not only helps us understand the model's decision-making process but is also a tool to improve model performance. By removing features with low importance, we can simplify the model, avoid overfitting, and enhance generalization capabilities. In business decisions, feature importance can reveal the underlying drivers behind data, increasing the transparency of decisions.
#### Example Code Block
The following example, using Python, shows how to use the Random Forest model in the Scikit-learn library to evaluate feature importance:
```python
from sklearn.ensemble import RandomForestClassifier
import numpy as np
# Assuming we have a dataset X and labels y
# X, y = load_your_data()
rf = RandomForestClassifier()
rf.fit(X, y)
# Print feature importance
importances = rf.feature_importances_
indices = np.argsort(importances)[::-1]
for f in range(X.sha
```
0
0
相关推荐
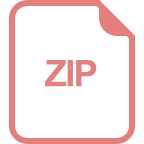
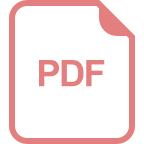
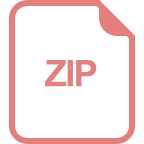
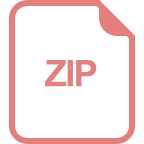
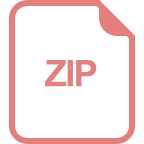
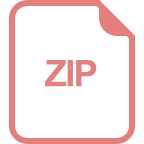
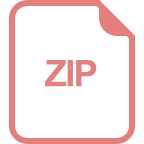
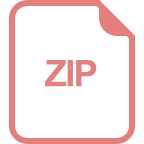
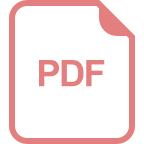