Comprehensive Analysis of Model Evaluation Metrics: How to Choose the Best Model and Optimize Performance
发布时间: 2024-09-15 11:17:24 阅读量: 39 订阅数: 31 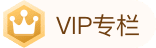
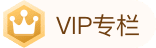
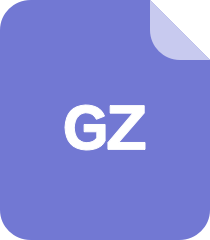
kube-state-metrics:v2.7.0
# 1. Introduction to Model Evaluation Metrics
In the realm of machine learning and data mining, model evaluation is a crucial step to ensure that the constructed models are effective, reliable, and widely applicable. Evaluation metrics not only measure the performance of models but also guide us in optimizing them. This chapter will explore the foundational concepts of evaluation metrics, their varied applications in classification and regression problems, and special considerations for multi-class classification issues, providing a theoretical basis for subsequent chapters.
## 1.1 The Role and Importance of Evaluation Metrics
Evaluation metrics help us answer the core question: "How well does the model perform?" The metrics we focus on differ depending on the type of problem. For instance, in classification problems, we may be interested in the accuracy of the model's predictions, while in regression problems, we may be more concerned with the size of the deviation between predicted and actual values. Correctly selecting and applying evaluation metrics can provide an objective basis for model selection, ensuring that the model performs well on unseen data.
## 1.2 Brief Introduction to Common Evaluation Metrics
Common model evaluation metrics include:
- Classification problems: Accuracy, Precision, Recall, F1 Score, ROC Curve, and AUC Value.
- Regression problems: Mean Squared Error (MSE), Root Mean Squared Error (RMSE), Coefficient of Determination (R²), and Mean Absolute Error (MAE).
- Multi-class classification: Confusion Matrix, Macro-average, Micro-average, and Cross-validation.
In the following chapters, we will delve into the definitions, calculation methods, and application scenarios of these metrics. With this knowledge, readers will better master the techniques of model evaluation and make wiser choices in practice.
# 2. Evaluation Metrics for Classification Problems
### 2.1 Accuracy and Precision
#### 2.1.1 Definition and Calculation of Accuracy
In classification tasks, accuracy is the most intuitive evaluation metric, representing the proportion of correctly classified samples to the total number of samples. The accuracy formula is as follows:
\[ \text{Accuracy} = \frac{\text{Number of correctly predicted samples}}{\text{Total number of samples}} \]
Although accuracy is easy to understand and calculate, it may not be the best evaluation metric in the case of imbalanced data. For example, if 99% of the samples in a dataset belong to one class, a simple model that always predicts that class can still achieve high accuracy, but it does not effectively learn the features of other classes.
The calculation of accuracy usually involves the confusion matrix, an important tool for evaluating classification quality. In the confusion matrix, each row represents the actual class, and each column represents the predicted class. Accuracy is the total number of correct predictions (i.e., the elements on the diagonal) divided by the total number of samples.
#### 2.1.2 Concept and Application Scenarios of Precision
Precision measures the proportion of samples predicted as the positive class that are actually positive. The precision formula is:
\[ \text{Precision} = \frac{\text{Number of correctly predicted positive samples}}{\text{Total number of predicted positive samples}} \]
Precision is applicable to situations where the correctness of predictions for the positive class is more important. For instance, in spam email classification, we prefer to reduce the number of legitimate emails incorrectly marked as spam to avoid losing information. In such cases, precision is an important evaluation metric.
Precision is often used in conjunction with other metrics (such as Recall) to provide a more comprehensive model evaluation. The relationship between accuracy, precision, and recall, and their importance in different applications, will be explored in depth in subsequent chapters.
### 2.2 Recall and F1 Score
#### 2.2.1 Meaning and Importance of Recall
Recall, also known as true positive rate, focuses on the proportion of all actual positive samples that the model correctly identifies. The recall formula is:
\[ \text{Recall} = \frac{\text{Number of correctly predicted positive samples}}{\text{Total number of actual positive samples}} \]
Recall is particularly important in scenarios where missing positive cases (false negatives) has a high cost. For example, in disease diagnosis, missing a real case could lead to severe consequences, so recall becomes a key performance indicator in the medical field.
Optimizing recall often involves sacrificing some precision because increasing recall means predicting more samples as positive, which could lead to more incorrect classifications. Therefore, in practical applications, it is necessary to balance precision and recall according to specific needs.
#### 2.2.2 Comprehensive Evaluation Ability of F1 Score
The F1 Score is the harmonic mean of precision and recall; it considers the relationship between the two and attempts to find a balance. The F1 Score formula is as follows:
\[ F1 = 2 \times \frac{\text{Precision} \times \text{Recall}}{\text{Precision} + \text{Recall}} \]
The maximum value of the F1 Score is 1, achieved when both precision and recall are 1, and the minimum value is 0. The F1 Score is particularly suitable for classification tasks where precision and recall are equally important. By calculating the F1 Score, one can avoid excessively reducing recall while improving precision, or vice versa.
### 2.3 ROC Curve and AUC Value
#### 2.3.1 Method of Drawing the ROC Curve
The ROC Curve (Receiver Operating Characteristic curve) is an effective tool for evaluating the performance of classification models. It plots the True Positive Rate (TPR, the same as Recall) against the False Positive Rate (FPR) on the same graph, thereby demonstrating the model's performance at different thresholds.
Steps to draw the ROC Curve include:
1. Calculate the model's predictive probabilities for positive and negative classes at different thresholds.
2. Set thresholds from high to low and calculate the corresponding TPR and FPR for each threshold.
3. On the ROC graph, plot the FPR on the x-axis and the TPR on the y-axis.
Generally, the closer the ROC Curve is to the top-left corner, the better the model's classification performance. This is because the top-left corner corresponds to high TPR and low FPR.
#### 2.3.2 Meaning and Evaluation Criteria of AUC Value
The AUC (Area Under Curve) value is the area under the ROC Curve, providing a single value to evaluate the performance of a classifier. The range of AUC values is from 0 to 1, where:
- An AUC value of 1 represents a perfect classifier, meaning the model has a higher predicted probability for all possible positive samples than for all possible negative samples.
- An AUC value of 0.5 represents a random classifier, meaning the model cannot distinguish between positive and negative samples.
Generally, an AUC value above 0.7 indicates that the model has good predictive performance; an AUC value between 0.5 and 0.7 indicates average predictive performance; and an AUC value below 0.5 suggests that the model's predictive performance is worse than random guessing. The AUC is a powerful tool for evaluating binary classification models, especially in situations where samples are imbalanced, providing more comprehensive information than accuracy.
Through accuracy, precision, recall, F1 Score, ROC Curve, and AUC value, we can evaluate classification models from multiple dimensions, gaining a more comprehensive understanding of model performance. The relationships between these metrics and how to choose the appropriate evaluation metric will be further explored in subsequent chapters.
# 3. Evaluation Metrics for Regression Problems
Regression problems are one of the common types in machine learning and data science, aimed at predicting continuous value outputs. In regression problems, evaluation metrics help us quantify the predictive performance of models. This chapter will delve into the main regression problem evaluation metrics, including Mean Squared Error (MSE), Root Mean Squared Error (RMSE), Coefficient of Determination (R²), and Mean Absolute Error (MAE), and gain a deeper understanding of how bias and variance concepts affect model performance.
## 3.1 Mean Squared Error (MSE) and Root Mean Squared Error (RMSE)
### 3.1.1 Calculation Formula for Mean Squared Error
Mean Squared Error (MSE) is one of the most commonly used performance metrics for regression problems. It calculates the average of the squared differences between predicted and actual values. The MSE formula is as follows:
```
MSE = (1/n) * Σ(y_i - ŷ_i)²
```
Where `y_i` is the actual value, `ŷ_i` is the predicted value, and `n` is the number of samples. A Python code example is as follows:
```python
import numpy as np
# Assuming actual and predicted values
actual = np.array([1, 2, 3, 4, 5])
predicted = np.array([1.1, 1.9, 3.1, 4.1, 5.1])
# Calculate MSE
mse = np.mean((actual - predicted) ** 2)
print(f"MSE: {mse}")
```
MSE penalizes larger errors more because it squares the errors, thus amplifying even very small errors. The better the model's performance, the smaller the value of MSE.
### 3.1.2 Characteristics and Advantages of Root Mean Squared Error
Root Mean Squared Error (RMSE) is the square root of MSE; it converts the error measure into the same unit as the target variable, making it easier to understand and interpret. The RMSE formula is:
```
RMSE = √(MSE)
```
The main advantage of using RMSE instead of MSE is its interpretability, as RMSE provides the actual range of errors. The code implementation for RMSE is as follows:
```python
import numpy as np
# Continuing with the actual and predicted variables from above
# Calculate RMSE
rmse = np.sqrt(np.mean((actual - predicted) ** 2))
pri
```
0
0
相关推荐
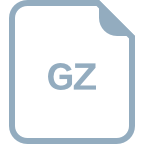
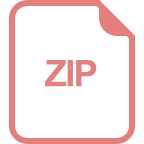
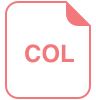
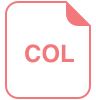
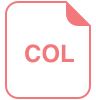
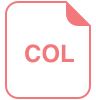
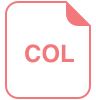
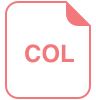