The Application of A/B Testing in Model Selection: 3 Key Steps to Success
发布时间: 2024-09-15 11:19:14 阅读量: 38 订阅数: 42 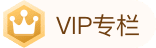
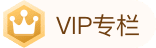
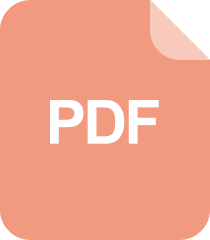
The Application of AHP+LP in the Evaluation and Selection of Suppliers
# A/B Testing in Machine Learning: Model Selection and Validation
## 1. The Basics of A/B Testing and Its Importance
### 1.1 Definition of A/B Testing
A/B testing, also known as split testing, is a method for comparing two versions (A and B) of a webpage or application to determine which performs better in terms of key performance indicators (KPIs) like conversion rate, click-through rate, or user engagement.
### 1.2 The Importance of A/B Testing
Data-driven decision-making has become a consensus in product development and marketing strategies. A/B testing is crucial as it provides empirical evidence, reduces subjective speculation, and enhances the objectivity and accuracy of decision-making. With A/B testing, companies can directly understand user preferences, continuously improve products and services, enhance the user experience, and ultimately achieve growth in revenue.
### 1.3 The Scope of A/B Testing in Business
A/B testing is not only applicable to website and mobile app design optimization but is also widely used in product feature iteration, marketing strategy optimization, and evaluating the effectiveness of advertising campaigns. By conducting scientific experiments on subtle changes, companies can ensure that every decision is based on actual user feedback, rather than intuition or assumptions.
## 2. The Theoretical Foundation of A/B Testing
### 2.1 Statistical Principles of A/B Testing
#### 2.1.1 Randomization and Experimental Design
One of the core principles of A/B testing is randomization, meaning users are randomly assigned to different test groups to ensure each has an equal chance of being placed in any test group. This randomization ensures the validity and fairness of experimental results, reducing biases such as selection bias, experimental bias, and temporal bias.
Randomization is a key step in experimental design. Proper implementation of randomization can minimize the impact of external variables on experimental outcomes. To achieve effective randomization, data randomization groups are required, which is typically achieved through generating random numbers.
**Example Code Block:**
```python
import pandas as pd
import numpy as np
# Assuming we have a user data frame
data = pd.DataFrame({
'user_id': np.arange(1, 101), # Generating user IDs
'user_data': np.random.randn(100) # Random user data
})
# Randomly dividing users into two groups, Group A and Group B
def assign_groups(df, size_of_group_A):
df['group'] = np.random.choice(['A', 'B'], size=df.shape[0], p=[size_of_group_A, 1 - size_of_group_A])
return df
data = assign_groups(data, 0.5)
print(data.head())
```
**Logical Analysis and Parameter Explanation:** The above code creates an example of randomly assigning users, where users are equally likely to be assigned to two groups, namely Group A and Group B. Here, the `assign_groups` function randomly assigns group labels "A" and "B" to users through the `random.choice` method, ensuring randomness. The `size_of_group_A` parameter allows controlling the size proportion of Group A in the test.
#### 2.1.2 Hypothesis Testing and Significance Levels
When conducting A/B testing, hypothesis testing is typically required to determine if there is a statistically significant difference between two options. A null hypothesis (H0) is usually set, assuming no significant difference between the two groups, and an alternative hypothesis (H1), assuming a significant difference.
To reject the null hypothesis, a significance level (α) is used, which is the maximum probability of type I errors (false positives) ***mon significance levels are 0.05 or 0.01.
**Logical Analysis and Parameter Explanation:** In A/B testing, t-tests or chi-square tests are commonly used to evaluate differences between groups. If the p-value is lower than the pre-set significance level, we reject the null hypothesis, considering the difference between the two groups to be statistically significant, rather than due to random error.
#### 2.1.3 Data Analysis and Effect Size
After obtaining test results, analyzing test data is crucial. Data analysis can help us determine if one option is more effective than another and whether this difference has practical significance. Calculating the effect size can quantify the difference between two options beyond statistical significance, providing information about the actual importance of the difference.
Effect size is typically represented by Cohen's d, Odds Ratio, or other standardized measures. The larger the effect size, the greater the actual difference between the two options, rather than just statistical significance.
**Logical Analysis and Parameter Explanation:** Calculating the effect size requires considering sample size, standard deviation, and mean values. In A/B testing, Cohen's d values can be calculated by dividing the difference in the means of two groups by the standard deviation. The size of the effect can be measured using standards such as small (0.2), medium (0.5), and large (0.8).
## 2.2 Definition of Variables in A/B Testing
### 2.2.1 Choosing Appropriate Test Variables
When conducting A/B testing, choosing the right test variables is crucial. Test variables are typically different versions of the features being tested, such as different layout designs of a webpage, different colors of buttons, or different content in advertising copy.
**Logical Analysis and Parameter Explanation:** When choosing test variables, it is essential to ensure that the choice of variables has a direct impact on business goals. For example, if the goal is to increase conversion rates, then the test variables might focus on the design of the purchase button. In choosing test variables, the three principles of variability, relevance, and measurability must be followed.
### 2.2.2 Setting Control Variables
Control variables are factors that remain unchanged in A/B testing to ensure that only changes to the test variables affect the results. Control variables play an important role in any experiment as they help isolate the effects, making differences between test groups attributable to changes in a single variable.
**Logical Analysis and Parameter Explanation:** For example, in an A/B test of website design, the test pages A and B should be consistent in all design elements except for button color. Therefore, any changes in conversion rates can be reasonably attributed to the change in button color.
### 2.2.3 Relationship Between Variables and User Behavior
In A/B testing, we usually expect to affect user behavior by changing certain variables. For example, by changing the layout of a webpage, we can alter the browsing path of users, which in turn affects their purchasing behavior.
**Logical Analysis and Parameter Explanation:** To accurately understand the relationship between variables and user behavior, it is generally necessary to collect user behavior data, such as click-through rates and page view times, which can be collected and analyzed during the test. This can help us understand which changes to variables positively impact user behavior.
## 2.3 Multivariate Testing Methods in A/B Testing
### 2.3.1 The Problem of Global and Local Optimality
In multivariate testing, an important issue that may arise is the conflict between global optimality and local optimality. Global optimality refers to finding the best solution within the entire system, while local optimality refers to finding the best solution within a single variable.
**Logical Analysis and Parameter Explanation:** For example, in website design, changing a specific button color may increase the click-through rate, but this color may not be consistent with the overall design style of the website, leading to a decrease in overall user experience. This is an example of the potential conflict between local and global optimality.
### 2.3.2 Strategies and Case Studies for Multivariate Testing
Multivariate testing, also known as full-factorial testing, is a method that tests multiple variables and their combinations simultaneously. This method helps understand the impact of different variable combinations on business goals, identifying which interactions between variables can lead to the most significant improvements.
**Logical Analysis and Parameter Explanation:** When conducting multivariate testing, a detailed test plan and strategy should be developed, such as using orthogonal arrays to ensure that the test design is both efficient and comprehensive. Case studies can help us understand how to handle and analyze the results of multivariate testing in practical operations.
### 2.3.3 Determining Experiment Duration and Sample Size
Determining the experiment duration and sample size is a critical part of A/B testing. A too-short duration may lead to unstable results, while a too-long duration may result in high costs. A too-small sample size may result in insufficient statistical power for testing, and a too-large sample size may require more resources.
**Logical Analysis and Parameter Explanation:** Determining experiment duration and sample size should be based on estimated changes, statistical power analysis, and available resources. For example, using power analysis can determine the minimum sample size needed to detect a specific effect size, ensuring the experimental results are stat
0
0
相关推荐







