MATLAB Genetic Algorithm Supply Chain Optimization: Three Key Steps in Practical Application
发布时间: 2024-09-15 04:28:19 阅读量: 62 订阅数: 40 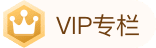
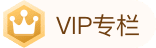
# 1. Introduction to Genetic Algorithms in MATLAB
As a widely-used mathematical computing and visualization software, MATLAB's powerful computational capabilities and rich toolbox functions make it an ideal platform for research and application of genetic algorithms. A genetic algorithm is a search and optimization algorithm that simulates the mechanisms of natural selection and genetics. It iteratively solves problems through selection, crossover, and mutation operations, making it suitable for solving complex optimization problems.
## 1.1 Origin and Development of Genetic Algorithms
The concept of genetic algorithms was first proposed by American computer scientist John Holland in the 1960s. Initially designed to simulate the mechanism of natural selection in the process of biological evolution, it treats potential solutions in the solution space as a "population" and simulates biological evolution through selection, crossover, and mutation operations to find better solutions over multiple generations. In recent years, with the development of computing technology, the application fields of genetic algorithms have been continuously expanding, extending from the initial function optimization problems to machine learning, data mining, artificial intelligence, and supply chain optimization, among other areas.
## 1.2 Overview of MATLAB Genetic Algorithm Toolbox
MATLAB provides a dedicated genetic algorithm toolbox (Global Optimization Toolbox), which includes a series of functions and programs to help users implement genetic algorithms. These tools not only simplify the implementation process of genetic algorithms but also allow users to adjust and customize parameters to address specific optimization problems. The toolbox supports various features, including custom fitness functions, constraint handling, multi-objective optimization, significantly enhancing the flexibility and effectiveness of genetic algorithms in practical applications.
In subsequent chapters, we will delve into the basics of supply chain optimization and how MATLAB plays a key role in the implementation and application of genetic algorithms. We will gradually uncover the mysteries of MATLAB genetic algorithms in an easy-to-understand manner and deepen our understanding through practical cases.
# 2. Fundamentals of Supply Chain Optimization
Supply chain optimization is crucial for enhancing the operational efficiency and effectiveness of the entire supply chain. Its goal is to reduce operational costs, shorten order cycles, increase responsiveness to market demands, and improve customer satisfaction. In this chapter, we will delve into the basic concepts, objectives, and significance of supply chain optimization, as well as the role of genetic algorithms in this process.
## 2.1 Concept of Supply Chain Optimization
### 2.1.1 Components and Processes of a Supply Chain
A supply chain is a complex network system consisting of raw material suppliers, manufacturing plants, warehouses, distribution centers, sales networks, and end customers. It encompasses the entire process from raw material procurement, product design, production manufacturing, inventory management, to the final delivery of products to consumers.
In modern supply chain management, process optimization is a dynamic and continuous process, including several key links:
1. Demand planning: Forecast market demand and develop corresponding production plans accordingly.
2. Procurement management: Select appropriate suppliers and ensure the quality and supply of raw materials and components.
3. Production planning and control: Optimize production processes, reduce waste, and ensure product quality.
4. Inventory management: Set inventory levels reasonably based on market demand forecasts.
5. Logistics distribution: Achieve a balance between cost and efficiency through effective logistics network planning.
6. Sales and distribution: Adjust sales strategies and optimize product distribution channels.
### 2.1.2 Objectives and Significance of Optimization
The ultimate goal of supply chain optimization is to enhance the efficiency and customer satisfaction of the entire supply chain. To achieve this goal, several core objectives usually need to be met:
1. Cost minimization: Reduce production, inventory, and transportation costs to achieve overall cost control of the supply chain.
2. Accelerated response speed: Optimize processes to reduce order processing time and increase responsiveness to market changes.
3. Improved customer service levels: Ensure customer needs are met, reduce stockouts and excess inventory situations.
4. Optimal resource utilization: Achieve efficient use of logistics, information flow, capital flow, and other supply chain resources.
The significance of supply chain optimization lies in:
1. Enhancing competitiveness: By optimizing the supply chain, companies can reduce costs, improve product and service quality, thereby enhancing their competitiveness in the market.
2. Risk management: Optimizing the supply chain helps companies better respond to market fluctuations, raw material price changes, natural disasters, and other risks.
3. Sustainable development: Supply chain optimization can also promote sustainable development for enterprises, reduce environmental impact, and meet corporate social responsibility requirements.
## 2.2 Role of Genetic Algorithms in Supply Chain Optimization
### 2.2.1 Basic Principles of Genetic Algorithms
Genetic algorithms (GA) are search heuristic algorithms that simulate natural selection and genetic mechanisms. Its basic principles draw on concepts such as selection, crossover (hybridization), and mutation from the theory of biological evolution, iteratively searching for optimal solutions.
In supply chain optimization, genetic algorithms can be used to solve complex combinatorial optimization problems, such as production scheduling, inventory optimization, and transportation and distribution. The algorithm starts with an initial population and generates a new generation through selection, crossover, and mutation operations, continuously iterating until an approximate optimal solution is found.
### 2.2.2 Comparison of Genetic Algorithms with Other Optimization Algorithms
Compared with other optimization algorithms, genetic algorithms have the following advantages:
1. Global search capability: Genetic algorithms do not rely on gradient information and can search for optimal solutions in the global search space.
2. Robustness: The algorithm is not sensitive to the initial conditions and parameter settings of the problem, making it easy to implement.
3. Simple coding method: Problems can be encoded as chromosomes, making genetic operations convenient.
4. Easy parallel processing: Calculations between individuals (chromosomes) are relatively independent, making them suitable for parallel processing.
However, genetic algorithms also have some limitations:
1. Slow convergence speed: Especially when facing large-scale problems.
2. Parameter adjustment: The performance of the algorithm largely depends on the parameter settings.
3. Requires a large number of iterations: It may be necessary to run thousands or even millions of iterations to obtain satisfactory solutions.
## 2.3 Application of MATLAB in Genetic Algorithms
### 2.3.1 Basic Introduction to MATLAB
MATLAB (Matrix Laboratory) is a high-performance numerical computing environment and fourth-generation programming language. It is widely used in engineering calculations, data analysis, algorithm development, and other fields.
The characteristics of MATLAB include:
1. Powerful matrix computation capabilities: As its core, MATLAB provides a rich set of matrix operation functions.
2. Efficient algorithm implementation: It includes a large number of mathematical, statistical, and engineering functions, supporting fast algorithm prototyping development.
3. User-friendly interface: Provides graphical user interface (GUI) development tools for interactive design.
4. A rich set of toolboxes: Provides specialized toolboxes for specific fields such as signal processing, image processing, genetic algorithms, etc.
### 2.3.2 Use of the MATLAB Genetic Algorithm Toolbox
The MATLAB genetic algorithm toolbox (GA Toolbox) provides standard functions and abundant parameter setting options for implementing genetic algorithms, greatly facilitating the implementation of genetic algorithms. The main functions of the toolbox include:
1. Parameter setting: Such as population size, crossover probability, mutation probability, etc.
2. Evolutionary algorithm functions: Used to execute the optimization process of genetic algorithms.
3. Visualization tools: Provide various data visualizations during the algorithm run, such as fitness curves, convergence curves, etc.
The basic steps for using the MATLAB genetic algorithm toolbox are as follows:
1. Define the fitness function: Transform the problem to be optimized into a fitness evaluation function.
2. Configure genetic algorithm parameters: Adjust the population size, crossover and mutation strategies, etc., according to the problem's characteristics.
3. Execute the optimization process: Start the genetic algorithm by calling functions like `ga`.
4. Analyze the results: Use the data and charts provided by the toolbox to analyze the optimization results.
The application of genetic algorithms in MATLAB is not limited to supply chain optimization; it is also widely used in engineering optimization, design automation, artificial intelligence, and other fields. With the MATLAB toolbox, even engineers and researchers who are not experts in genetic algorithms can effectively utilize this powerful optimization strategy.
# 3. Implementation Steps of MATLAB Genetic Algorithms
## 3.1 Encoding and Initial Population Generation
### 3.1.1 Choosing an Appropriate Encoding Strategy
In genetic algorithms, the encoding strategy is the process of representing pr***mon encoding methods include binary encoding, real-number encoding, permutation encoding, etc. Binary encoding is simple and intuitive but may require more complex crossover and mutation operations. Real-number encoding is suitable for optimization problems in the real-number domain, reducing the complexity of encoding and decoding. Permutation encoding is suitable for solving sequence arrangement problems, such as the Traveling Salesman Problem (TSP).
In the context of supply chain optimization, if the problem is about path selection or scheduling, permutation encoding may be a good choice. If it is a resource allocation problem, real-number encoding may be more appropriate. When choosing an encoding strategy, one should consider the characteristics of the problem and the efficiency of the algorithm.
### 3.1.2 Methods and Techniques for Initializing the Population
Initializing the population is the first step in genetic algorithms, involving the random generati
0
0
相关推荐
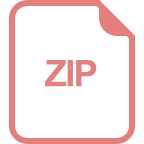
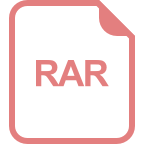
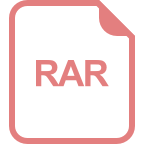
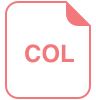
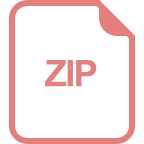
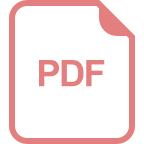
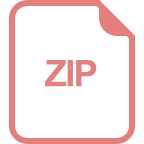
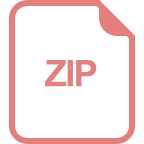
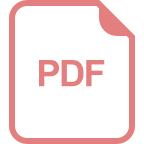