Application of MATLAB Genetic Algorithms in Bioinformatics: Frontier Research and Case Studies
发布时间: 2024-09-15 04:14:42 阅读量: 34 订阅数: 40 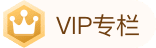
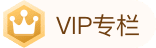
# 1. The Intersection of Genetic Algorithms and Bioinformatics
In the vast ocean of modern science, the intersection of genetic algorithms and bioinformatics is a vibrant confluence. Inspired by biological evolution theories, genetic algorithms mimic the natural processes of genetics and natural selection to solve complex problems. In the field of bioinformatics, the emergence of big biological data and the deep demand for analysis of biological systems provide a broad stage for the application of genetic algorithms. This chapter will briefly analyze the mutually beneficial relationship between genetic algorithms and bioinformatics and look forward to their potential integration paths in future technological development. By exploring the application of genetic algorithms in bioinformatics, we reveal how to use this intelligent optimization technology to analyze complex biological data and its profound impact on related fields.
The applications of genetic algorithms in bioinformatics mainly include, but are not limited to, gene sequence analysis, protein structure prediction, and metabolic network reconstruction in systems biology. They efficiently handle massive amounts of data in bioinformatics, providing a quick means of analysis and prediction. The application of these algorithms not only improves the efficiency of problem-solving but also provides new perspectives and tools for biomedical research. The subsequent sections of this chapter will delve into the theoretical foundations of genetic algorithms and how they are combined with specific applications in bioinformatics.
# 2. Theoretical Foundations and Mathematical Models of Genetic Algorithms
Before exploring the intersection of genetic algorithms (Genetic Algorithms, GA) and bioinformatics, it is essential to deeply understand the theoretical foundations and mathematical models of genetic algorithms. This chapter will interpret the core principles of genetic algorithms in detail and demonstrate their potential applications in bioinformatics.
## 2.1 Basic Principles of Genetic Algorithms
### 2.1.1 Evolutionary Computation and Natural Selection
Genetic algorithms draw on Charles Darwin's theory of natural selection and evolution. In nature, organisms evolve through the processes of genetics and natural selection, allowing the survival of the fittest and the elimination of the unfit. Genetic algorithms simulate this process by encoding potential solutions to problems as "chromosomes" and iteratively improving the quality of solutions through genetic operations such as selection, crossover (also known as hybridization or recombination), and mutation.
An example of code demonstrating how to use genetic algorithms in MATLAB:
```matlab
% Example MATLAB code showing how to initialize and run a genetic algorithm
% Define the fitness function
fitnessFcn = @myFitnessFunction; % Assume myFitnessFunction is a predefined fitness function
% Genetic algorithm options
options = optimoptions('ga', 'PopulationSize', 100, 'MaxGenerations', 100, 'Display', 'iter');
% Run the genetic algorithm
[x, fval] = ga(fitnessFcn, nvars, [], [], [], [], lb, ub, nonlcon, options);
```
Explanation: This code defines a fitness function `myFitnessFunction`, sets parameters for the genetic algorithm, and runs the algorithm to find the optimal solution. The operation of the genetic algorithm relies on the initial setup of the population, with a population size of 100 and a maximum of 100 generations. The `ga` function is a general function provided by the MATLAB Genetic Algorithm Toolbox for various optimization problems.
### 2.1.2 Key Operations and Steps of Genetic Algorithms
The key operations of genetic algorithms include selection, crossover, and mutation. The selection operation mimics the principle of "survival of the fittest" in nature, where superior chromosomes are selected and have the chance to reproduce. The crossover operation simulates the genetic process in organisms by exchanging parts of the parent chromosomes to produce new offspring. The mutation operation changes some genes in individuals randomly to increase the genetic diversity of the population.
A table comparing different genetic algorithm operations:
| Operation | Functional Description | Implementation Method |
|------------|-----------------------------------------------------------|---------------------------------------------|
| Selection (Selection) | Selection based on individual fitness, with higher fitness individuals having a greater chance of being inherited to the next generation | Roulette wheel selection, tournament selection, elitist selection, etc. |
| Crossover (Crossover) | Combines parent chromosomes to produce offspring with genetic diversity | Single-point crossover, multi-point crossover, uniform crossover, arithmetic crossover, etc. |
| Mutation (Mutation) | Changes certain genes in individuals with a certain probability to prevent premature convergence of the algorithm | Gene flip, uniform mutation, Gaussian mutation, etc.|
Explanation: The table lists the functional descriptions and implementation methods of the three primary operations in genetic algorithms. Selection operations use different strategies to simulate natural selection. Crossover operations use different methods to simulate chromosome recombination. Mutation operations use various mutation techniques to maintain the genetic diversity of the population.
## 2.2 Mathematical Models of Genetic Algorithms
### 2.2.1 Chromosome Encoding and Gene Representation
In genetic algorithms, chromosome encoding refers to how potential solutions to a problem are represented in a form that the genetic algorithm can manipulate. Gene representation refers to the form of individual genes within a chromosome, such as binary encoding, real-number encoding, symbolic encoding, etc.
Explanation: Chromosome encoding is the first step in simulating biological genetic behavior in genetic algorithms. Choosing the appropriate encoding method is crucial for effectively solving problems. For example, when solving optimization problems, real-number encoding may provide faster convergence speeds and more refined search capabilities in solution space than binary encoding.
### 2.2.2 Construction of the Fitness Function
The fitness function measures the quality of chromosomes (potential solutions). It defines the criteria for the selection operation, meaning that the higher the individual's fitness, the greater the chance of being selected to reproduce.
An example of code demonstrating the construction of a fitness function:
```matlab
function f = myFitnessFunction(x)
% x is the potential solution to the problem
f = -sum(x.^2); % Example fitness function using a simple quadratic equation
end
```
Explanation: The above example code defines a simple fitness function `myFitnessFunction`, which calculates the negative sum of squares of the input vector `x`. The design of the fitness function should be customized according to the actual problem's requirements, with a lower fitness value indicating a better solution.
### 2.2.3 Selection, Crossover, and Mutation Mechanisms
The selection mechanism determines how individuals are selected to participate in the reproduction of the next generation. The crossover and mutation mechanisms are responsible for creating genetic diversity within the population and guiding the population towards directions that are more adaptive to the environment.
A code block demonstrating the implementation of selection, crossover, and mutation in MATLAB:
```matlab
% Example MATLAB code for the selection mechanism using roulette wheel selection
parents = selectionFunction(population, fitness);
% Example MATLAB code for crossover operation
offspring = crossoverFunction(parents);
% Example MATLAB code for mutation operation
offspring = mutationFunction(offspring);
```
Explanation: The above code snippets demonstrate how to implement selection, crossover, and mutation mechanisms in MATLAB. `selectionFunction`, `crossoverFunction`, and `mutationFunction` represent the selection, crossover, and mutation functions, respectively, and are predefined functions that may need to be customized depending on the specific implementation of the algorithm.
## 2.3 Optimization Strategies of Genetic Algorithms
### 2.3.1 Methods for Maintaining Population Diversity
Population diversity is an important factor in preventing premature convergence of genetic algorithms. If there is insufficient diversity within the population, the algorithm may become stuck in local optima and fail to continue searching for the global optimum.
Explanation: When designing genetic algorithms, various strategies can be introduced to maintain population diversity, such as introducing foreign genes, increasi
0
0
相关推荐
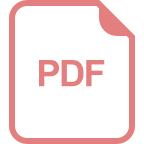
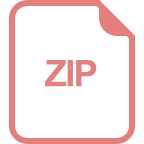
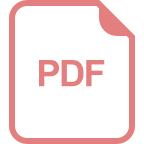
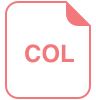
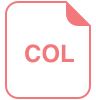
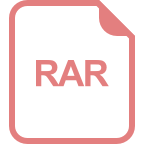
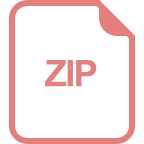
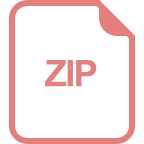
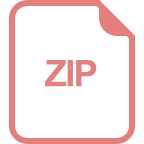