MATLAB Genetic Algorithm Function Optimization: Four Efficient Implementation Methods
发布时间: 2024-09-15 04:31:42 阅读量: 127 订阅数: 45 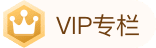
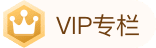
# Genetic Algorithm Function Optimization in MATLAB: Four Efficient Methods
## 1. Fundamental Theory of Genetic Algorithms
Genetic algorithms are optimization algorithms that simulate natural selection and genetics. They excel at solving optimization and search problems by effectively locating high-quality solutions in a broad search space. The essence of the algorithm is the use of probabilistic evolutionary processes to guide the search toward potential optimal regions. Based on the principle of "survival of the fittest," each solution is considered an individual. Through operations such as selection, crossover, and mutation, individual fitness is evaluated, and over generations of iteration, the population gradually evolves into an increasingly optimal state.
## 2.1 Basic Components of Genetic Algorithms
Genetic algorithms consist of the following basic components:
### 2.1.1 Initializing the Population
The initialization process of genetic algorithms involves generating an initial population, a series of randomly created candidate solutions. Each individual in the population is referred to as a chromosome, typically represented by binary strings, real numbers, or other encoding methods. The quality of the initial population is crucial to the performance of the algorithm.
### 2.1.2 Selection Mechanism
The selection mechanism is a process based on individual fitness for "reproduction." ***mon selection algorithms include roulette wheel selection and tournament selection.
### 2.1.3 Crossover and Mutation Strategies
Crossover (hybridization) is an important step in genetic algorithms that simulates biological genetics. It involves selecting two parent individuals and exchanging some of their genes to produce new offspring. The mutation strategy introduces randomness to avoid premature convergence to local optima by randomly changing certain genes within individuals.
The theoretical foundations of genetic algorithms provide us with powerful tools for exploring complex optimization problems. In the next chapter, we will delve into the specific implementation framework of genetic algorithms in MATLAB, provide code examples, and offer suggestions for parameter configurations.
# 2. Implementation Framework of Genetic Algorithms in MATLAB
As search algorithms that simulate natural selection and genetics, genetic algorithms are a potent tool for optimization problems. On the powerful MATLAB mathematical computing platform, implementing genetic algorithms not only has the support of ready-made toolboxes but also allows users to customize many details to meet the needs of various optimization problems. This chapter will详细介绍 the implementation framework of genetic algorithms in MATLAB, from basic components to function applications, to the design of fitness functions, gradually delving deeper to help advanced practitioners in the IT field better understand and apply this algorithm.
### 2.1 Basic Components of Genetic Algorithms
#### 2.1.1 Initializing the Population
The first step in genetic algorithms is to initialize a population, a set of potential solutions. In MATLAB, populations can be initialized through random generation. These solutions are usually encoded as a string of numbers representing points in the possible solution space.
```matlab
% MATLAB code example: Initializing the population
popSize = 100; % population size
chromLength = 30; % chromosome length
pop = randi([0, 1], popSize, chromLength); % generates a popSize x chromLength matrix
```
In the above MATLAB code, the `randi` function generates a matrix `pop`, where each row represents an individual, and each individual consists of `chromLength` genes. The range of gene values is 0 to 1, which is suitable for binary encoding.
#### 2.1.2 Selection Mechanism
The purpose of the selection mechanism is to decide which individuals will be preserved for the next generation. Methods such as roulette wheel selection and tournament selection can be used in MATLAB. Roulette wheel selection determines the probability of an individual being selected based on the size of its fitness.
```matlab
% MATLAB code example: Roulette wheel selection
fitness = sum(pop, 2); % calculates the fitness of each individual in the population
selected = rouletteWheelSelection(fitness); % selection operation
```
In actual implementation, `rouletteWheelSelection` is a custom function that assigns different selection probabilities based on individual fitness and simulates a lottery to select the next generation of individuals.
#### 2.1.3 Crossover and Mutation Strategies
Crossover and mutation are the two main methods for generating new individuals in genetic algorithms. Crossover refers to the exchange of gene segments between two individuals based on a certain method, while mutation randomly changes certain genes within an individual.
```matlab
% MATLAB code example: Crossover and mutation
children = crossover(pop); % crossover operation
mutatedChildren = mutate(children); % mutation operation
```
In the above code segment, the `crossover` and `mutate` functions simulate the crossover and mutation processes, operating on the individuals in the current population to generate the next generation.
### 2.2 Genetic Algorithm Functions in MATLAB
#### 2.2.1 Function Overview
The MATLAB genetic algorithm toolbox provides some predefined functions for solving optimization problems. One of the most important functions is the `ga` function, which can be used to solve unconstrained or constrained nonlinear optimization problems.
```matlab
% MATLAB code example: Using the ga function to solve an optimization problem
[x, fval] = ga(fun, nvars, A, b, Aeq, beq, lb, ub, nonlcon, options);
```
The parameters are as follows:
- `fun`: Objective function.
- `nvars`: Number of variables in the objective function.
- `A`, `b`, `Aeq`, `beq`: Linear constraints.
- `lb`, `ub`: Upper and lower bounds of variables.
- `nonlcon`: Nonlinear constraints.
- `options`: Structure for setting algorithm parameters.
- `x`: Returns the optimal solution.
- `fval`: Returns the value of the objective function at the optimal solution.
#### 2.2.2 Parameter Parsing and Configuration
In MATLAB, the `optimoptions` function can be used to configure various parameters of the `ga` function. For example, population size, crossover probability, mutation probability, etc., can be set.
```matlab
% MATLAB code example: Configuring ga function parameters
options = optimoptions('ga', 'PopulationSize', 150, 'CrossoverFraction', 0.8, 'MutationRate', 0.01, 'Display', 'iter');
```
The parameters configured in the above code will affect the search ability and convergence speed of the genetic algorithm. Careful adjustment of these parameters is key to optimizing the performance of genetic algorithms.
### 2.3 Design of the Fitness Function for Genetic Algorithms
#### 2.3.1 Role of the Fitness Function
The fitness function is the standard for evaluating the quality of individuals, determining the likelihood of individuals being selected for reproduction. In MATLAB, the fitness function should be defined as a function that takes a chromosome as input and returns a fitness value as output.
```matlab
% MATLAB code example: Defining a fitness function
function f = fitnessFunction(chromosome)
% Decoding the chromosome to obtain problem parameters
decodedParams = decodeChromosome(chromosome);
% Calculating the objective function value
f = objectiveFunction(decodedParams);
end
```
The design of the fitness function needs to be closely related to the actual problem and often utilizes prior knowledge of the problem domain to optimize.
#### 2.3.2 Key Points for Designing an Efficient Fitness Function
When designing a fitness function, the following points should be noted:
- The function should accurately reflect the quality of individuals.
- It should be as simple as possible to reduce computation time.
- If possible, avoid a fitness value of zero, which may cause the algorithm to stop evolving.
```matlab
% MATLAB code example: A fitness function avoiding zero fitness
function f = safeFitnessFunction(chromosome)
% Smoothing the objective function value
rawFitness = fitnessFunction(chromosome);
f = max(rawFitness, eps); % eps is a very small value in MATLAB
end
```
With the above method, all individuals have a certain chance to survive, allowing the genetic algorithm to explore more robustly.
In this section, by introducing the framework of MATLAB genetic algorithms, readers are provided with a complete process from population initialization, selection, crossover, mutation operations to the use of genetic algorithm functions, as well as the design of fitness functions. These contents provide a theoretical basis and practical methods for using MATLAB to solve optimization problems and lay a solid foundation for deeper exploration and application of genetic algorithms in subsequent chapters.
# 3. Advanced Techniques of Genetic Algorithms in MATLAB
## 3.1 Coding Strategies for Optimization Problems
### 3.1.1 Binary Coding and Real Number Coding
In genetic algorithms, coding strategies represent problem solutions in a chromosome structure, allowing genetic operations (selection, crossover, and mutation) t***mon coding strategies include binary coding and real number coding.
Binary coding is the most classic coding method, converting each decision variable into a string of binary bits, representing various possible decision variable values through combinations of 0 and 1. For example, a real number variable between 0 and 1 can be converted into a binary sequence, which is then used for crossover and mutation operations. Binary coding is simple and easy to implement, but when dealing with continuous variables, it may face discretization errors and excessively long coding lengths.
Real number coding directly represents decision variables in their decimal form, rather than converting them into binary sequences. In real number coding, crossover and mutation operations are performed on decimal values, which can avoid the precision loss caused by binary coding and is suitable for optimization problems involving continuous variables. Real number coding algorithms have straightforward crossover and mutation operations, high computational efficiency, and are easy to parallelize.
### 3.1.2 Innovative Application Examples of Coding
Taking the path planning problem as an example, traditional coding methods may not effectively represent complex path information, making innovative coding methods particularly important. For instance, graph theory can be used to represent paths with node sequences, where each chromosome represents a path sequence, and the genes represent specific nodes. This node sequence coding method can directly reflect the continuity of the path and facilitates the application of crossover and mutation operations.
In addition, coding methods designed for specific problems can greatly improve algorithm efficien
0
0