MATLAB Genetic Algorithm Parallel Computing: The Secret Weapon to Unlock Computational Potential and Enhance Performance
发布时间: 2024-09-15 04:07:11 阅读量: 42 订阅数: 21 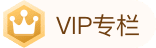
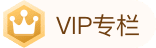
# 1. Genetic Algorithms and MATLAB Overview
In this chapter, we provide a brief introduction to Genetic Algorithms (GA) and explore its applications within the MATLAB environment. We start by introducing the fundamental concepts of genetic algorithms, including its origins, definition, and background as a heuristic search algorithm. We then highlight MATLAB, a powerful mathematical computing and simulation platform, which provides convenient tools for the implementation of genetic algorithms, laying the groundwork for in-depth exploration in subsequent chapters.
Genetic Algorithms are search and optimization algorithms inspired by Darwin's theory of evolution, which simulate natural selection and genetic principles to find optimal solutions in a given solution space. The core idea is to continuously evolve the fitness of individuals in a population to achieve problem-solving goals. MATLAB, as a common tool for scientific computing, offers the Genetic Algorithm Toolbox, providing developers with a rich set of functions and algorithmic frameworks, making the implementation and testing of genetic algorithms in MATLAB more convenient.
In the next chapter, we will delve into the basic principles of genetic algorithms and discuss in detail how to implement these algorithms in MATLAB.
# 2. Fundamental Principles and Implementation of Genetic Algorithms
## 2.1 Theoretical Basis of Genetic Algorithms
### 2.1.1 Origins and Definition of Genetic Algorithms
Genetic Algorithms (Genetic Algorithms, GAs) were developed by American scholar John Holland and his colleagues and students in the early 1970s. This class of search algorithms simulates natural selection and genetic mechanisms to solve complex optimization and search problems.
The fundamental idea of genetic algorithms is to encode potential solutions to problems as strings (often called chromosomes), and then perform operations such as selection, crossover (hybridization), and mutation on these strings within a collection (population) to iteratively produce new generations of solutions that are better adapted to the environment. After multiple generations of iteration, the algorithm tends to produce solutions of high performance, achieving the goals of optimization problems.
The definition of genetic algorithms includes several core components:
1. **Encoding**: Representing potential solutions to problems as chromosomes, usually using binary strings, real number strings, or other data structures.
2. **Initial Population**: Randomly generate an initial set of solutions.
3. **Fitness Function**: A criterion for evaluating the quality of chromosomes.
4. **Selection**: Selecting superior chromosomes based on the fitness function.
5. **Crossover**: Simulating biological genetic processes to produce offspring.
6. **Mutation**: Randomly changing parts of the chromosome to introduce new genetic information.
7. **New Generation Population**: Replacing the old population with chromosomes after selection, crossover, and mutation.
### 2.1.2 Main Operations of Genetic Algorithms: Selection, Crossover, Mutation
The three basic operations of genetic algorithms (selection, crossover, and mutation) are at the core of its algorithmic flow, and we will discuss each of them in detail below:
#### Selection Operation
The purpose of the selection operation is to select individuals with excellent qualities from the current population and give them the opportunity to enter the next generation. The selection process simulates the "survival of the fittest" principle of natural selection, ***mon selection methods include roulette wheel selection, tournament selection, and rank selection.
In roulette wheel selection, the probability of each individual being selected is proportional to its fitness. Assuming the population size is N, and the fitness of individual i is f(i), the probability P(i) that individual i is selected can be represented as:
\[ P(i) = \frac{f(i)}{\sum_{j=1}^{N}{f(j)}} \]
In this way, individuals with higher fitness have a higher chance of being selected, but individuals with lower fitness also have the possibility of being selected, maintaining the diversity of the population.
#### Crossover Operation
The crossover operation is the primary method of generating new individuals in genetic algorithms, simulating the hybridization process in biological genetics. The crossover process involves exchanging parts of the chromosomes of two (or more) parent individuals in a certain way to produce offspring individuals containing the genetic information of the parents.
In binary encoding, common crossover methods include single-point crossover, multi-point crossover, and uniform crossover. In single-point crossover, a crossover point is randomly determined, and parent individuals exchange parts of their chromosomes at this point to generate offspring. For example, if the chromosomes of parent individuals A and B are:
\[ A = 10110 \]
\[ B = 01001 \]
Setting the crossover point at the third position, the crossover operation produces the following offspring:
\[ A' = 10001 \]
\[ B' = 01110 \]
The key to the crossover operation is to find the appropriate crossover point and strategy to ensure that new effective solutions can be generated and useful genetic information can be preserved.
#### Mutation Operation
The mutation operation is the process of randomly changing one or more gene values in the chromosome. Its purpose is to introduce new genetic information in the search process of genetic algorithms, increase the diversity of the population, and avoid the algorithm converging prematurely to local optimal solutions. Mutation usually occurs with a smaller probability, ensuring the algorithm's exploration ability.
In binary encoding, the mutation operation can simply change a gene from 0 to 1 or from 1 to 0. For example, an individual whose gene is 0 before mutation becomes:
\[ \text{Before mutation} \quad 01001 \]
\[ \text{After mutation} \quad 01101 \]
In real number encoding, mutation may be a random perturbation, which is a small random number added to the current gene value. The mutation probability is usually set low to ensure the stability and convergence of the algorithm.
These are the three basic operations of genetic algorithms, which together constitute the core of the genetic algorithm framework. Through the iterative execution of these operations, genetic algorithms can efficiently search for optimal solutions in the solution space.
## 2.2 Programming Foundations of Genetic Algorithms in the MATLAB Environment
### 2.2.1 Overview of the MATLAB Genetic Algorithm Toolbox
MATLAB is a high-performance numerical computing and visualization software package released by MathWorks, widely used in engineering calculations, data analysis, algorithm development, and other fields. MATLAB has powerful matrix computation capabilities and provides a variety of toolboxes (Toolbox), among which the Genetic Algorithm Toolbox (GA Toolbox) facilitates the implementation and application of genetic algorithms.
The MATLAB Genetic Algorithm Toolbox mainly provides the following functions:
- **Problem Modeling and Encoding**: Supports direct encoding of target functions and implements the definition of fitness functions.
- **Parameter Control**: Provides a rich set of genetic algorithm parameter settings, allowing users to adjust algorithm parameters according to the characteristics and needs of the problem.
- **Genetic Operation Implementation**: Includes built-in implementations of selection, crossover, and mutation genetic operations, and provides interfaces for custom operations.
- **Population Management**: The toolbox manages operations such as population initialization, fitness calculation, individual selection, and the generation of new populations.
- **Result Output and Visualization**: After the algorithm runs, it can output results and provide visualization of the running process to help users analyze the performance of the algorithm.
The MATLAB Genetic Algorithm Toolbox is very easy to use; simply define the target function and corresponding parameters, and you can run the genetic algorithm for optimization. Below we will use a simple example to demonstrate how to use the MATLAB Genetic Algorithm Toolbox to write a genetic algorithm program.
### 2.2.2 Writing a Simple Genetic Algorithm Program
To demonstrate how to use the MATLAB Genetic Algorithm Toolbox, we will take a simple optimization problem as an example: finding the maximum value of the function f(x) = x^2 in the interval [-10, 10].
Here are the basic steps to write the genetic algorithm program for this problem using the MATLAB Genetic Algorithm Toolbox:
#### Step 1: Define the Target Function
First, you need to define the target function of the optimization problem, which is to find the maximum value of f(x):
```matlab
function y = myObjFunction(x)
y = -(x.^2); % Note that we are looking for the maximum value, but MATLAB defaults to finding the minimum, so we use a negative sign
end
```
#### Step 2: Set Genetic Algorithm Parameters
Next, set the parameters for running the genetic algorithm. These parameters include population size, crossover rate, mutation rate, and the number of iterations. Here we use MATLAB's `optimoptions` function to set these parameters:
```matlab
% Set genetic algorithm parameters
options = optimoptions('ga', ...
'PopulationSize', 100, ... % Population size
'MaxGenerations', 100, ... % Maximum number of iterations
'CrossoverFraction', 0.8, ... % Crossover rate
'MutationRate', 0.01, ... % Mutation rate
'Display', 'iter'); % Display information for each generation
```
#### Step 3: Call the Genetic Algorithm Function
Finally, call the genetic algorithm function `ga` to run the algorithm:
```matlab
% Run the genetic algorithm
[x, fval] = ga(@myObjFunction, 1, [], [], [], [], -10, 10, [], options);
```
Here, `@myObjFunction` is the handle to the target function, `1` indicates that the target function has 1 variable, `[-10, 10]` indicates the search range of the variable, and `options` is the parameter setting defined earlier.
After executing the above code, MATLAB will run the genetic algorithm and output the final solution found (the value of variable x) and the corresponding target function value (fval). In addition, information for each generation will be displayed in the console, including the best solution and average solution of each generation.
This simple example demonstrates how to use MATLAB's genetic algorithm toolbox to solve optimization problems. By modifying the target function and parameter settings, this toolbox can be applied to various complex optimization problems.
## 2.3 Parameter Tuning and Performance Evaluation of Genetic Algorithms
### 2.3.1 Impact of Parameter Settings on Algorithm Performance
There are several parameters in genetic algorithms that significantly affect their performance, including population size, crossover rate, mutation rate, and selection strategy. In this subsection, we will explore the impact of these parameters on the performance of genetic algorithms and how to effectively adjust parameters.
#### Population Size
Population size determines the breadth of the genetic algorithm's search space. A larger population can increase the diversity and coverage of the search space, thereby increasing the probability of finding the global optimum. However, a larger population will also lead to increased computational costs because the fitness of more individuals needs to be calculated in each generation. Therefore, a balance needs to be found between exploration and exploitation.
#### Crossover Rate
Crossover rate determines the degree of information exchange between individuals in the population. If the crossover rate is too high, it may destroy the better solutions currently present in the population; while a crossover rate that is too low may cause the search to陷入 local optimum, lacking diversity. Therefore, a reasonable crossover rate can effectively balance exploration and exploitation in the algorithm.
#### Mutation Rate
Mutation rate determines the probability of genetic changes in the population. Mutation is the primary way of introducing new genetic information and helps the algorithm escape local optima, but a mutation rate that is too high can cause the algorithm to become randomized and lose directionality. Generally, the mutation rate is set lower to maintain the stability of the algorithm.
#### Selection Strategy
The selection strategy affects which individuals are preserved in the next generation of the population. If the selection pressure is too high, it may cause excellent individuals to dominate the entire population too early, reducing diversity; if the selection pressure is too low, ***mon strategies include roulette wheel selection, tournament selection, etc., each with its own characteristics and applicable scenarios.
### 2.3.2 How to Evaluate the Performance of Genetic Algorithms
Evaluating the performance of genetic algorithms typically involves the following aspects:
1. **Convergence Speed**: The number of iterations it takes for the algorithm to find a satisfactory solution.
2. **Solution Quality**: The degree of closeness of the final solution to the optimal solution.
3. **Stability**: The stability of the solution across multiple runs of the algorithm.
4. **Diversity**: The diversity of individuals in the popula
0
0
相关推荐








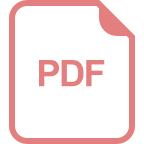