MATLAB Genetic Algorithm Advanced Applications: The Ultimate Guide to Multi-Objective Optimization
发布时间: 2024-09-15 03:56:01 阅读量: 42 订阅数: 45 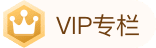
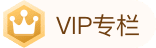
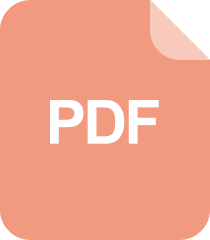
Multi-Objective Optimization using Evolution Algorithms
# Step-by-Step Implementation of a Genetic Algorithm in MATLAB
## Theoretical Foundations and Core Principles of Genetic Algorithms
### Theoretical Foundations and Origins
Genetic Algorithms (GA) are search and optimization algorithms inspired by the principles of natural selection and genetics. They simulate the process of natural evolution and use the "survival of the fittest" mechanism to solve complex optimization problems. In this chapter, we will explore its theoretical foundations and understand the core components of GA.
### The Four Basic Operations of Genetic Algorithms
The basic operations of GA include Selection, Crossover, Mutation, and Fitness Evaluation. Each operation simulates a part of the natural biological evolution process and works together on the population to produce offspring that are more adapted to the environment.
#### Selection
The selection operation simulates the natural selection process within genetic algorithms. It selects individuals based on their fitness, ***mon selection methods include roulette wheel selection, tournament selection, etc.
#### Crossover
The crossover operation is the genetic recombination process in genetic algorithms, simulating the mating process in nature. Crossover is usually performed between two parent individuals, recombining their chromosomes to produce offspring.
#### Mutation
The mutation operation is the random search process in genetic algorithms, where certain genes in individuals are changed with a small probability to ensure the diversity of the population and avoid the algorithm converging prematurely on local optima.
#### Fitness Evaluation
Fitness evaluation is the function in genetic algorithms that measures an individual's ability to adapt to the environment. In optimization problems, the fitness function is usually directly related to the objective function to be optimized, guiding the algorithm to find the optimal solution.
### In-depth Analysis of Core Principles
After understanding the four basic operations of genetic algorithms, we can delve into the core principles of GA, that is, how to use these operations to guide the search process and gradually approach the optimal solution. This process involves key steps such as population initialization, iterative evolution, and convergence analysis, which we will continue to explore in subsequent chapters.
# Using and Implementing MATLAB Genetic Algorithm Toolbox
MATLAB, as a high-performance numerical computing and visualization software, provides a set of functions in its Genetic Algorithm Toolbox (GA Toolbox) to simplify the implementation of genetic algorithms. In this chapter, we will delve into how to use this toolbox in the MATLAB environment, including the introduction of basic concepts, functions, and modules, as well as the implementation of single-objective and multi-objective optimization problems in MATLAB.
## Basic Concepts and Usage Methods of the Toolbox
### Installation and Configuration of the Toolbox
First, ensure your MATLAB environment is the latest version before installing the MATLAB Genetic Algorithm Toolbox, as older versions may not support the latest toolbox. The installation process usually involves unzipping the toolbox files into a folder and then using the `addpath` command in the MATLAB command window to add the folder path to MATLAB's path. For example:
```matlab
addpath('C:\path\to\your\ga\toolbox');
```
After installation and configuration, you can check if the installation was successful by typing `ga` in the command window.
### Main Functions and Modules in the Toolbox
The MATLAB Genetic Algorithm Toolbox provides a series of functions for genetic algorithm operations. The most core function is `ga`, which is used to execute genetic algorithms to solve optimization problems. In addition, it includes a series of auxiliary functions to handle encoding, population initialization, fitness evaluation, selection, crossover, and mutation operations. Here are brief descriptions of some commonly used functions:
- **ga**: The main function for executing genetic algorithms, used to solve optimization problems.
- **gamultiobj**: Used to solve multi-objective optimization problems.
- **gaoptimset**: Creates or modifies the parameter options structure for genetic algorithms.
- **crossover**: The crossover function, used to generate offspring.
- **mutation**: The mutation function, introduces new genetic variation.
## MATLAB Practice for Single-Objective Optimization Problems
### Encoding and Initializing the Population
In MATLAB, genetic algorithms typically use binary, integer, or real number encoding. The choice of encoding method depends on the nature of the specific problem and its requirements. For single-objective optimization problems, initializing the population usually uses the following commands:
```matlab
nvars = 3; % Number of variables
lb = [0, 0, 0]; % Lower bounds of variables
ub = [1, 1, 1]; % Upper bounds of variables
pop = rand(nvars, 20); % Randomly generate an initial population of 20 individuals, within the range [0, 1]
pop = bsxfun(@plus, pop, lb); % Adjust the values in the population to the corresponding range
```
### Implementing Selection, Crossover, and Mutation Operations
The MATLAB Genetic Algorithm Toolbox provides default selection, crossover, and mutation functions, but users can customize these functions according to their needs. Here is a simple example:
```matlab
% The selection function uses the default roulette wheel selection
options = gaoptimset('CreationFcn', {@gacreationuniform, [], nvars, lb, ub});
% The crossover function uses single-point crossover
options = gaoptimset(options, 'CrossoverFcn', {@crossoversinglepoint});
% The mutation function uses uniform mutation
options = gaoptimset(options, 'MutationFcn', {@mutationuniform});
```
### Designing and Evaluating the Fitness Function
The fitness function is the standard for evaluating the quality of individuals, and the design of the fitness function varies for different problems. In MATLAB, define the fitness function as follows:
```matlab
function f = myFitnessFunction(x)
f = x(1)^2 + x(2)^2; % An example of a fitness function
end
```
Then, call this fitness function using the `ga` function:
```matlab
[x, fval] = ga(@myFitnessFunction, nvars, [], [], [], [], lb, ub, [], options);
```
In this way, MATLAB will execute the genetic algorithm and return the optimal solution `x` and the fitness value of the optimal solution `fval`.
## MATLAB Practice for Multi-Objective Optimization Problems
### Theoretical Background of Multi-Objective Optimization
Multi-objective optimization problems involve two or more conflicting objective functions and require finding a set of compromise solutions, known as the Pareto optimal set. In MATLAB, multi-objective genetic algorithms usually use the `gamultiobj` function to solve.
### Implementation and Analysis of the Pareto Front
The Pareto front is a key concept in multi-objective optimization. In MATLAB, the Pareto front can be solved and analyzed using the following methods:
```matlab
% Define two objective functions
function [f1, f2] = myMultiObjFitnessFunction(x)
f1 = x(1)^2;
f2 = (x(2) - 2)^2;
end
% Solve the multi-objective optimization problem
[x, fval] = gamultiobj(@myMultiObjFitnessFunction, nvars, [], [], [], [], lb, ub, options);
```
### Algorithm Selection and Improvement for Multi-Objective Optimization
MATLAB provides different algorithm options for handling multi-objective optimization problems. Users can customize these options, for example, by adjusting the population size, crossover rate, mutation rate, etc. The `gamultiobj` function allows users to set various parameters to optimize algorithm performance.
Next, we will delve into how to customize the development of genetic algorithms in MATLAB, including parameter adjustment, constraint handling, and integration with other optimization methods.
# Customized Development of Genetic Algorithms in MATLAB
As a search and optimization method that simulates natural selection and genetic principles, genetic algorithms have wide applications in engineering and scientific fields. The MATLAB environment, with the help of the genetic algorithm toolbox, can easily implement the optimization of various problems. However, to better adapt to the needs of specific problems, it is often necessary to customize the development of genetic algorithms. This chapter will explore the parameter adjustment, constraint handling, and integration with other optimization methods of genetic algorithms in MATLAB in depth.
## Parameter Adjustment and Optimization of Genetic Algorithms
### Basic Methods for Parameter Adjustment
In MATLAB, the performance of genetic algorithms largely depends on its parameter settings, including population size, crossover rate, mutation rate, selection pressure, etc. The adjustment of these parameters is to maintain population diversity while accelerating the convergence speed to obtain better solutions.
In practical applications, parameter adjustment usually needs to be combined with the characteristics of the problem, for example:
- The population size should be large enough to contain sufficient genetic information, but also avoid excessive population size causing computational burden;
- The crossover rate and mutation rate need to find a balance; a high crossover rate helps information exchange, but too high may lead to convergence too quickly to local optima;
- Too much selection pressure can lead to premature convergence, while too little may cause the algorithm to converge too slowly or fall into random search.
Here is a MATLAB code example showing how to adjust parameters in genetic algorithms:
```matlab
% Define genetic algorithm parameters
options = optimoptions('ga', ...
'PopulationSize', 100, ... % Population size
'CrossoverFraction', 0.8, ... % Crossover rate
'MutationRate', 0.01, ... % Mutation rate
'MaxGenerations', 100, ... % Maximum generations
'EliteCount', 2, ... % Number of elite individuals in elite strategy
'Display', 'iter'); % Display information for each iteration
% Define fitness function
fitnessFunction = @(x) x(1)^2 + x(2)^2;
% Run genetic algorithm
[x,fval] = ga(fitnessFunction, 2, [], [], [], [], [], [], [], options);
```
### Sensitivity Analysis of Crossover Rate and Mutation Rate
The crossover rate and mutation rate are two crucial parameters in genetic algorithms that directly affect the search behavior and performance of the algorithm. The purpose of sensitivity analysis is to assess the impact of these two parameters at different levels on the performance of the algorithm.
Sensitivity analysis can be performed by designing experiments, systematically changing parameter values, and observing the impact on algorithm performance. For example, the following MATLAB code can be used to perform a sensitivity analysis of the crossover rate and mutation rate:
```matlab
% Set the range of crossover rate and mutation rate changes
crossoverRates = linspace(0.6, 1, 10);
mutationRates = linspace(0.001, 0.05, 10);
% Store the optimal solutions and average solutions
```
0
0
相关推荐
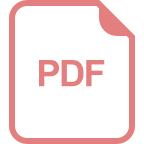
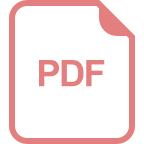
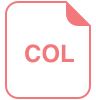
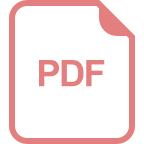
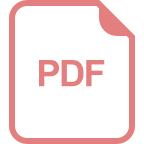
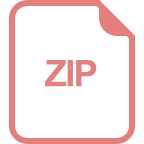
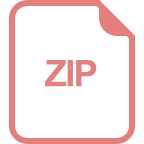
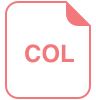