MATLAB Genetic Algorithm Optimization of Neural Network Weights: Applied Research and Practical Guide
发布时间: 2024-09-15 04:34:05 阅读量: 28 订阅数: 45 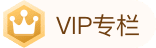
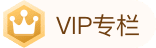
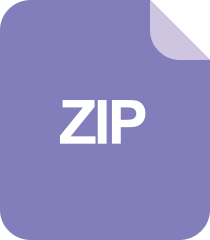
2021最新直播系统+短视频源码+教程+演示APP+开发文档+IOS与安卓源码
# MATLAB Genetic Algorithm Neural Network Weight Optimization: Applied Research and Practical Guide
## 1. Theoretical Foundations of Genetic Algorithms and Neural Networks
### 1.1 Optimization Problems and Heuristic Algorithms
In addressing optimization problems, traditional methods such as linear programming or integer programming may be limited in practical applications due to high computational complexity. Heuristic algorithms, especially Genetic Algorithms (GA) and Neural Networks (NN), offer a new perspective for tackling such issues, particularly suited for nonlinear, multi-modal, and high-complexity optimization problems.
### 1.2 Introduction to Genetic Algorithms
Genetic Algorithms are search algorithms that simulate natural selection and genetic mechanisms, employing three main operations: "selection," "crossover," and "mutation," to mimic the biological evolution process. This approach does not rely on specific domain knowledge of the problem and can efficiently search through complex solution spaces, demonstrating strong global search capabilities and robustness.
### 1.3 Concept of Neural Networks
Neural Networks are computational models composed of numerous interconnected simple nodes that can simulate information processing and learning functions of the human brain. They consist of input layers, hidden layers, and output layers, adjusting inter-layer connection weights to learn data features. Neural Networks have a wide range of applications in pattern recognition, classification, and prediction.
### 1.4 Theoretical Framework for Cross-Application
The theoretical cross-application between Genetic Algorithms and Neural Networks opens new avenues for solving complex optimization problems. Neural Network optimization problems can be addressed by adjusting their weights through Genetic Algorithms to find the optimal network structure and parameters. This combination leverages the global search ability of Genetic Algorithms with the learning and generalization capabilities of Neural Networks, providing a powerful tool for solving optimization problems.
# 2. Fundamental Principles and Implementation of Genetic Algorithms
## 2.1 Core Concepts of Genetic Algorithms
Genetic Algorithms (GA) are search and optimization algorithms that simulate natural selection and genetic mechanisms. Their core concepts include selection, crossover (hybridization), mutation operations, and the design and application of fitness functions.
### 2.1.1 Selection, Crossover, and Mutation Operations
The purpose of selection operations is to choose superior individuals from the current population to pass on to the next generation, hoping that these excellent genes will be preserved and combined to produce even better offspring. Methods include roulette wheel selection and tournament selection.
Crossover operations are the primary means of generating new individuals in Genetic Algorithms, creating offspring by exchanging gene segments between parent individuals. Typical crossover methods include single-point crossover, multi-point crossover, and uniform crossover.
Mutation operations are to maintain diversity in the population and avoid premature convergence to local optimal solutions. Mutation typically randomly changes certain genes in an individual; common types include point mutation and uniform mutation.
```mermaid
flowchart LR
A[Start] --> B[Selection]
B --> C[Crossover]
C --> D[Mutation]
D --> E[Generate New Population]
E --> F[Check if stopping criteria are met]
F --> |Yes| G[End]
F --> |No| B
```
### 2.1.2 Fitness Function in Genetic Algorithms
The design of the fitness function is crucial as it directly affects the outcome of selection operations. The fitness function needs to accurately reflect an individual's ability to adapt to the environment, often a function related to the problem's objective function.
For maximization problems, the fitness function is often designed as the positive value of the objective function (or a transformed positive value), making the higher the objective function value, the higher the individual's fitness.
```mermaid
flowchart LR
A[Start] --> B[Assess Individual Fitness]
B --> C{Is fitness satisfactory?}
C --> |Yes| D[Select Higher Fitness Individuals]
C --> |No| E[Modify Individual Fitness]
D --> F[Crossover and Mutation]
F --> G[Generate New Individuals]
G --> H[Assess New Individual Fitness]
H --> C
```
## 2.2 Coding Strategies of Genetic Algorithms
The coding strategy determines how problem solutions are represented as chromosomes in Genetic Algorithms, with binary coding and real number coding being common.
### 2.2.1 Binary Coding and Real Number Coding
Binary coding is the most common form of coding, representing problem solutions as binary strings, simple to implement, and convenient for crossover and mutation operations. However, its ability to represent complex problems or continuous parameter problems is limited.
Real number coding directly uses real numbers to represent chromosomes, suited for handling continuous parameter problems. It simplifies the coding and decoding process and allows for easy integration with the natural representation of the problem domain.
### 2.2.2 Selection and Design of Coding Schemes
Choosing the appropriate coding scheme has a significant impact on the efficiency of the algorithm and the quality of solutions. For complex problems, it may be necessary to design multi-layer coding schemes, combining the advantages of binary and real number coding.
```mermaid
flowchart LR
A[Start] --> B[Determine Problem Characteristics]
B --> C{Select Coding Scheme}
C --> |Binary Coding| D[Design Binary Coding Strategy]
C --> |Real Number Coding| E[Design Real Number Coding Strategy]
D --> F[Coding Implementation]
E --> F
F --> G[Crossover and Mutation Operations]
G --> H[Assessment and Selection]
H --> I{Have Optimization Goals Been Reached?}
I --> |Yes| J[Output Best Solution]
I --> |No| F
```
## 2.3 Parameter Settings and Optimization of Genetic Algorithms
The setting of algorithm parameters directly affects the algorithm's running efficiency and solution quality. In practice, the determination of population size, crossover rate, and mutation rate is key to parameter settings.
### 2.3.1 Determination of Population Size, Crossover Rate, and Mutation Rate
The population size determines the breadth of the algorithm's search space. A population that is too small may lead to insufficient searching, while a population that is too large increases computational costs. The crossover rate and mutation rate should be appropriately balanced to ensure the algorithm's exploratory and developmental abilities.
### 2.3.2 Evaluation and Adjustment of Algorithm Performance
Common methods for evaluating algorithm performance include convergence speed, solution quality, and stability. Based on evaluation results, algorithm parameters can be adjusted to optimize performance.
```mermaid
flowchart LR
A[Start] --> B[Initialize Parameters]
B --> C[Run Genetic Algorithm]
C --> D[Assess Performance]
D --> E{Is Performance Satisfactory?}
E --> |Yes| F[Output Results]
E --> |No| G[Adjust Parameters]
G --> C
```
In this chapter, we introduced the core operations of genetic algorithms, coding strategies, and the impact of parameter settings on algorithm performance, laying the theoretical foundation for further exploration of how to implement genetic algorithms in MATLAB. The following chapters will specifically introduce how to implement genetic algorithms in MATLAB and perform parameter tuning.
# 3. Theory and Methods for Neural Network Weight Optimization
The performance of neural networks largely depends on the setting of their weights. Appropriate weight selection can improve network prediction accuracy and reduce the risk of overfitting. This chapter will explore the theoretical foundations of neural network weight optimization, analyze weight optimization problems, compare the pros and cons of different optimization strategies, and help readers better understand and apply weight optimization techniques.
## Basic Architecture of Neural Networks
### Neurons and Network Layers
Neural networks consist of a large number of interconnected neurons, where each neuron can be seen as a simple computing unit. These neurons are organized into different layers, forming input layers, hidden layers, and
0
0
相关推荐
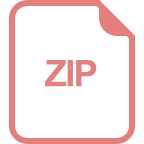
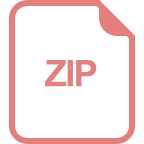
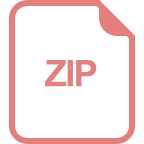
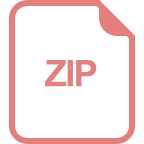
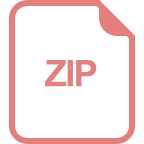
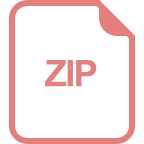