MATLAB Genetic Algorithm Real-World Examples: From Theory to Practice, Witnessing the Power of Optimization
发布时间: 2024-09-15 04:47:35 阅读量: 20 订阅数: 25 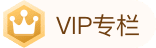
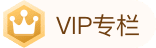
# Genetic Algorithm in Action: From Theory to Practice, Witnessing the Power of Optimization
## 1. Foundations of Genetic Algorithms**
A genetic algorithm is an optimization algorithm inspired by the processes of natural selection and evolution. It searches for the optimal solution in the solution space by simulating the biological evolution process. The basic principles of genetic algorithms include:
***Population:** A group of candidate solutions, known as chromosomes.
***Fitness:** The degree to which each chromosome is adapted to the target function.
***Selection:** Choosing better chromosomes for reproduction based on fitness.
***Crossover:** Exchanging parts of chromosomes to produce new ones.
***Mutation:** Randomly modifying chromosomes to introduce diversity.
Genetic algorithms gradually optimize the population through iterative steps, ultimately finding the optimal or near-optimal solution.
## 2. Genetic Algorithm Programming in MATLAB
### 2.1 MATLAB Genetic Algorithm Toolbox
MATLAB provides a genetic algorithm toolbox containing functions for creating and running genetic algorithms. The main functions include:
**2.1.1 ga function**
The `ga` function is MATLAB's genetic algorithm function for solving optimization problems. It accepts the following parameters:
```
[x,fval,exitflag,output,population,scores] = ga(fitnessfcn,nvars,options)
```
- `fitnessfcn`: A handle to the target function for evaluating individual fitness.
- `nvars`: The number of variables.
- `options`: Genetic algorithm options, including population size, crossover probability, mutation probability, etc.
- `x`: The variable values of the best individual.
- `fval`: The fitness value of the best individual.
- `exitflag`: The algorithm termination flag.
- `output`: Algorithm runtime information, including the number of iterations, best fitness, etc.
- `population`: The final population.
- `scores`: The fitness values of the final population.
**2.1.2 gaoptimset function**
The `gaoptimset` function is used to set genetic algorithm options. It accepts the following parameters:
```
options = gaoptimset('param1',value1,'param2',value2,...)
```
Where `param1` and `value1` ***mon options include:
- `PopulationSize`: Population size.
- `CrossoverFraction`: Crossover probability.
- `MutationRate`: Mutation probability.
- `Generations`: Maximum number of iterations.
- `Display`: Display options, including `off`, `iter`, and `final`.
### 2.2 Genetic Algorithm Parameter Settings
The settings of genetic algorithm parameters have a significant impact on the algorithm's performance. Here are the introductions to the main parameters:
**2.2.1 Population Size**
The population size determines the number of individuals existing simultaneously in the algorithm. A larger population can provide a larger search space but also increases computation time.
**2.2.2 Crossover Probability**
The crossover probability controls the likelihood of two individuals exchanging genetic information (genes). A higher crossover probability can promote population diversity but may also destroy good gene combinations.
**2.2.3 Mutation Probability**
The mutation probability controls the likelihood of random changes in individual genes. A higher mutation probability can prevent the algorithm from getting stuck in local optima but may also introduce harmful mutations.
### 2.3 Genetic Algorithm Process
The genetic algorithm is an iterative process, including the following steps:
**2.3.1 Initialize Population**
Randomly generate a set of individuals to form the initial population. Each individual represents a possible solution.
**2.3.2 Evaluate Fitness**
Calculate the fitness of each individual, i.e., the value of the target function. Individuals with higher fitness are more likely to be selected for reproduction.
**2.3.3 Selection**
***mon selection methods include roulette wheel selection, tournament selection, and elitist selection.
**2.3.4 Crossover**
Combine two selected individuals to produce new individuals. The crossover operation can exchange genes between two individuals, creating new solutions.
**2.3.5 Mutation**
Perform mutations on the new individuals, i.e., randomly change some genes. The mutation operation can introduce new gene combinations, preventing the algorithm from getting stuck in loc
0
0
相关推荐
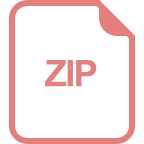
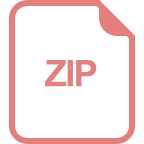
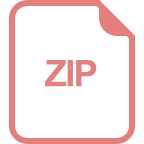
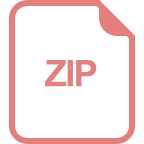
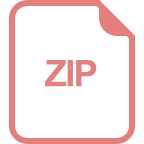
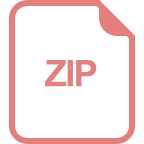
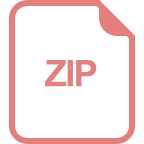
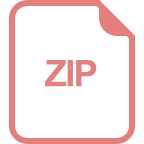
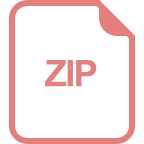