Ethical Considerations of Genetic Algorithms in MATLAB: Responsible Use of Optimization Technologies
发布时间: 2024-09-15 04:50:26 阅读量: 16 订阅数: 27 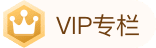
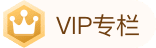
# 1. Introduction to MATLAB Genetic Algorithms
MATLAB Genetic Algorithm (GA) is a powerful optimization tool that simulates the process of natural selection to solve complex problems. GA follows these steps:
***Initialization:** Randomly generate a set of candidates called chromosomes.
***Selection:** Choose the fittest chromosomes based on their quality (i.e., the quality of the solutions).
***Crossover:** Combine two selected chromosomes to create new ones.
***Mutation:** Randomly modify new chromosomes to introduce diversity.
***Repetition:** Repeat these steps until a stopping condition is met (e.g., reaching the maximum number of iterations or finding the optimal solution).
The advantages of GA include:
***Robustness:** Capable of handling complex, nonlinear problems.
***Global Search:** Avoids getting stuck in local optima.
***Parallelization:** Performance can be enhanced through parallel processing.
# 2. Ethical Considerations of Genetic Algorithms
Genetic Algorithms (GAs) are widely used as powerful optimization tools across various domains. However, as their influence grows, so does the attention on their ethical implications. This chapter delves into the ethical considerations of GAs, including bias and discrimination, transparency and interpretability, and responsibility and accountability.
### 2.1 Bias and Discrimination
#### 2.1.1 Sources of Bias in Algorithms
GAs may produce bias due to:
- **Bias in training data:** If the training data itself is biased, GAs may learn and amplify these biases.
- **Algorithm design:** Parameters and selection strategies in GA algorithms can lead to bias, for example, excessive selection pressure may cause the algorithm to converge on local optima, ignoring potentially fairer solutions.
- **Human intervention:** The development and deployment of GA algorithms involve human decision-making, which can introduce bias, such as the selection of specific features or objective functions.
#### 2.1.2 Methods to Mitigate Bias
Mitigating bias in GA algorithms is crucial and can be achieved through:
- **Ensuring fairness in training data:** Collecting and preprocessing training data should ensure its fairness and representativeness, avoiding bias.
- **Optimizing algorithm parameters:** Adjust GA parameters through cross-validation and hyperparameter optimization to maximize fairness and generalization.
- **Introducing fairness constraints:** Incorporate fairness constraints in GA algorithms to ensure the solutions meet specific fairness standards.
- **Using fairness metrics:** Employ fairness metrics like disparate impact or fairness index to evaluate the fairness of GA algorithms.
### 2.2 Transparency and Interpretability
#### 2.2.1 Challenges to GA Transparency
GA transparency and interpretability face challenges due to:
- **Algorithm complexity:** GAs involve multiple components and parameters, making them difficult to understand and interpret.
- **Randomness:** The randomness of GA algorithms increases the difficulty of interpretability, as results may vary with each run.
- **Black-box models:** Some GAs are considered black-box models, meaning their internal workings are difficult to comprehend.
#### 2.2.2 Strategies to Improve Interpretability
Improving GA interpretability is vital and can be achieved through:
- **Visualization:** Use visualization techniques such as decision trees or scatter plots to display the running process and results of GA algorithms.
- **Simplifying algorithms:** Simplify GAs to make them easier to understand and interpret while maintaining their optimization capabilities.
- **Interpretability methods:** Use interpretability methods like SHAP or LIME to explain the decision-making process of GA algorithms.
- **Documentation:** Document the design, implementation, and evaluation process of GA algorithms in detail to improve transparency.
### 2.3 Responsibility and Accountability
#### 2.3.1 Responsibilities of GA Developers
GA developers have the following responsibilities:
- **Ensuring fairness and interpretability:** Developers should take measures to ensure GA algorithms are fair and interpretable and consider their potential ethical impacts.
- **Providing transparency and documentation:** Developers should provide transparency and documentation for their algorithms, allowing users to understand their workings and limitations.
- **Education and training:** Developers should educate and train users of their algorithms, helping them understand their ethical impacts and responsible use practices.
#### 2.3.2 Responsibilities of GA Users
GA users have the following responsibilities:
- **Understanding the ethical implications:** Users should be aware of the potential ethical implications of GA algorithms and consider their fairness and interpretability when using them.
- **Using algorithms responsibly:** Users should use GA algorithms responsibly, avoiding the creation or amplification of bias and discrimination.
- **Evaluating algorithm performance and bias:** Users should assess the performance and bias of GA algorithms and take steps to mitigate any negative impacts.
# 3. Responsible GA Practices
### 3.1 Data Collection and Preprocessing
#### 3.1.1 Ensuring Data Fairness and Representativeness
Responsible GA practices begin with ensuring that the data used for training and evaluating algorithms is fair and representative. This is critical to prevent bias and d
0
0
相关推荐
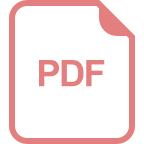
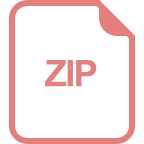
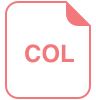
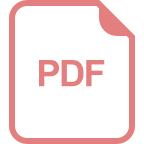
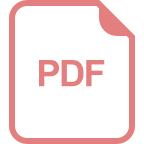
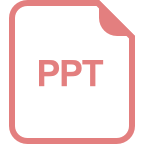
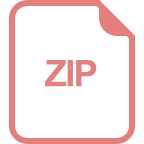
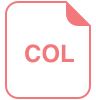