【Advanced】Image Depth Estimation in MATLAB: Using Deep Learning for Image Depth Estimation
发布时间: 2024-09-15 03:23:04 阅读量: 65 订阅数: 60 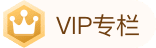
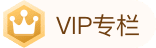
## 2.1 The Architecture and Principles of Deep Neural Networks
A Deep Neural Network (DNN) is a machine learning model with multiple processing units (neurons) organized in layers connected by weighted links. The architecture of a DNN typically consists of an input layer, hidden layers, and an output layer.
### 2.1.1 Convolutional Neural Networks (CNN)
A CNN is a type of DNN, specialized for handling data with a grid-like structure, such as images. The main feature of a CNN is the use of convolutional operations, which extract features by sliding a small filter, known as a convolutional kernel, over the input data. The weights in the convolutional kernel are used to calculate the feature map for each input location, resulting in a feature representation that is invariant to spatial translations.
### 2.1.2 Recurrent Neural Networks (RNN)
An RNN is a type of DNN, designed for sequential data, such as text or time series. The primary feature of an RNN is the use of memory cells that can store information from the past and pass it on to subsequent layers of the network. RNNs are suitable for tasks that require an understanding of dependencies between elements in a sequence, such as language modeling and time-series prediction.
# 2. Applications of Deep Learning in Image Depth Estimation
### 2.1 The Architecture and Principles of Deep Neural Networks
A Deep Neural Network (DNN) is a powerful machine learning model composed of multiple nonlinear layers that can learn complex patterns from data. DNNs are widely applied in image depth estimation due to their ability to process high-dimensional data and learn spatial relationships within images.
#### 2.1.1 Convolutional Neural Networks (CNN)
A CNN is a specialized form of DNN that uses convolutional operations to process data. Convolutional operations involve sliding a convolutional kernel (a small matrix of weights) ***Ns are particularly effective in image depth estimation as they can extract local features and spatial relationships from images.
#### 2.1.2 Recurrent Neural Networks (RNN)
An RNN is another type of DNN that utilizes recurrent connections to process sequential data. RNNs are used in image depth estimation for time series data, such as video sequences. They are capable of learning temporal relationships within image sequences, which is particularly useful for estimating depth in dynamic scenes.
### 2.2 Training and Evaluating Deep Learning Models
#### 2.2.1 Preparing and Preprocessing Training Datasets
Training deep learning models requires a large amount of labeled data. Image depth estimation datasets typically include pairs of images and depth maps. Depth maps provide the depth values of each pixel in the image. Before training, the data usually needs to be preprocessed, including resizing, cropping, and normalization.
#### 2.2.2 Hyperparameter Optimization for Model Training
Training deep learning models involves adjusting many hyperparameters, such as learning rate, batch size, and regularization parameters. These hyperparameters can affect the model's performance, so careful optimization is necessary. Hyperparameter optimization can be accomplished through techniques such as grid search or Bayesian optimization.
### 2.3 Examples of Deep Learning Model Application in Image Depth Estimation
#### 2.3.1 Monocular Depth Estimation Based on Deep Learning
Monocular depth estimation is the task of estimating a depth map from a single image. Deep learning models have been extensively used for monocular depth estimation and have achieved impressive results. These models typically use CNNs to extract image features and then use deconvolutional layers or other techniques to generate depth maps.
#### 2.3.2 Multi-view Depth Estimation Based on Deep Learning
Multi-view depth estimation is the task of estimating depth maps from multiple images. Deep learning models have also been applied to multi-view depth estimation, leveraging the consistency information from multiple images to improve accuracy. These models typically use CNNs or RNNs to process multiple images and generate a fused depth map.
**Code Example:**
```python
import tensorflow as tf
# Define a simple CNN model
model = tf.keras.models.Sequential([
tf.keras.layers.Conv2D(32, (3, 3), activation='relu'),
tf.keras.layers.MaxPooling2D((2, 2)),
tf.keras.layers.Conv2D(64, (3, 3), activation='relu'),
tf.keras.layers.MaxP
```
0
0
相关推荐








