【Advanced】Stereo Matching of Images in MATLAB: Using SGBM Algorithm for Image Stereo Matching
发布时间: 2024-09-15 03:24:19 阅读量: 66 订阅数: 50 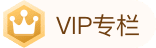
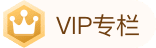
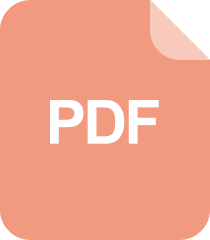
PM-PM: PatchMatch With Potts Model for Object Segmentation and Stereo Matching
## 2.1 Principles of Image Stereo Matching
Image stereo matching is a computer vision technique that utilizes a pair of stereoscopic images with overlapping areas to generate depth information of a scene. The principle behind this technology is that when an observer views the same scene from different angles, due to the presence of disparity, the projection positions of corresponding points in the image will shift. By measuring these disparities, we can calculate the distance of objects in the scene from the observer.
The image stereo matching process typically includes the following steps:
- **Image Registration:** Aligning two stereoscopic images so that they share the same viewpoint and coordinate system.
- **Disparity Calculation:** For each pixel in the image, calculate the disparity of its corresponding point in the other image.
- **Depth Calculation:** Based on the disparity and known camera parameters, calculate the depth value for each pixel in the scene.
## 2. Theoretical Foundations of SGBM Algorithm
### 2.1 Principles of Image Stereo Matching
Image stereo matching is an important technique in computer vision, aimed at recovering depth information from a pair of overlapping images. The principle of stereo matching is based on the binocular vision system of the human eye. When the human eye observes an object, due to the disparity between the two eyes, different images are produced. The brain fuses these two images, allowing for the perception of depth of objects.
Stereo matching algorithms simulate this process. Given a pair of images with overlapping areas, the algorithm first seeks matches for corresponding points in the images. Then, based on the disparity of the matched points, the depth value for each pixel point in the scene is calculated.
### 2.2 Principles of SGBM Algorithm
The SGBM (Semi-Global Block Matching) algorithm is a local stereo matching algorithm that divides the image into small blocks and performs local matching on each block. The SGBM algorithm mainly includes the following steps:
1. **Cost Calculation:** For each pixel in the image, calculate the cost of all possible matching points in the other image. Cost functions often use pixel intensity differences or normalized cross-correlation coefficients (NCC) as measures.
2. **Local Matching:** For each pixel in the image, select the matching point with the smallest cost as its corresponding point.
3. **Cost Aggregation:** Aggregate the local matching results to reduce noise and improve matching accuracy. The SGBM algorithm uses a technique called "semi-global matching," which aggregates local matching results within the range of image blocks.
4. **Disparity Calculation:** Based on the disparity of the matching points, calculate the depth value for each pixel point in the scene.
The advantage of the SGBM algorithm lies in its high computational efficiency and its ability to handle images with less texture or occlusions. However, its drawback is that the matching accuracy may be lower than other global stereo matching algorithms.
**Code Block:**
```python
import cv2
# Calculate cost
cost = cv2.StereoBM_compute(left_image, right_image, numDisparities=16, blockSize=5)
# Local matching
disp = cv2.StereoBM_compute(left_image, right_image, numDisparities=16, blockSize=5)
```
**Logical Analysis:**
* The `cv2.StereoBM_compute()` function is used to calculate the cost and disparity map for image stereo matching.
* The `numDisparities` parameter specifies the maximum disparity range that the algorithm searches.
* The `blockSize` parameter specifies the size of the local matching block.
**Parameter Explanation:**
* `left_image`: Left image
* `right_image`: Right image
* `numDisparities`: Maximum disparity range
* `blockSize`: Size of the local matching block
**mermaid Flowchart:**
```mermaid
graph LR
subgraph Calculate Cost
A[Calculate Cost]
end
subgraph Local Matching
B[Local Matching]
end
subgraph Cost Aggregation
C[Cost Aggregation]
end
subgraph Disparity Calculation
D[Disparity Calculation]
end
A --> B
B --> C
C --> D
```
**Table:**
| Parameter | Description |
|---|---|
| `numDisparities` | Maximum disparity range |
| `blockSize` | Size of local matching blocks |
| `minDisparity` | Minimum disparity value |
| `textureThreshold` | Texture threshold |
# 3.1 Introduction to MATLAB Image Processing Library
MATLAB (Matrix Laboratory) is a programming language an
0
0
相关推荐
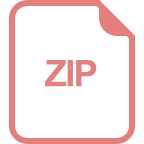
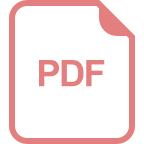
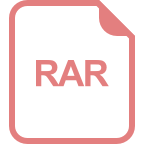
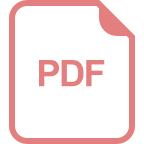
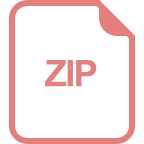
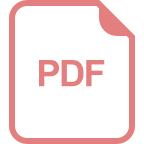
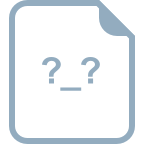
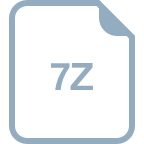