【Advanced】Image Quality Assessment in MATLAB: Calculating SSIM and PSNR
发布时间: 2024-09-15 03:01:42 阅读量: 20 订阅数: 37 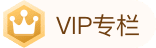
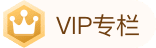
## 2.1 Principles and Formula of SSIM
SSIM (Structural Similarity) is an image quality assessment metric that measures the similarity between two images in terms of luminance, contrast, and structure. The fundamental principle involves dividing the images into small blocks and then calculating the SSIM value for each block.
The SSIM formula is as follows:
```
SSIM(x, y) = (2μ_xμ_y + C_1)(2σ_{xy} + C_2) / ((μ_x^2 + μ_y^2 + C_1)(σ_x^2 + σ_y^2 + C_2))
```
Where:
* x and y are two images
* μ_x and μ_y are the averages of x and y
* σ_x and σ_y are the standard deviations of x and y
* σ_{xy} is the covariance of x and y
* C_1 and C_2 are constants used to stabilize the calculation
## 2. SSIM (Structural Similarity) Image Quality Assessment
### 2.1 Principles and Formula of SSIM
Structural Similarity (SSIM) is an image quality assessment metric that measures the similarity between two images in terms of structure, luminance, and contrast. The SSIM calculation formula is as follows:
```
SSIM(x, y) = (2μxμy + C1)(2σxy + C2) / ((μx^2 + μy^2 + C1)(σx^2 + σy^2 + C2))
```
Where:
* `x` and `y` are two images
* `μx` and `μy` are the means of `x` and `y`
* `σx^2` and `σy^2` are the variances of `x` and `y`
* `σxy` is the covariance of `x` and `y`
* `C1` and `C2` are constants used to stabilize the calculation
### 2.2 Steps and Code Example for SSIM Implementation
**Steps:**
1. Calculate the mean, variance, ***
***pute the SSIM value based on the formula.
**Code Example:**
```python
import numpy as np
def ssim(x, y):
"""
Calculate the SSIM value for two images.
Parameters:
x (ndarray): First image.
y (ndarray): Second image.
Returns:
float: SSIM value.
"""
# Calculate mean, variance, and covariance
μx = np.mean(x)
μy = np.mean(y)
σx2 = np.var(x)
σy2 = np.var(y)
σxy = np.cov(x, y)[0, 1]
# Calculate SSIM value
C1 = (0.01 * 255)**2
C2 = (0.03 * 255)**2
ssim_value = (2 * μx * μy + C1) * (2 * σxy + C2) / ((μx**2 + μy**2 + C1) * (σx2 + σy2 + C2))
return ssim_value
```
## 3. PSNR (Peak Signal-to-Noise Ratio) Image Quality Assessment
### 3.1 Principles and Formula of PSNR
Peak Signal-to-Noise Ratio (PSNR) is an image quality assessment metric used to measure the difference between an original image and a distorted image. The basic principle involves calculating the Mean Squared Error (MSE) between the pixel values of the distorted image and the corresponding pixel values of the original image, and then converting this into a Signal-to-Noise Ratio (SNR).
The PSNR formula is as follows:
```
PSNR = 10 * log10(MAX_I^2 / MSE)
```
Where:
* `MAX_I` is the maximum possible pixel value in the image (usually 255)
* `MSE` is the Mean Squared Error between the pixel values of the original and distorted images
### 3.2 Steps and Code Example for PSNR Implementation
The steps to implement PSNR in MATLAB are as follows:
1. **Import the original and distorted images:**
```
original_image = imread('original_image.jpg');
distorted_image = imread('distorted_image.jpg');
```
2. **Calculate the Mean Squared Error (MSE) between pixel values:**
```
mse = mean((double(original_image) - double(distorted_image)).^2, 'all');
```
3. **Calculate the Peak Signal-to-Noise Ratio (PSNR):**
```
psnr = 10 * log10(255^2 / mse);
```
### Code Logic Line-by-Line Explanation
```
% Import the original and distorted images
original_image = imread('original_image.jpg');
distorted_image = imread('d
```
0
0
相关推荐
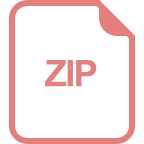
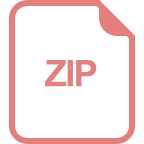
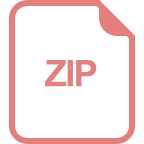





