【Basic】Image Registration in MATLAB: Aligning and Registering Images
发布时间: 2024-09-15 02:29:26 阅读量: 25 订阅数: 38 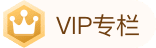
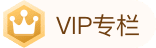
# 1. Overview of Image Registration
Image registration is a process that aligns and overlaps two or more images to make them spatially consistent in geometry. This technique is widely used in computer vision and image processing fields, including medical imaging, remote sensing, and industrial inspection.
The goal of image registration is to find a transformation model that maps pixels from one image (referred to as the source image) to the corresponding positions in another image (referred to as the target image). By applying this transformation model, the source image can be aligned with the target image, thus achieving image registration.
# 2. Theoretical Foundations of Image Registration
### 2.1 Mathematical Models of Image Registration
The mathematical models of image registration are built on two foundations: image transformation and similarity measurement.
#### 2.1.1 Transforma***
***mon transformation models include:
- **Affine Transform:** A combination of translation, rotation, scaling, and shearing.
- **Projective Transform:** A perspective transform that projects an image onto another plane.
- **Elastic Transform:** A nonlinear transform that allows local deformation of the image.
#### 2.1.2 Similarity Measure***
***mon similarity measurements include:
- **Root Mean Square Error (RMSE):** The square root of the squared differences between pixel values.
- **Mutual Information:** A measure of the joint probability distribution in the images.
- **Normalized Cross-Correlation (NCC):** A measure of the correlation of pixel values in the images.
### 2.2 Image Registration Algorithms
Image registration algorithms use mathematical models and similarity measurements to calculate the optimal image transformation that aligns the source image with the target image. These algorithms are divided into three categories:
#### 2.2.1 Feature-Based Algorithms
Feature-based algorithms detect and match feature points (e.g., corners, edges) in the images to compute the transformation.
- **Scale-Invariant Feature Transform (SIFT):** Detects and matches key points that are robust to image scaling and rotation.
- **Speeded-Up Robust Features (SURF):** A faster variant of SIFT with similar performance.
#### 2.2.2 Region-Based Algorithms
Region-based algorithms segment the image into regions and then match these regions.
- **Block Matching Algorithm:** Divides the image into small blocks and computes the transformation by minimizing the error between blocks.
- **Phase Correlation (PC):** Computes the transformation by calculating the phase difference of the image spectra.
#### 2.2.3 Pixel-Based Algorithms
Pixel-based algorithms directly compare pixel values in the images.
- **Least Squares Method (LS):** Minimizes the error between pixel values to calculate the transformation.
- **Maximum Likelihood Estimation (MLE):** Assumes that pixel values follow a specific distribution and maximizes the likelihood function to calculate the transformation.
# 3. Image Registration in Practice with MATLAB
### 3.1 Image Reading and Preprocessing
#### 3.1.1 Image Reading
MATLAB provides various functions to read images, including `imread`, `imfinfo`, and `dicomread`. The `imread` function is used for general image formats such as JPEG, PNG, and TIFF. The `imfinfo` function provides information about the image file, such as size, format, and color space. The `dicomread` function is specifically designed for reading DICOM medical image files.
```matlab
% Reading images
image1 = imread('image1.jpg');
image2 = imread('image2.jpg');
```
#### 3.1.2 Image Preprocessing
Image preprocessing is an i***mon preprocessing techniques include:
***Grayscale Conversion:** Converts color images to grayscale to reduce the interference of color information.
***Noise Removal:** Uses filters (such as median or Gaussian filters) to remove noise from the image.
***Image Enhancement:** Adjusts image contrast, brightness, and gamma values to improve clarity.
```matlab
% Grayscale conversion
image1_gray = rgb2gray(image1);
image2_gray = rgb2gray(image2);
% Noise removal
image1_denoised = medfilt2(image1_gray);
image2_denoised = medfilt2(image2_gray);
```
### 3.2 Implementation of Image Registration Algorithms
#### 3.2.***
***mon feature extraction methods include:
***Scale-Invariant Feature Transform (SIFT):** Detects local features in the image and computes their descriptors.
***Speeded-Up Robust Features (SURF):** Similar to SIFT but faster in computation.
***Histogram of Oriented Gradients (HOG):** Calculates the histogram of gradients of pixels in the image to form feature vectors.
```matlab
% Feature extraction
[features1, descriptors1] = vl_sift(image1_gray);
[features2, descriptors2] = vl_sift(image2_gray);
% Feature matching
matches = vl_ubcmatch(descriptors1, descriptors2);
```
#### 3.2.2 Region-Based Algo***
***mon region segmentation methods include:
***Region Growing:** Groups together pixels with similar features starting from seed points.
***Watershed Transform:** Treats the image
0
0
相关推荐
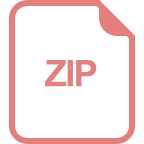
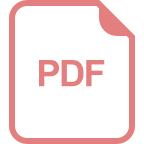
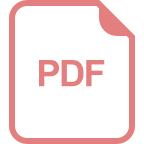
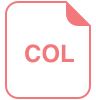
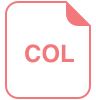
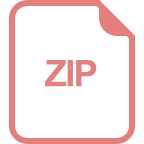
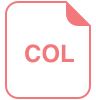
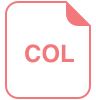
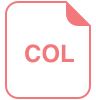