【Practical Exercise】Image Denoising Algorithms Based on Matlab: Gaussian Filtering, Mean Filtering, Median Filtering, and Bilateral Filtering
发布时间: 2024-09-15 03:33:10 阅读量: 27 订阅数: 62 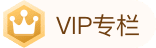
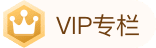
# 2.1 Principle of Gaussian Filtering
Gaussian filtering is a type of linear smoothing filter that uses a Gaussian function as its convolution kernel. The Gaussian function is a bell-shaped curve with the highest value at its center, gradually decreasing towards the sides. The principle behind Gaussian filtering is to convolve the Gaussian function with an image, thereby performing a weighted average on each pixel of the image.
The expression for the Gaussian function is:
```
G(x, y) = (1 / (2πσ^2)) * exp(-(x^2 + y^2) / (2σ^2))
```
Where σ is the standard deviation of the Gaussian function, which controls the smoothness of the filter. The larger σ is, the smoother the filter becomes, the more noise is removed from the image, but more details are also lost.
# 2. Gaussian Filtering
### 2.1 Principle of Gaussian Filtering
Gaussian filtering is a linear smoothing filter that uses a Gaussian function as its filter kernel. The Gaussian function is a bell-shaped curve, shaped by the standard deviation σ. The larger the standard deviation, the smoother the curve, and the stronger the filtering effect.
The mathematical formula for Gaussian filtering is as follows:
```
G(x, y) = (1 / (2πσ^2)) * exp(-(x^2 + y^2) / (2σ^2))
```
Where (x, y) are the pixel coordinates, and σ is the standard deviation.
### 2.2 Implementation of Gaussian Filtering
#### 2.2.1 Gaussian Filtering Function in Matlab
Matlab provides the `imgaussfilt` function for Gaussian filtering. The syntax for this function is:
```
B = imgaussfilt(A, sigma)
```
Where `A` is the input image and `sigma` is the standard deviation.
#### 2.2.2 Gaussian Filtering Parameter Settings
The main parameters for Gaussian filtering include the standard deviation `sigma`. The larger the standard deviation, the stronger the filtering effect, but it also leads to more image blur. Typically, it is recommended to set the standard deviation to an estimate of the noise in the image.
```
% Original image
I = imread('noisy_image.png');
% Set the standard deviation
sigma = 2;
% Perform Gaussian filtering
J = imgaussfilt(I, sigma);
% Display the original and filtered images
subplot(1, 2, 1);
imshow(I);
title('Original Image');
subplot(1, 2, 2);
imshow(J);
title('Image after Gaussian Filtering');
```
**Code Logic Analysis:**
* `imread('noisy_image.png')`: Reads the noisy image.
* `sigma = 2`: Sets the standard deviation to 2.
* `J = imgaussfilt(I, sigma)`: Performs Gaussian filtering on image `I`, storing the result in `J`.
* `subplot(1, 2, 1)`: Creates the first subplot to display the original image.
* `imshow(I)`: Displays the original image.
* `title('Original Image')`: Sets the title for the subplot.
* `subplot(1, 2, 2)`: Creates the second subplot to display the denoised image.
* `imshow(J)`: Displays the denoised image.
* `title('Image after Gaussian Filtering')`: Sets the title for the subplot.
**Parameter Description:**
* `sigma`: The standard deviation of the Gaussian function, controlling the filtering effect.
**Code Extension:**
```
% Comparison of Gaussian filtering effects with different standard deviations
sigma_values = [1, 2, 4, 8];
for i = 1:length(sigma_values)
sigma = sigma_values(i);
J = imgaussfilt(I, sigma);
subplot(2, 2, i);
imshow(J);
title(['Standard Deviation = ' num2str(sigma)]);
end
```
**Code Logic Analysis:**
* `sigma_values = [1, 2, 4, 8]`: Defines different standard deviation values.
* `for i = 1:length(sigma_values)`: Iterates through the standard deviation values.
* `sigma = sigma_values(i)`: Sets the current standard deviation.
* `J = imgaussfilt(I, sigma)`: Performs Gaussian filtering on image `I`, storing the result in `J`.
* `subplot(2, 2, i)`: Creates a subplot to display the denoised image with the current standard deviation.
* `imshow(J)`: Displays the denoised image.
* `title(['Standard Deviation = ' num2str(sigma)])`: Sets the title for the subplot, displaying the current standard deviation.
**Parameter Description:**
* `sigma_values`: Different standard deviation values.
# 3. Mean Filtering
### 3.1 Principle of Mean Filtering
Mean filtering is a nonlinear filtering technique that replaces the value of a pixel with the average value of the pixels in its surrounding neighborhood. The main principle is as follows:
1. **Define a neighborhood window:** For each pixel in the image, define a fixed-size neighborhood window, usually square or circular.
2. **Calculate the neighborhood average:** For each pixel, calculate the average value of all pixel values in its neighborhood window.
3. **Replace pixel values:** Replace the original pixel value with the neighborhood average.
The adva
0
0
相关推荐








