【Advanced】Image Tracking in MATLAB: Tracking Images with KCF Algorithm
发布时间: 2024-09-15 03:11:31 阅读量: 26 订阅数: 43 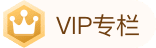
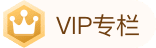
# 2.1 Fundamental Principles of Object Tracking
Object tracking is a significant task in the field of computer vision, aimed at estimating the position and size of an object within a sequence of continuous images. Object tracking algorithms generally follow these basic principles:
1. **Object Initialization:** Manually or automatically initialize the position and size of the object in the first frame of the image sequence.
2. **Object Prediction:** Predict the object's position in the current frame based on the state of the object in the previous frame.
3. **Object Search:** Search for the object within the search area of the current frame and calculate the similarity to the predicted position.
4. **Object Update:** Update the object's position and size based on the search results.
5. **Cyclic Iteration:** Repeat steps 2-4 until the tracking ends or the object is lost.
# 2. Theoretical Foundations of Image Tracking
### 2.1 Fundamental Principles of Object Tracking
Object tracking is a fundamental task in computer vision, with the goal of estimating the position and state of an object within a sequence of continuous images. Object tracking algorithms typically follow these basic principles:
- **Object Representation:** Object tracking algorithms need a method to represent the object. This can be the object's bounding box, contour, or other features.
- **Motion Model:** Object tracking algorithms need to predict the object's position in the next frame. This is usually accomplished using a motion model, such as a Kalman filter or particle filter.
- **Appearance Model:** Object tracking algorithms need to learn the appearance of the object to identify it in subsequent frames. This is typically done using feature extraction techniques, such as histograms or deep learning models.
- **Matching Strategy:** Object tracking algorithms need a strategy to match the representation of the object in the current frame with the representation of the object in the previous frame. This is usually accomplished using similarity metrics or probability models.
### 2.2 Principles and Implementation of the KCF Algorithm
The Kernelized Correlation Filters (KCF) algorithm is a widely used object tracking algorithm. It is based on correlation filters and utilizes the object's appearance information and motion model to predict the object's position. The principles of the KCF algorithm are as follows:
- **Training Phase:** During the training phase, the KCF algorithm extracts features from the object's bounding box. It then uses these features to train a correlation filter.
- **Tracking Phase:** In the tracking phase, the KCF algorithm uses the trained correlation filter to search for the object in the current frame. The correlation filter outputs a response map, where peaks indicate the location of the object.
- **Update Phase:** In the update phase, the KCF algorithm updates the object's appearance model and motion model. This ensures that the algorithm can adapt to changes in the object's appearance and motion.
**Implementation of the KCF Algorithm:**
```matlab
% Create an object bounding box
boundingBox = [x, y, width, height];
% Extract features
features = extractFeatures(image, boundingBox);
% Train the correlation filter
filter = trainFilter(features);
% Track the object
while true
% Obtain the current frame
image = getCurrentFrame();
% Predict the object's location
predictedBoundingBox = predictLocation(filter, image);
% Update the correlation filter
filter = updateFilter(filter, image, predictedBoundingBox);
% Draw the object bounding box
drawBoundingBox(image, predictedBoundingBox);
end
```
**Code Logic Analysis:**
- The `extractFeatures()` function extracts features from the object bounding box.
- The `trainFilter()` function uses the extracted features to train the correlation filter.
- The `predictLocation()` function uses the correlation filter to predict the object's location in the current frame.
- The `updateFilter()` function updates the correlation filter to adapt to changes in the object's appearance and motion.
- The `drawBoundingBox()` function draws the object bounding box on the image.
**Parameter Description:**
- `image`: The image of the current frame.
- `boundingBox`: The object's bounding box.
- `filter`: The correlation filter.
- `predictedBoundingBox`: The predicted object bounding box.
# 3. MATLAB Image Tracking Practice
### 3.1 Setting Up the MATLAB Image Tracking Environment
**1. Install MATLAB**
* Download and install the MATLAB software (recommended to use the latest version).
* Follow the prompts during the installati
0
0
相关推荐
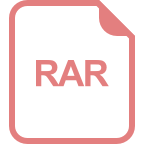
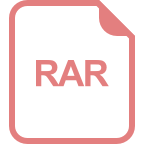
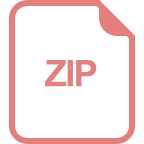
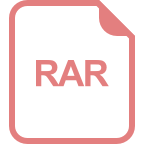
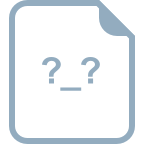
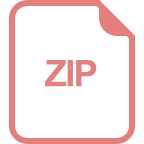
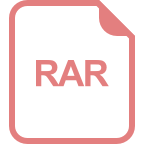
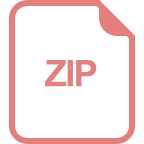
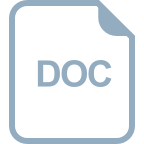