【Basics】Image Reading and Display in MATLAB: Reading Images from File and Displaying Them
发布时间: 2024-09-15 02:15:00 阅读量: 25 订阅数: 42 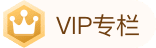
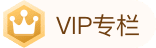
# 1. An Overview of MATLAB Image Processing
The MATLAB Image Processing Toolbox is a powerful set of functions designed for the processing and analysis of digital images. It offers a variety of functions that can be used for image reading, display, enhancement, segmentation, feature extraction, and matching. The toolbox is widely used across various fields, including medicine, remote sensing, industrial automation, and scientific research.
# 2. Image Reading and Display
### 2.1 Image File Formats and Reading Functions
#### 2.1.1 Common Image File Formats
Image file formats determine the storage method and compression algorithms of image data. Some commonly used image formats are:
- **Bitmap (BMP)**: A lossless format, but with larger file sizes.
- **JPEG (JPG)**: A lossy compression format with adjustable compression rates that balance image quality against file size.
- **PNG (Portable Network Graphics)**: A lossless compression format that supports transparency.
- **TIFF (Tagged Image File Format)**: A lossless format often used for professional image processing.
- **GIF (Graphics Interchange Format)**: A lossy compression format that supports animations and transparency.
#### 2.1.2 MATLAB Image Reading Functions
MATLAB provides a variety of functions for reading images, selecting the appropriate one based on the image file format:
- `imread`: Reads various image file formats, such as BMP, JPEG, PNG, TIFF, etc.
- `imfinfo`: Retrieves information about the image file, including size, format, color space, etc.
**Code Block: Reading an Image**
```matlab
% Reading a JPEG image
image = imread('image.jpg');
% Getting image information
info = imfinfo('image.jpg');
```
**Logical Analysis:**
- The `imread` function retrieves the specified JPEG image and stores it in the variable `image`.
- The `imfinfo` function returns a structure containing metadata about the image, such as `info.Width`, `info.Height`, `info.ColorType`, etc.
### 2.2 Image Display Functions and Parameters
#### 2.2.1 MATLAB Image Display Functions
MATLAB provides the `imshow` function to display images:
- `imshow(image)`: Displays the specified image `image`.
- `imshow(image, [low, high])`: Displays the image with the specified display range for pixel values.
#### 2.2.2 Image Display Parameter Settings
The `imshow` function supports various parameters to control image display:
- `'InitialMagnification'`: Sets the initial magnification factor for the image.
- `'Border'`: Specifies the color of the border around the image.
- `...
# 3. Basic Operations in Image Processing
### 3.1 Image Conversion and Type Conversion
#### 3.1.1 Image Format Conversion
Image format conversion involves changing an image from one format to another. MATLAB offers various functions for image format conversion, the commonly used ones include:
- `imread`: Reads images from files, supporting various formats such as JPEG, PNG, BMP, etc.
- `imwrite`: Writes images to files, supporting various formats such as JPEG, PNG, BMP, etc.
- `imformats`: Retrieves information about supported image formats.
**Code Block:**
```matlab
% Reading a JPEG image
image = imread('image.jpg');
% Converting the image to PNG format
imwrite(image, 'image.png', 'PNG');
```
**Logical Analysis:**
* The `imread` function reads the JPEG image and stores it in the variable `image`.
* The `imwrite` function converts the image in the `image` variable to PNG format and writes it to the file `image.png`.
#### 3.1.2 Image Data Type Conversion
Image data type conversion refers to changing image data from one data type to another. MATLAB supports various image data types, with common ones including:
- `uint8`: 8-bit unsigned integer with a range of 0-255.
- `uint16`: 16-bit unsigned integer with a range of 0-65535.
- `double`: 64-bit floating-point with a range from -Inf to Inf.
**Code Block:**
```matlab
% Converting a uint8 image to double
image_double = im2double(image);
% Converting a double image to uint8
image_uint8 = im2uint8(image_double);
```
**Logical Analysis:**
* The `im2double` function converts the uint8 image in the `image` variable to a double image and stores it in the `image_double` variable.
* The `im2uint8` function converts the double image in the `image_double` variable back to a uint8 image and stores it in the `image_uint8` variable.
### 3.2 Image Enhancement and Adjustment
#### 3.2.1 Image Brightness and Contrast Adjustment
Adjustments to image brightness and contrast can improve the visual appearance of an image. MATLAB provides the following functions for these adjustments:
- `imadjust`: Adjusts the brightness and contrast of an image.
- `brighten`: Increases or decreases the brightness of an image.
- `contrast`: Increases or decreases the contrast of an image.
**Code Block:**
```matlab
% Increasing image brightness
image_brightened = brighten(image, 0.2);
% Decreasing image contrast
image_contrasted = contrast(image, 0.5);
```
**Logical Analysis:**
* The `brighten` function increases the brightness of the image in the `image` variable by 0.2 and stores the result in the `image_brightened` variable.
* The `contrast` function decreases the contrast of the image in the `image` variable by 0.5 and stores the result in the `image_contrasted` variable.
#### 3.2.2 Image Color Space Conversion
Image color space conversion refers to changing an image from one color space to another. MATLAB provides the following functions for conversion:
- `rgb2gray`: Converts an RGB image to a grayscale image.
- `gray2rgb`: Converts a grayscale image to an RGB image.
- `hsv2rgb`: Converts an HSV image to an RGB image.
**Code Block:**
```matlab
% Converting an RGB image to grayscale
image_gray = rgb2gray(image);
% Converting a grayscale image to an RGB image
image_rgb = gray2rgb(image_gray);
```
**Logical Analysis:**
* The `rgb2gray` function converts the RGB image in the `image` variable to a grayscale image and stores it in the `image_gray` variable.
* The `gray2rgb` function converts the grayscale image in the `image_gray` variable back to an RGB image and stores it in the `image_rgb` variable.
# 4. Advanced Operations in Image Processing
### 4.1 Image Segmentation and Edge Detection
#### 4.1.1 Image Segmentation ***
***mon image segmentation algorithms include:
- **Threshold-based segmentation**: Divides image pixels based on their grayscale or color values into different regions.
- **Region-based segmentation**: Clusters image pixels into regions with similar features, such as color, texture, or shape.
- **Edge-based segmentation**: Segments objects by detecting edges in the image.
- **Graph-based segmentation**: Represents the image as a graph and uses graph theory algorithms to segment the image.
#### 4.1.2 Edge Detection Algorithms
E***mon edge detection algorithms include:
- **Sobel operator**: Uses a first-order derivative operator to detect edges.
- **Canny operator**: Smooths the image with a Gaussian filter and then detects edges using the Sobel operator.
- **Laplacian operator**: Uses a second-order derivative operator to detect edges.
### 4.2 Image Feature Extraction and Matching
#### 4.2.1 Image Feature Ext***
***mon image feature extraction methods include:
- **Color histogram**: Counts the number of pixels of different colors in an image.
- **Texture features**: Describe the texture characteristics of an image, such as texture energy and texture direction.
- **Shape features**: Describe the shape of objects in an image, such as area, perimeter, and shape factor.
#### 4.***
***mon image matching algorithms include:
- **Correlation matching**: Calculates the correlation between local areas of two images.
- **Feature matching**: Extracts image features and then matches based on feature similarity.
- **Geometric transform matching**: Deforms one image to match another using geometric transformations.
**Code Examples:**
```matlab
% Image segmentation example
I = imread('image.jpg');
segmentedImage = imsegment(I);
imshow(segmentedImage);
% Edge detection example
I = imread('image.jpg');
edges = edge(I, 'canny');
imshow(edges);
% Feature extraction example
I = imread('image.jpg');
features = extractHOGFeatures(I);
% Feature matching example
I1 = imread('image1.jpg');
I2 = imread('image2.jpg');
[matchedFeatures, matchedPoints1, matchedPoints2] = matchFeatures(I1, I2);
```
**Code Logical Analysis:**
- Image segmentation example: The `imsegment` function performs region-based segmentation on the image.
- Edge detection example: The `edge` function performs Canny edge detection on the image.
- Feature extraction example: The `extractHOGFeatures` function extracts Histogram of Oriented Gradient features from the image.
- Feature matching example: The `matchFeatures` function matches features based on their similarity between two images.
# 5. Applications of MATLAB Image Processing
### 5.1 Medical Image Processing
Medical image processing is a significant application area of MATLAB image processing, utilizing image processing techniques to analyze and process medical images, aiding in medical diagnosis and treatment.
#### 5.1.1 Medical Image Segmentation
Medical image segmentation involves separating different tissues or structures in medical images into distinct regions. It is crucial for medical image analysis and can be used for:
- **Lesion detection**: Identifying and locating tumors, lesions, and other abnormal areas.
- **Organ measurement**: Measuring the volume, shape, and other parameters of organs.
- **Surgical planning**: Providing detailed anatomical views for surgical procedures.
MATLAB provides various image segmentation algorithms, including:
- **Region growing**: Starting from seed points, gradually adding adjacent pixels to the region.
- **Threshold segmentation**: Dividing the image into different regions based on pixel grayscale values.
- **Edge detection**: Detecting edges in the image and segmenting regions along these edges.
#### 5.1.2 Medical Image Diagnosis
Medical image processing can also assist in medical diagnosis by analyzing features in medical images to identify diseases or abnormalities. For example:
- **Cancer detection**: Analyzing CT or MRI images to detect tumors or lesions.
- **Heart disease diagnosis**: Analyzing cardiac ultrasound images to assess cardiac structure and function.
- **Bone disease diagnosis**: Analyzing X-ray images to detect fractures, osteoporosis, and other diseases.
MATLAB provides tools for image enhancement, feature extraction, and classification, which can be used to build medical image diagnostic systems.
### 5.2 Remote Sensing Image Processing
Remote sensing image processing is another significant application area of MATLAB image processing, utilizing remote sensing images captured by satellites or aircraft for analysis and processing to extract surface information.
#### 5.2.1 Remote Sensing Image Classification
Remote sensing image classification involves categorizing the pixels in remote sensing images into different physical categories. It is crucial for remote sensing applications and can be used for:
- **Land use classification**: Identifying and classifying different types of land use, such as forests, farmland, urban areas, etc.
- **Crop monitoring**: Monitoring the growth and health of crops.
- **Disaster assessment**: Assessing the damage caused by natural disasters, such as floods, earthquakes, etc.
MATLAB provides various image classification algorithms, including:
- **Support Vector Machines (SVM)**: A supervised learning algorithm that can be used for classifying remote sensing images.
- **Random Forest**: An ensemble learning algorithm that can improve classification accuracy.
- **Neural Networks**: A deep learning algorithm that can handle complex remote sensing images.
#### 5.2.2 Remote Sensing Image Interpretation
Remote sensing image interpretation involves extracting surface information by analyzing features in remote sensing images. It is crucial for remote sensing applications and can be used for:
- **Geological survey**: Identifying and locating geological structures and mineral resources.
- **Environmental monitoring**: Monitoring changes in the environment, such as water pollution, deforestation, etc.
- **Urban planning**: Planning urban development and optimizing land use.
MATLAB provides tools for image enhancement, feature extraction, and spatial analysis, which can be used to build remote sensing image interpretation systems.
# 6. MATLAB Image Processing Toolbox
### 6.1 Introduction to the Image Processing Toolbox
The Image Processing Toolbox is a professional toolbox in MATLAB for image processing. It offers a series of powerful functions and algorithms covering all aspects of image processing, including image reading, display, conversion, enhancement, segmentation, feature extraction, and matching.
### 6.2 Commonly Used Functions in the Image Processing Toolbox
The Image Processing Toolbox includes a rich set of functions for performing various image processing tasks. Here are some commonly used ones:
- **imread()**: Reads image files.
- **imshow()**: Displays images.
- **imresize()**: Adjusts image size.
- **im2gray()**: Converts color images to grayscale.
- **imadjust()**: Adjusts image brightness and contrast.
- **imbinarize()**: Binarizes images.
- **edge()**: Detects image edges.
- **regionprops()**: Extracts image region properties.
- **matchFeatures()**: Matches image features.
### 6.3 Examples of Image Processing Toolbox Applications
The Image Processing Toolbox is widely applied across various fields, including:
- **Medical image processing**: Image segmentation, diagnosis, and analysis.
- **Remote sensing image processing**: Image classification, interpretation, and monitoring.
- **Industrial image processing**: Defect detection, quality control, and automation.
- **Computer vision**: Object detection, tracking, and recognition.
- **Scientific research**: Image analysis, data visualization, and modeling.
0
0
相关推荐
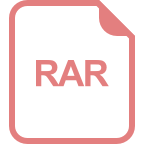
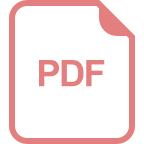
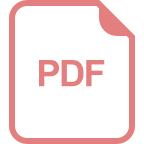
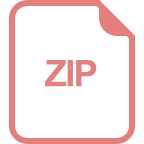
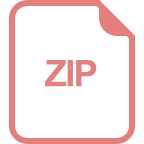
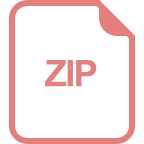
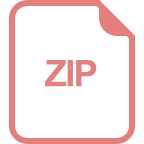
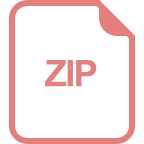
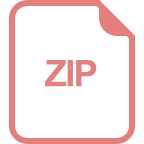