[Advanced] Pedestrian Detection in MATLAB Images: Using HOG+SVM for Pedestrian Detection
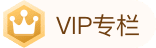
2.1 Histogram of Oriented Gradients (HOG) Features
The Histogram of Oriented Gradients (HOG) is a feature descriptor used to depict the distribution of gradient orientations in localized portions of an image. It involves the following steps:
- Image Tiling: Dividing the image into equal-sized, overlapping tiles.
- Gradient Calculation: Computing the gradient magnitude and direction for each pixel within each tile.
- Gradient Quantization: Quantizing gradient directions into a finite number of bins (e.g., 9 bins).
- Histogram Statistics: Counting the gradient magnitudes within each bin for every tile.
- Feature Vector Formation: Concatenating histograms from each tile to form a feature vector.
2. Theoretical Foundations of Image Pedestrian Detection
2.1 Histogram of Oriented Gradients (HOG) Features
2.1.1 The Process of Extracting HOG Features
HOG features are descriptors based on image gradient information, and their extraction process mainly includes the following steps:
- Image Preprocessing: Applying preprocessing operations such as grayscale conversion, normalization, and gamma correction to the input image to enhance robustness and reduce noise effects.
- Gradient Calculation: Using a Sobel operator or other gradient operators to compute the horizontal and vertical gradients of the image.
- Cell Division: Dividing the image into cells of equal size, typically 8x8 pixels.
- Gradient Histogram Calculation: Calculating the histogram of gradient magnitudes and directions within each cell, usually using 9 bins.
- Block Normalization: Grouping adjacent cells into blocks, typically 2x2 cells. Normalizing the histograms within each block to reduce the effects of lighting variations.
- Feature Vector Formation: Concatenating the normalized histograms of all blocks into a long vector to form the HOG feature vector.
2.1.2 Advantages and Limitations of HOG Features
The advantages of HOG features include:
*Robustness: Strong resilience to changes in lighting, noise, and cluttered backgrounds. *High Computational Efficiency: A relatively simple feature extraction process with high computational efficiency. *Strong Feature Expression: Effectively capturing the shape and texture information of pedestrians in images.
The limitations of HOG features are:
*Sensitivity to Deformation: The recognition effect of HOG features may decrease for pedestrians with significant deformations. *High Feature Dimensionality: HOG feature vectors usually have high dimensions, which could increase the training and testing time for classifiers.
2.2 Support Vector Machine (SVM) Classifier
2.2.1 Principles of SVM Classifier
SVM is a binary classification algorithm. Its fundamental principle is to map data points to a high-dimensional feature space and find a hyperplane that separates points of different classes. The selection of the hyperplane satisfies the following conditions:
*Maximizing Margin: The distance (margin) between the hyperplane and the nearest data points of different classes is maximized. *Support Vectors: Data points on the margin boundaries are called support vectors, which play a decisive role in the position of the hyperplane.
2.2.2 Parameter Selection for SVM Classifier
The performance of the SVM classifier is affected by the following parameters:
*Kernel Function: The kernel function determines the mapping method of data points in the feature space, with common kernel functions including linear, polynomial, and Gaussian kernels. *Penalty Parameter C: The penalty parameter controls the classifier’s tolerance for misclassification. A larger value of C leads to stricter classification but may cause overfitting. *Kernel Parameter γ (gamma): The kernel parameter γ controls the shape and range of influence of the kernel function. For the Gaussian kernel, a larger γ value results in a smaller range of influence.
Parameter selection is usually optimized through methods like cross-validation or grid search to achieve the best classification performance.
3. Image Pedestrian Detection in Practice with MATLAB
3.1 Image Preprocessing and HOG Feature Extraction
相关推荐







