MATLAB Genetic Algorithm Selection Guide: Matching Requirements, Optimizing Efficiency
发布时间: 2024-09-15 04:52:01 阅读量: 19 订阅数: 25 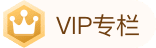
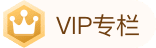
# 1. Genetic Algorithm Overview
A Genetic Algorithm (GA) is an optimization algorithm inspired by biological evolution, which simulates the process of natural selection to solve complex optimization problems. GA operates through the following steps:
1. **Initialize Population:** Randomly generate a set of candidate solutions, known as a population.
2. **Evaluate Fitness:** Calculate the fitness of each solution, measuring the extent to which it addresses the target problem.
3. **Selection:** Select the fittest solutions for reproduction based on their fitness.
4. **Crossover:** Combine two or more selected solutions to create new solutions.
5. **Mutation:** Randomly modify new solutions to introduce diversity.
6. **Repeat:** Repeat steps 2-5 until reaching a predetermined stopping condition (e.g., maximum number of generations or fitness convergence).
# 2. Parameter Selection for Genetic Algorithms
The performance of a Genetic Algorithm largely depends on the settings of its parameters. These parameters control various aspects of the algorithm, from population size to crossover and mutation probabilities. In this chapter, we will explore the most important parameters in Genetic Algorithms and discuss how to choose their optimal values.
### 2.1 Population Size and Generations
**Population Size** refers to the number of individuals in the population. The larger the population size, the greater the search space, increasing the likelihood of finding the optimal solution. However, a larger population size also increases the computational cost.
**Generations** refer to the number of iterations the algorithm runs. More generations allow the algorithm more time to evolve the population and find better solutions. But too many generations may lead to the algorithm getting stuck in local optima.
**Parameter Selection:**
***Population Size:** Typically between 50 and 1000. Larger population sizes can be used for more complex problems.
***Generations:** Typically between 100 and 1000. Fewer generations can be used for simpler problems.
### 2.2 Crossover and Mutation Probabilities
**Crossover** is an operation in genetic algorithms that generates new individuals by exchanging the genes of two parent individuals to create offspring. The crossover probability controls how frequently crossover occurs.
**Mutation** is another operation in genetic algorithms that generates new individuals by randomly altering the genes of an individual to create offspring. The mutation probability controls how frequently mutations occur.
**Parameter Selection:**
***Crossover Probability:** Typically between 0.5 and 1.0. Higher crossover probabilities create more diversity but may disrupt useful gene combinations.
***Mutation Probability:** Typically between 0.01 and 0.1. Higher mutation probabilities increase the chance of exploring the search space but may disrupt useful genes.
### 2.3 Selection Strategies
**Selection Strategy** determines which individuals are selected as the parents of the next generation. There are many different selection strategies, each with its own advantages and disadvantages.
**Common Selection Strategies:**
***Roulette Wheel Selection:** Individuals are selected based on their fitness values, with higher fitness individuals having a greater chance of being selected.
***Tournament Selection:** A small subset of individuals is randomly selected from the population, and the individual with the highest fitness value in this subset is chosen.
***Elitism Selection:** The best individuals from the population are always carried over to the next generation.
**Parameter Selection:**
***Selection Strategy:** Depends on the specific nature of the problem. Roulette wheel selection is suitable for problems with a larger population size, while tournament selection is suitable for problems with a smaller population size. Elitism is often used to prevent the population from losing useful genes.
**Code Example:**
```python
import random
def roulette_wheel_selection(population):
"""
Perform roulette wheel selection based on fitness values.
Parameters:
population: The population, where each element represents an individual.
Returns:
The selected individual.
"""
# Calculate total fitness
total_fitness = sum(individual.fitness for individual in population)
# Generate a random number between 0 and total fitness
random_value = random.uniform(0, total_fitness)
# Accumulate individual fitness values until the random value is exceeded
for individual in population:
random_value -= individual.fitness
if random_value <= 0:
return individual
# If no individual is found, return the last individual
return population[-1]
```
# 3. Genetic Algorithm Implementation
### 3.1 MATLAB Genetic Algorithm Toolbox
The MATLAB Genetic Algorithm Toolbox is a collection of tools specifically designed for genetic algorithms. It provides a variety of features, including:
- **Population Creation and Management:** `gapopulation`, `gareplace`
- **Crossover and Mutation Operations:** `crossover`, `mutation`
- **Selection Strategies:** `selection`
- **Fitness Functions:** `fitnessfcn`
- **Algorithm Control:** `gaoptimset`
**Example Code:**
```matlab
% Create a population
population = gapopulation(100, 10);
% Set crossover and mutation probabilities
options = g
```
0
0
相关推荐
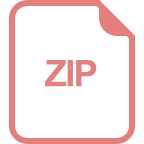
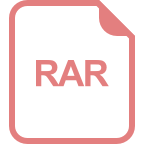
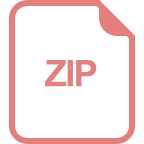
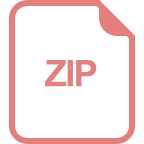
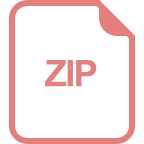
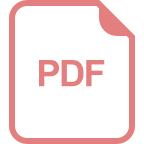
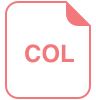
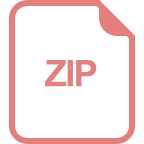
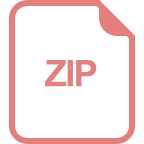