Application of MATLAB in Robot Control Systems: Modeling and Control Strategies
发布时间: 2024-09-15 01:12:33 阅读量: 29 订阅数: 30 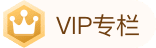
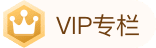
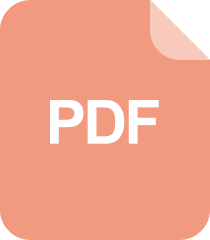
Robot Modeling and Control
# 1. Fundamental Applications of MATLAB in Robot Control Systems
## 1.1 Introduction to MATLAB and its Role in the Robotics Field
As an advanced numerical computing environment, MATLAB boasts powerful matrix manipulation capabilities and a wealth of toolboxes. Especially in the realm of robot control, it plays a crucial role. MATLAB not only assists engineers in performing complex mathematical computations and simulation verification but also can quickly transform theoretical models into practical applications, significantly shortening the R&D cycle.
## 1.2 Basic Functions of MATLAB in Robot Control
In the fundamental applications of robot control systems, MATLAB primarily undertakes several core functions:
- **System Modeling**: Using MATLAB's Simulink module, it is convenient to construct the mathematical model of a control system.
- **Algorithm Simulation**: Through writing scripts or functions, MATLAB can simulate and test various control algorithms, such as PID control, and more complex advanced control strategies.
- **Performance Analysis**: MATLAB's built-in analysis tools and visualization features can quickly display the dynamic response and performance indicators of a control system.
Through in-depth discussion in this chapter, we will gradually uncover the mysterious veil of MATLAB's fundamental applications in robot control systems and lay a solid foundation for subsequent explorations of more complex applications such as dynamic modeling, control algorithm design, and path planning.
# 2. Implementation of Robot Dynamic Models in MATLAB
## 2.1 Basic Applications of MATLAB in Modeling
### 2.1.1 Theoretical Foundation of Modeling and Overview of MATLAB Toolboxes
Robot dynamics is the science that studies the interaction forces and torques between the joints and links of a robot. In MATLAB, we can use multiple toolboxes to implement complex dynamic models, including the Robotics Toolbox, Simulink, Simscape, etc. These toolboxes provide a rich set of functions and graphical interfaces, which can help researchers and engineers quickly build a robot's dynamic model, perform dynamic simulation, control strategy design, and verification.
The Robotics Toolbox was developed by Professor Peter Corke; it is a collection of MATLAB functions and classes for robot modeling, simulation, and visualization. It includes tools for defining robot models, drawing robots, and computing inverse kinematics.
When modeling in practice, physical parameters such as mass, length, and friction coefficients must first be defined. Then, models are established using functions provided by the toolboxes, defining the type of joints (such as revolute joints, prismatic joints) and the properties of links. For complex dynamic analysis, MATLAB's Simulink provides a more advanced simulation environment capable of simulating more complex dynamic systems.
```matlab
% Example: Using the Robotics Toolbox to define a simple two-link planar robot model
L1 = Link('d', 0, 'a', 1, 'alpha', 0);
L2 = Link('d', 0, 'a', 1, 'alpha', 0);
robot = SerialLink([L1 L2], 'name', 'TwoLinkRobot');
robot.plot([0.5*pi 0.5*pi]); % Draws the robot image
```
The above code defines a two-link robot model and draws its image. The construction of the robot model is the prerequisite for performing dynamic simulation.
### 2.1.2 Derivation of Dynamic Equations and MATLAB Representation Methods
The derivation of dynamic equations is typically based on Newton-Euler equations or Lagrange's equations. The Newton-Euler method is more suitable for intuitive force and acceleration analysis, while the Lagrange method provides a more general and elegant approach to building dynamic models.
In MATLAB, we can use the `gravity` and `inertia` functions in the Robotics Toolbox to calculate the system's gravity and inertia matrix and then use the `fdyn` or `rne` functions to calculate the system's dynamic equations. These functions are algorithmic implementations based on Lagrange's equations.
The dynamic equations are represented as:
\[ M(q)\ddot{q} + C(q, \dot{q})\dot{q} + G(q) = \tau \]
where \( M(q) \) is the inertia matrix, \( C(q, \dot{q}) \) is the Coriolis force and centrifugal force matrix, \( G(q) \) is the gravity term, \( \tau \) is the joint torque or force.
```matlab
% Example: Calculate and display the inertia matrix and gravity term of the robot model
q = [0; 0]; % Configuration of the robot joint angles
tau = robot梯队*ones(2,1); % Simulate an input joint torque
M = robot.inertia(q);
G = robot.gravity(q);
[C, Cd] = robot.coriolis(q, ones(2,1));
fprintf('Inertia Matrix (M):\n');
disp(M);
fprintf('Gravity Term (G):\n');
disp(G);
```
This code calculates the robot's inertia matrix and gravity term. Since MATLAB's Robotics Toolbox is optimized for symbolic computation, engineers can directly obtain different parts of the dynamic equations through function calls. Thus, more attention can be focused on the design of control strategies.
## 2.2 Robot Dynamic Simulation in MATLAB
### 2.2.1 Using Simulink for Dynamic Simulation
Simulink provides a graphical interactive environment for dynamic simulation, allowing users to build complex dynamic system models and run simulations on these models. In Simulink, dynamic simulation can be achieved by drawing system block diagrams. Users can convert the dynamically calculated equations directly into block diagrams in Simulink models, achieving intuitive system simulation.
The "SimMechanics" module in Simulink is specifically designed for dynamic simulation of mechanical systems. By dragging and dropping different blocks, such as "Body", "Joint", "Force", etc., users can build complex robot models and conduct dynamic analysis.
In "SimMechanics", each component of the model has corresponding parameter inputs, such as mass, inertia tensor, coordinate system, etc. These parameters can be set according to the characteristics of the real robot. After creating the dynamic simulation model, simulation can be started through the "Simulation" menu's "Start Simulation".
```matlab
% Example: Create a simple dynamic simulation model in Simulink
simulinkModel = 'TwoLinkRobotSimulink';
open_system(simulinkModel);
sim(simulinkModel); % Start simulation run
```
The above code assumes that we have already created a Simulink model named "TwoLinkRobotSimulink", which contains all the necessary mechanical components and parameter settings, and then启动仿真运行 through the sim function.
### 2.2.2 Optimization and Verification of Dynamic Parameters
After completing the dynamic simulation, it is necessary to verify the accuracy of the simulation results and optimize the model parameters to ensure that the simulation results match the performance of the real system. This usually involves comparing experimental data with simulation data and adjusting model parameters.
Parameter optimization can be performed using MATLAB's `fmincon`, `ga`, and other optimization functions, as well as Simulink's Parameter Estimation tool. By defining one or more performance indicators (e.g., minimizing errors), we can automatically adjust the model parameters to make the simulation data approach the experimental data.
```matlab
% Example: Using the fmincon function for parameter optimization
% Assuming we have a cost function cost_function, which contains parameters to be optimized param
% Initial parameters
initial_params = [1, 1]; % Example parameters
% Use fmincon for optimization
options = optimoptions('fmincon', 'Display', 'iter', 'Algorithm', 'interior-point');
opt_params = fmincon(@cost_function, initial_params, [], [], [], [], [], [], [], options);
% Update model parameters
robot.param = opt_params;
% Resimulate
sim(simulinkModel);
```
In this code, we define a cost function `cost_function`, which returns the error between the model output and experimental data. Through the `fmincon` function, we attempt to find a set of parameters that can minimize this cost function. Once the optimal parameters are found, the robot model is updated, and the simulation is restarted to verify the optimization effect.
## 2.3 Integration of MATLAB and Robot Control Algorithms
### 2.3.1 Implementation of Common Control Algorithms in MATLAB
In robot dynamic simulation, the design and implementation of control algorithms are key. MATLAB provides a wide range of functions and toolboxes to implement various control algorithms, including PID control, adaptive control, robust control, etc. These control algorithms can be used to adjust the joint torques of the robot model to achieve the desired motion state.
For example, we can use the `pid` function in MATLAB to design a simple PID controller with three control actions: proportional, integral, and derivative. By adjusting the parameters of the PID controller (proportional gain P, integral gain I, derivative gain D), we can achieve precise control of the robot.
```matlab
% Example: Using MATLAB's PID controller to control the joint position of a robot
% Define the PID controller
Kp = 10; % Proportional gain
Ki = 0.1; % Integral gain
Kd = 0.01; % Derivative gain
controller = pid(Kp, Ki, Kd);
% Assume we have a desired joint position target_position and an actual position current_position
% Calculate error
error = target_position - current_position;
% Calculate the controller output
control_signal = controller(error);
% Apply the control signal to the robot model
robot.addTorque(control_signal);
% Simulate
sim(simulinkModel);
```
In this code, we create a PID controller and calculate the control signal, then apply this signal to the robot model for simulation. By continuously adjusting the PID parameters, we can control the robot to reach the desired
0
0
相关推荐
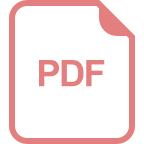
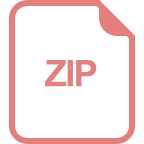
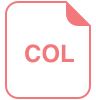
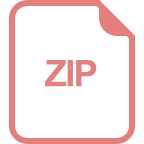
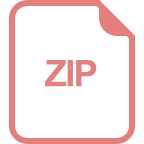
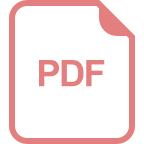
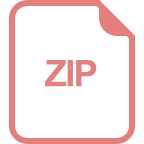
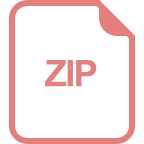