"Introduction to MATLAB Control System Design": Master the Basics and Practical Skills Quickly
发布时间: 2024-09-15 00:27:55 阅读量: 34 订阅数: 30 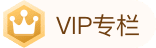
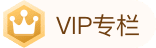
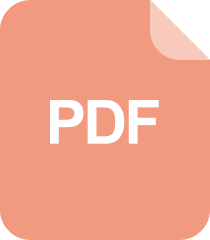
Xcode Treasures: Master the Tools to Design, Build, and Distribute Great Apps
# 1. Introduction to Control System Design with MATLAB
MATLAB, an abbreviation for Matrix Laboratory, is a significant tool in the field of control system design. It offers a suite of engineering computation, visualization, and programming capabilities. The software is particularly well-suited for the design, simulation, and analysis of control systems, thanks to its powerful built-in algorithm library and toolboxes.
In control system design, MATLAB's primary applications include, but are not limited to:
- **System Modeling**: Ability to establish mathematical models for both linear and nonlinear control systems.
- **System Analysis**: In-depth analysis of system stability and performance.
- **Controller Design**: Assisting engineers in designing PID controllers, state feedback controllers, etc.
- **System Simulation**: Testing the performance and stability of control systems through simulation.
This chapter will provide a preliminary introduction to the applications of MATLAB in control system design, laying the foundation for more in-depth analysis and applications in subsequent chapters. We will begin exploring the basic theories and mathematical tools of MATLAB, as well as how to use these tools to complete the workflow of system modeling, analysis, and controller design.
# 2. Basic Theories and Mathematical Tools in MATLAB
## 2.1 Mathematical Computation Foundations in MATLAB
### 2.1.1 Linear Algebra Operations
MATLAB's capabilities in linear algebra are powerful and indispensable for designing control systems. It provides a series of linear algebra functions that support basic matrix operations, such as matrix creation, transposition, inversion, eigenvalue decomposition, and singular value decomposition.
**Matrix Creation**:
Creating a matrix is the first step in performing linear algebra operations. In MATLAB, matrices can be created through direct assignment or by using specific functions like `zeros`, `ones`, `eye`, etc., to create matrices with particular element values.
```matlab
A = [1, 2; 3, 4]; % Creating a 2x2 matrix
B = zeros(3, 3); % Creating a 3x3 zero matrix
```
**Matrix Transposition**:
Matrix transposition is the operation of swapping all rows and columns of a matrix, which can be achieved using the single quote `'` operator.
```matlab
A = [1, 2; 3, 4];
A_transpose = A'; % Transposed matrix
```
**Matrix Inversion**:
For square matrices, if an inverse exists, it can be found using the `inv` function.
```matlab
A = [1, 2; 3, 4];
A_inv = inv(A); % Inverse matrix
```
Other linear algebra operations on matrices include finding the trace, determinant, etc., and MATLAB has corresponding functions like `trace` and `det` to perform these operations.
### 2.1.2 Calculus Operations
MATLAB is equally powerful in calculus operations, supporting functions for limits, derivatives, integrals, and numerical solutions to differential equations.
**Derivative Calculation**:
MATLAB's Symbolic Math Toolbox provides functionality for symbolic differentiation of expressions.
```matlab
syms x;
f = x^2;
df = diff(f, x); % Derivative of f with respect to x
```
**Integral Operations**:
Similar to derivatives, integral operations can also be accomplished using the Symbolic Math Toolbox.
```matlab
int_f = int(f, x); % Indefinite integral of f with respect to x
```
**Numerical Integration**:
For integrals that require numerical solutions, MATLAB provides the `integral` function.
```matlab
fun = @(x) x.^2;
integral_value = integral(fun, 0, 1); % Integrating from 0 to 1
```
### 2.1.3 Fourier Analysis and Signal Processing
MATLAB includes toolboxes for Fourier analysis, supporting operations such as Fast Fourier Transform (FFT), Inverse Fast Fourier Transform (IFFT), filter design, and signal convolution.
**Fast Fourier Transform**:
FFT is an essential tool for analyzing frequency components in signal processing. MATLAB provides the `fft` function to compute the FFT of a signal.
```matlab
x = [1, 2, 3, 4];
X = fft(x); % Computing the FFT of x
```
**Signal Filtering and Convolution**:
Filter design and signal convolution are critical in the field of signal processing. MATLAB's Signal Processing Toolbox provides the corresponding functions.
```matlab
% Assuming there is a signal x and a filter coefficient h
y = filter(h, 1, x); % Using the filter function for convolution
```
## 2.2 MATLAB's Control System Toolbox
### 2.2.1 Toolbox Function Introduction
MATLAB's Control System Toolbox offers a rich set of functions and graphical user interfaces for the design, analysis, and simulation of control systems. It supports various mathematical models, including transfer functions, state-space models, and discrete-time systems.
The toolbox includes modules for linear system analysis, controller design, and system simulation, enabling users to model, analyze, and design systems conveniently.
### 2.2.2 Modeling and Analysis of Control Systems
In terms of modeling, MATLAB provides methods for creating system models directly from transfer functions, zero-pole-gain, and state-space representations. Additionally, functions within the toolbox support model simplification and transformation for easier analysis and design.
```matlab
% Assuming there is a transfer function model G(s)
num = [1]; % Numerator coefficients
den = [1, 3, 2]; % Denominator coefficients
G = tf(num, den); % Creating a transfer function model
```
**Model Simplification**:
Model simplification involves converting complex models into more manageable forms for analysis. The `minreal` function in MATLAB can be used to simplify models.
```matlab
G_minreal = minreal(G); % Simplifying the G(s) model
```
### 2.2.3 Frequency Domain and Time Domain Analysis Methods
Frequency domain and time domain analysis are two important methods for analyzing control systems. MATLAB's Control System Toolbox offers functions for frequency domain and time domain analysis, such as step response analysis (`step`), impulse response analysis (`impulse`), and frequency response analysis (`bode`).
**Step Response Analysis**:
Step response analysis shows how a system reacts to a step input and is a crucial means for assessing system stability and dynamic performance.
```matlab
step(G); % Plotting the step response of G(s)
```
## 2.3 MATLAB's Graphical User Interface
### 2.3.1 Graphic Drawing and Editing
MATLAB offers powerful graphic drawing capabilities, suitable for creating various types of charts, such as two-dimensional line plots, three-dimensional surface plots, and scatter plots.
**Drawing Two-Dimensional Line Plots**:
Two-dimensional line plots are typically drawn using the `plot` function, which can easily display data points and connecting lines.
```matlab
x = [1, 2, 3, 4];
y = [1, 4, 9, 16];
plot(x, y); % Plotting points (1,1), (2,4), (3,9), (4,16)
```
### 2.3.2 Interactive Input and Data Visualization
In addition to basic graphic drawing, MATLAB supports interactive input, providing a more dynamic approach to data visualization. Users can select points directly on a graph using the `ginput` function or create custom controls using the `uicontrol` function.
```matlab
[x, y] = ginput(3); % User clicks three times on the graph to record the coordinates of the points
```
### 2.3.3 Interface Layout and Control Use
When designing graphical user interfaces, the `uicontrol` function enables the creation of various controls like buttons, sliders, and text boxes. Interface layout can be managed using MATLAB's layout managers, such as `uifigure` and `uipanel`.
```matlab
uicontrol('Style', 'pushbutton', 'String', 'Click Me', 'Callback', @button_callback);
```
This overview has covered some of the basic theories and tools involved in control system design with MATLAB. In the next chapter, we will delve into the modeling and simulation of control systems, including methods for creating, editing, and analyzing system models.
# 3. Control System Modeling and Simulation
The design and implementation of a control system is a complex process, often involving several stages from concept to practical application. Within the MATLAB environment, modeling and simulation are crucial steps in understanding and verifying the performance of control systems. This chapter will delve into how to conduct control system modeling and simulation with MATLAB, covering the creation and editing of system models, system response analysis, and system simulation and validation.
## 3.1 Creating and Editing System Models
Creating and editing control system models in MATLAB is a prerequisite for simulation and analysis. Control system models typically include forms such as transfer functions and state-space models. This section will discuss in detail the methods for creating these models and editing techniques.
### 3.1.1 Creating Transfer Functions and State-Space Models
#### Transfer Function Models
Transfer function models are commonly used to describe linear time-invariant systems in the frequency domain. In MATLAB, we can use the `tf` function to create transfer function models. For example, for a simple first-order system:
```matlab
num = [2]; % Numerator coefficients
den = [1 3 2]; % Denominator coefficients
sys_tf = tf(num, den); % Creating a transfer function model
```
Here, `num` is the array of coefficients for the numerator polynomial, and `den` is the array of coefficients for the denominator polynomial.
#### State-Space Models
State-space models provide a mathematical expression to describe a system's dynamic behavior over time. In MATLAB, state-space models can be created using the `ss` function:
```matlab
A = [-1]; % State matrix
B = [1]; % Input matrix
C = [1]; % Output matrix
D = [0]; % Direct transmission matrix
sys_ss = ss(A, B, C, D); % Creating a state-space model
```
In this example, `A`, `B`, `C`, and `D` represent the coefficient matrices of the state-space model, respectively.
### 3.1.2 Model Conversion and Simplification
In practical applications, there may be a need to convert a model from one form to another, such as converting a transfer function to a state-space model or simplifying a model for easier analysis. MATLAB provides tools and functions to support these operations.
#### Transfer Function to State-Space Model Conversion
```matlab
% Assuming sys_tf has been created
sys_ss_from_tf = tf2ss(sys_tf);
```
This function `tf2ss` converts a transfer function model into a state-space model.
#### Model Reduction
For high-order systems, reduced-order models are sometimes easier to analyze and simulate. MATLAB provides the `balred` function for balanced reduction of models:
```matlab
% Assuming sys_ss has been created
redOrder = 2; % Desired reduced model order
sys_ss_reduced = balred(sys_ss, redOrder);
```
The `balred` function reduces a model using the balanced truncation method.
### 3.1.3 Setting and Modifying Model Parameters
During a system's actual operation, it may be necessary to modify model parameters to adapt to new working conditions or performance requirements. MATLAB allows users to directly manipulate model parameters.
#### Modifying Transfer Function Parameters
```matlab
sys_tf_new = tf(num * 2, den); % Modifying the transfer function model by doubling the 'num' coefficient
```
#### Modifying State-Space Model Parameters
```matlab
sys_ss_new = ss(A, B, C, D * 2); % Modifying the state-space model by doubling the 'D' coefficient
```
In these examples, we change model parameters by directly modifying the coefficient arrays.
## 3.2 System Response Analysis
System response analysis refers to the dynamic response of a system to external inputs (such as step signals, impulse signals, etc.). This helps us understand the system's performance characteristics in both the time and frequency domains. This section will discuss how to perform system response analysis using MATLAB.
### 3.2.1 Step Response and Impulse Response Analysis
#### Step Response Analysis
Step response is one of the common methods for assessing the stability and performance of control systems. MATLAB's `step` function can be used to analyze step responses:
```matlab
figure; % Creating a graphic window
step(sys_tf); % Plotting the step response of the transfer function model
title('Step Response of Transfer Function Model');
```
#### Impulse Response Analysis
Impulse response is used to analyze the free dynamic characteristics of a system. MATLAB's `impulse` function performs impulse response analysis:
```matlab
figure; % Creating a graphic window
impulse(sys_tf); % Plotting the impulse response of the transfer function model
title('Impulse Response of Transfer Function Model');
```
### 3.2.2 Frequency Response Analysis
Frequency response analysis studies a system's response characteristics at different input frequencies. MATLAB's `bode` function can be used to analyze the frequency response characteristics of system models:
```matlab
figure; % Creating a graphic window
bode(sys_tf); % Plotting the frequency response of the transfer function model
title('Bode Plot of Transfer Function Model');
```
### 3.2.3 Steady-State and Transient Performance Evaluation
Steady-state performance refers to the stable state that the system ultimately reaches, while transient performance describes the dynamic process before reaching the steady state. In MATLAB, the step response and frequency response results can be combined to assess these performance indicators.
## 3.3 System Simulation and Validation
System simulation and validation are key aspects of control system design, allowing for the prediction and evaluation of system behavior under various conditions. This section will explore methods for conducting system simulation and validation with MATLAB.
### 3.3.1 Time-Domain Simulation Operations
Time-domain simulation focuses on the dynamic behavior of a system within a specific time frame. MATLAB's `lsim` function can be used for time-domain simulation:
```matlab
t = 0:0.01:10; % Defining a time vector
u = sin(2 * pi * t); % Defining an input signal (e.g., a sine wave)
figure; % Creating a graphic window
lsim(sys_tf, u, t); % Plotting the system's response to a specific input
title('Time Domain Simulation of Transfer Function Model');
```
### 3.3.2 Parameter Scanning and Sensitivity Analysis
Parameter scanning involves changing system model parameters to observe changes in system performance. MATLAB's `gensig` function can be used to generate different types of signals for simulation.
```matlab
% Assuming sys_tf has been created
% Scanning the 'D' parameter, changing from 0 to 1
D_params = 0:0.1:1;
for D_val = D_params
sys_tf_modified = tf(num, den, D_val); % Modifying the model parameters
step(sys_tf_modified); % Plotting the step response
hold on; % Retaining the current graphic to overlay new plots
end
title('Parameter Scan of Transfer Function Model');
```
### 3.3.3 Evaluation and Optimization of Simulation Results
Simulation results need to be evaluated and analyzed to determine if the system meets performance requirements. The evaluation process may involve calculating performance metrics and plotting performance charts. MATLAB's `stepinfo` function can be used to obtain performance metrics for step responses:
```matlab
info = stepinfo(sys_tf); % Getting the performance metrics of the step response of the transfer function model
disp(info); % Displaying the performance metrics
```
The optimization process typically involves adjusting model parameters or control strategies based on simulation results until the desired performance level is achieved. In this环节, MATLAB provides a robust environment that can integrate other toolboxes for more advanced optimization tasks.
Through this section's introduction, we can see how MATLAB offers powerful tools for creating, editing, analyzing, and validating control system models. This lays a solid foundation for the design and implementation of control systems and paves the way for subsequent stages of controller design and implementation.
# 4. Controller Design and Implementation
## 4.1 Theoretical Foundations of Controller Design
### 4.1.1 PID Control Theory
Proportional-Integral-Derivative (PID) control is one of the most common control strategies in industrial automation control systems. It calculates the system's error using proportional, integral, and derivative components and outputs a control signal to drive the controlled object to achieve stable control. The general form of a PID controller's transfer function is:
\[ C(s) = K_p + \frac{K_i}{s} + K_d s \]
where \(K_p\), \(K_i\), and \(K_d\) represent the proportional, integral, and derivative gains, respectively. The proportional component responds to the current error; the integral component eliminates steady-state errors; and the derivative component anticipates the trend of the error, aiding the system's rapid response.
In practical applications, adjusting PID parameters is often an empirical trial-and-error process. The PID Tuner tool in MATLAB can simplify this process. A critical step in PID controller design is parameter tuning, which involves adjusting \(K_p\), \(K_i\), and \(K_d\) to achieve optimal system performance.
### 4.1.2 State Feedback and Observer Design
State feedback control is an important method in modern control theory that uses the state information of the system for control. The design of state feedback control generally involves determining the state feedback gain matrix \(K\) and the state observer gain matrix \(L\). With these two gain matrices, precise control over system performance can be achieved.
In MATLAB, the `place` function can be used to calculate the state feedback gain \(K\) that places the closed-loop poles at desired locations, and the `acker` function can be used to design state observers. These methods help engineers design controllers that meet specific performance criteria.
### 4.1.3 Robust Control and Optimal Control Concepts
Robust control primarily studies the stability and performance of control systems in the presence of model uncertainties and external disturbances. The key to robust controller design is to ensure that the controller can maintain optimal performance in the face of these uncertainties.
Optimal control focuses on finding a control strategy that optimizes system performance metrics (e.g., minimizing energy consumption). In MATLAB, the `lqr` and `kalman` functions are used to design linear quadratic regulators and Kalman filters, respectively, which are common tools for implementing optimal control strategies.
## 4.2 MATLAB Controller Design Tools
### 4.2.1 PID Controller Design Tool
MATLAB offers a user-friendly PID Tuner tool that allows users to adjust PID controller parameters through a graphical interface directly. By integrating MATLAB with Simulink models, users can observe the effects of changes to controller parameters on system responses in real-time.
The typical steps for using the PID Tuner toolbox are as follows:
1. Open PID Tuner from the system model.
2. Use the graphical interface for quick tuning or automatic tuning of PID parameters.
3. Analyze the system response and make further adjustments as needed.
4. Apply the obtained PID parameters to a Simulink model for further testing.
### 4.2.2 State Space Controller Design
In MATLAB, state space controller design can utilize the `ss` function to create state space models and use functions like `acker`, `place`, or `kalman` to design controllers. This process usually requires a deep understanding of the system's dynamic characteristics and precise calculations and analysis of the state matrices.
### 4.2.3 Automatic Adjustment of Controller Parameters
MATLAB's Control System Toolbox provides several automatic tuning tools, such as `sisotool` and `pidtool`, which automate the manual parameter adjustment process. When using these tools, users can specify performance metrics (such as damping ratio, overshoot, etc.), and the toolbox will recommend a set of suitable controller parameters.
The automatic adjustment process generally involves the following steps:
1. Import or create a system's transfer function or state space model.
2. Start the automatic tuning tool and specify performance metrics.
3. The toolbox automatically calculates and recommends a suitable set of controller parameters.
4. Users can accept the recommended parameters or continue to make fine-tuning adjustments based on simulation results.
## 4.3 Comprehensive Practice of Control Systems
### 4.3.1 Modeling Cases of Actual Control Systems
In actual projects, modeling the control system is fundamental. Taking an inverted pendulum system as an example, the modeling process first requires establishing a mathematical model based on physical principles and then converting the mathematical model into an expression suitable for MATLAB processing.
```matlab
% Assuming the system is a simple second-order system
num = [1]; % Numerator coefficients
den = [1, 3, 2]; % Denominator coefficients
sys = tf(num, den); % Transfer function model
```
In the above code block, we use MATLAB's `tf` function to create a transfer function model. Although the process is simple, it lays the foundation for subsequent controller design and simulation.
### 4.3.2 Controller Design and Simulation Experiments
After designing a controller, an important step is to conduct simulation experiments to verify the controller's effectiveness. MATLAB provides a rich simulation environment, such as Simulink, which can intuitively build a system's simulation model and add controllers.
In the Simulink environment, we can build a system by dragging and dropping different modules and set simulation parameters to perform simulations. The results can show the system's response to a given input, and based on these responses, we can evaluate the controller's performance.
### 4.3.3 System Debugging and Performance Verification
Once simulation results are obtained, it is necessary to debug the system and verify its performance based on the results. In MATLAB, this can be done by adjusting controller parameters or adding correction networks (such as feedforward, feedback control, etc.) to improve performance.
```matlab
% Design a simple PID controller
Kp = 2.0; Ki = 5.0; Kd = 1.0;
controller = pid(Kp, Ki, Kd);
```
In the above code segment, we designed a simple PID controller and then added it to the Simulink model for further debugging and verification. Through iterative refinement, the system can ultimately achieve the desired performance metrics.
The above demonstrates the entire process from modeling to design, simulation experiments, as well as system debugging and performance verification in the MATLAB environment. Through comprehensive practice, engineers can ensure the robustness of control systems and meet the requirements of practical applications.
# 5. Advanced Applications of MATLAB in Control Systems
## 5.1 Nonlinear Control System Analysis
### 5.1.1 Characteristics and Modeling of Nonlinear Systems
A nonlinear control system refers to a system where there is a nonlinear relationship between inputs and outputs. The analysis and design of such systems are much more complex than linear systems, as they generally do not satisfy the superposition principle and homogeneity properties. Typical characteristics of nonlinear systems include saturation, dead zone, dead point, and relay characteristics, among others.
In the MATLAB environment, modeling nonlinear systems usually involves numerical methods, leveraging MATLAB's powerful numerical computing capabilities to simulate the dynamic behavior of nonlinear systems. Simulink provides a graphical modeling environment that allows engineers to quickly build models of nonlinear systems by dragging and dropping.
### 5.1.2 Phase Plane Analysis and Describing Function Method
Phase plane analysis is a method of studying the motion of nonlinear systems in a two-dimensional plane. It uses the system's differential equations to analyze the stability and motion characteristics of the system by drawing trajectories in the phase plane.
The describing function method is a tool for analyzing the steady-state response of nonlinear systems. The basic idea is to approximate a nonlinear element with a linear element that has a complex gain. By doing so, the response characteristics of nonlinear systems under specific inputs can be analyzed using linear system theory.
### 5.1.3 Lyapunov Stability Analysis
Lyapunov stability theory is a powerful tool for studying the stability of dynamic systems. It determines the stability of a system's equilibrium points by constructing a Lyapunov function (energy function). If the derivative of the Lyapunov function along the system's trajectory is negative, the system's equilibrium point is asymptotically stable.
In MATLAB, custom functions can be written to calculate the derivative of Lyapunov, thus verifying the stability of nonlinear systems. Using MATLAB's symbolic computation capabilities, complex mathematical operations can be simplified, making Lyapunov stability analysis practical for nonlinear control system design.
## 5.2 Multivariable Control System Design
### 5.2.1 Multivariable (MIMO) System Concept
In control system design, a multivariable (MIMO) system refers to a system with multiple inputs and multiple outputs. The analysis and design of these systems are much more complex than single-input single-output (SISO) systems, as they require simultaneous consideration of the interaction and coupling between multiple variables.
MATLAB provides various toolboxes for analyzing and designing MIMO systems, such as the Robust Control Toolbox and the Model Predictive Control Toolbox. These toolboxes include a series of functions and commands that can assist engineers in designing effective MIMO control strategies.
### 5.2.2 Decoupling Control Strategies and Design Methods
In MIMO systems, decoupling control is a common method to reduce the effects of internal cross-coupling within the system. It aims to ensure that each control input affects only the corresponding output variable, thus simplifying the control system's structure and reducing control complexity.
In MATLAB, matrix operations on transfer functions or state-space models can be used to calculate decoupling matrices and design corresponding decoupling controllers. By doing so, complex MIMO systems can be transformed into several independent SISO systems, making the design and implementation of control strategies more manageable.
### 5.2.3 Simulation and Analysis of Multivariable Control Systems
The simulation and analysis of multivariable control systems need to consider the stability and dynamic performance of the system comprehensively. MATLAB's simulation tools, such as Simulink, offer powerful multivariable simulation capabilities that enable rapid system model construction and dynamic response analysis.
When conducting multivariable control system simulations, engineers need to define appropriate performance metrics, such as minimizing response time, reducing overshoot, and improving robustness. MATLAB's optimization toolbox can help engineers find optimal controller parameters that meet these performance metrics.
## 5.3 Application of Modern Control Theory
### 5.3.1 Basics of Adaptive Control and Predictive Control
Adaptive control is a control strategy that can automatically adjust controller parameters based on system performance, suitable for dynamic systems with uncertainties. It can continuously learn and adapt during system operation to achieve good control results.
Predictive control is a model-based control strategy that can predict a system's future dynamic behavior and develop current control strategies based on these predictions. Predictive control is widely used in industrial processes because it can handle system delays, uncertainties, and constraints.
MATLAB provides design and simulation tools for adaptive control and predictive control, such as the Adaptive Control Toolbox and the Model Predictive Control Toolbox. With these tools, engineers can easily implement adaptive control and predictive control strategies.
### 5.3.2 Sliding Mode Variable Structure Control Theory
Sliding mode variable structure control (SMC) is a special type of nonlinear control method that designs a sliding hyperplane, allowing system states to slide onto the desired state trajectory. SMC has strong robustness and can cope with changes in system parameters and external disturbances.
MATLAB provides some specialized tools for the design and simulation of sliding mode variable structure control. With these tools, engineers can conveniently design SMCs and verify their performance through simulation.
### 5.3.3 Application Examples of MATLAB Toolboxes in Modern Control Theory
To better illustrate the application of MATLAB toolboxes in modern control theory, the following provides an example. Suppose we need to design a sliding mode controller to control a simple second-order nonlinear system.
First, we need to define the system's dynamic model:
```matlab
function dxdt = nonlinear_system(t, x)
% System state equations
dxdt = [x(2); -x(1) + x(1)^2 - x(1)*x(2)]; % A simple nonlinear system
end
```
Then, we define the sliding hyperplane for the SMC:
```matlab
function s = sliding_surface(x)
% Define the sliding hyperplane
s = [1, 0] * x; % A simple linear sliding hyperplane
end
```
Next, we use MATLAB's simulation capabilities to evaluate the controller's performance:
```matlab
% Initialize system state and controller parameters
x0 = [0.1; 0]; % Initial state
controller_params = [0.1, 1]; % Sliding mode controller parameters
% Run simulation
[t, x] = ode45(@(t, x) nonlinear_system(t, x), [0, 10], x0);
% Plot system state over time
plot(t, x);
xlabel('Time');
ylabel('State');
legend('x1', 'x2');
title('State Variables vs. Time');
```
By following these steps, we can simulate the dynamic response of a nonlinear system under a sliding mode controller and observe the system's stability and performance through graphics.
Through the above example, we can see the significant role MATLAB plays in the application of modern control theory. Whether it's adaptive control, predictive control, or sliding mode variable structure control, MATLAB provides corresponding toolboxes and functions to assist engineers in designing and implementing control strategies. These toolboxes greatly simplify complex mathematical computations, shorten design cycles, and improve design quality.
# 6. Application of MATLAB in Robotic Control Systems
In modern engineering and research fields, robotic control systems are one of the key technologies driving automation and intelligence. MATLAB, with its powerful computing capabilities, rich toolboxes, and modules, provides convenient conditions for the development of robotic control systems. This chapter will explore MATLAB's specific applications in robotic control systems, including dynamic modeling, motion control, path planning, and interface techniques with actual hardware.
## 6.1 Robotic Dynamic Modeling and Simulation
In the design of robotic control systems, accurate dynamic models are indispensable. MATLAB can establish robotic dynamic models based on Newton-Euler equations or Lagrangian equations and conduct simulation verification.
```matlab
% Example code: Using MATLAB for robotic dynamic modeling and simulation
% Define robot parameters and structure
robot = robotics.RigidBodyTree('example URDF file.urdf');
% Define initial and target states
q0 = [0; 0; 0; 0; 0; 0]; % Initial joint angles
q1 = [pi/2; pi/2; pi/2; pi/2; pi/2; pi/2]; % Target joint angles
tFinal = 5; % Simulation time
% Set simulation parameters
options = odeset('RelTol', 1e-3, 'AbsTol', 1e-3);
[t, q] = ode45(@(t, q) robotics.RigidBodyTree.RigidBodyDynamics('tree', robot, t, q), [0, tFinal], q0, options);
% Visualize the results
figure;
plot robot motion trajectory
```
## 6.2 Robotic Motion Control
Robotic motion control strategies include various advanced algorithms, such as inverse kinematics algorithms, PID control, and more sophisticated control strategies. MATLAB provides many ready-made algorithms and functions that can conveniently implement these control strategies.
```matlab
% Example code: Using MATLAB for inverse kinematics solution
% Define target pose
target_pose = [1 0 0 0 1 0 0];
% Solve for joint angles using inverse kinematics algorithm
q_inverse = robotics.InverseKinematics('RigidBodyTree', robot);
weights = [1 1 1 1 1 1];
config = q_inverse('preshape', target_pose, weights);
% Visualize the robot reaching the target position
robot.plot(q_inverse);
```
## 6.3 Robotic Path Planning
In the autonomous navigation and operation of robots, path planning is a core issue. MATLAB supports various path planning algorithms, such as A* algorithm, Rapidly-exploring Random Tree (RRT), and Probabilistic Roadmaps (PRM).
```matlab
% Example code: Using MATLAB for path planning
% Create an environment map and obstacles
map = robotics.BinaryOccupancyGrid(10,10,ones(10,10));
% Set start and goal points
start = [1 1];
goal = [10 10];
% Perform path planning using the A* algorithm
planner = robotics.PRM(map);
[pthObj, solnInfo] = plan(planner, start, goal);
% Visualize the planning results
show(planner);
```
## 6.4 MATLAB and Robotic Hardware Interface
In addition to providing simulation and algorithm design, MATLAB also supports interfaces with real robotic hardware. By directly participating in the robot's actual operations through real-time data acquisition and control command sending, MATLAB can be directly involved in the actual operation of the robot.
```matlab
% Example code: MATLAB controlling robotic hardware
% Initialize robot hardware interface
robot = robotics.Robot('RealRobot');
% Send control commands
cmd = struct('JointAngle', [pi/4, pi/4, pi/4, pi/4, pi/4, pi/4]);
robot.sendCommand(cmd);
% Read sensor data
sensor_data = robot.getSensorData();
```
The applications of MATLAB in robotic control systems are not limited to the few aspects mentioned above but also involve model validation, system debugging, performance optimization, and other aspects. These features and capabilities combined make MATLAB an ideal platform for the design and implementation of robotic control systems.
0
0
相关推荐
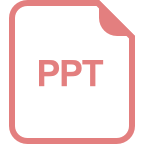
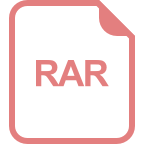
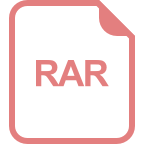
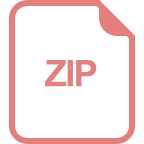
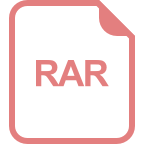
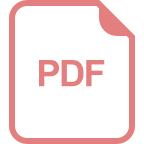
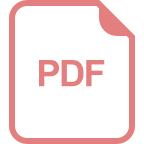
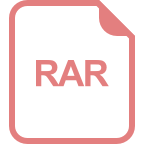