MATLAB-Based Fault Diagnosis and Fault-Tolerant Control in Control Systems: Strategies and Practices
发布时间: 2024-09-15 01:19:02 阅读量: 15 订阅数: 18 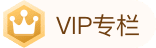
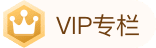
# 1. Overview of MATLAB Applications in Control Systems
MATLAB, a high-performance numerical computing and visualization software introduced by MathWorks, plays a significant role in the field of control systems. MATLAB's Control System Toolbox provides robust support for designing, analyzing, and simulating complex control systems. MATLAB offers a comprehensive system simulation environment, assisting engineers in designing control systems, diagnosing faults, and optimizing control strategies.
In this chapter, we first explore the foundational applications of MATLAB in control systems, including design and simulation. We then specifically analyze how MATLAB aids engineers in system modeling, controller design, and system performance evaluation. Furthermore, this chapter delves into MATLAB's applications in the development of advanced control systems, such as adaptive control and robust control.
To enrich the content, this chapter is based on a simple control system design case, demonstrating how to use MATLAB to build and simulate systems. Through practical examples, readers will understand MATLAB's practical applications in control systems and master basic operational skills.
# 2. Fundamental Theories of Control System Fault Diagnosis
### 2.1 Mathematical Models of Fault Diagnosis
#### 2.1.1 Mathematical Description of System State
In control systems, the system state is typically described by a set of state variables that reflect the system's dynamic characteristics at any given moment. Mathematically, these state variables along with the system's input and output constitute a state-space model. In discrete-time systems, the state-space model can be represented as:
```mermaid
graph TD
A[System Input u(k)] --> B[System State x(k+1)]
B --> C[System Output y(k)]
```
- **System Input u(k)**: External input at time step k.
- **System State x(k)**: Internal state of the system at time step k.
- **System Output y(k)**: The system's external manifestation at time step k.
The update of the system state can be represented by the following equation:
```math
x(k+1) = A(k) x(k) + B(k) u(k)
```
Where A(k) and B(k) are the system's dynamic matrix and input matrix, respectively, typically determined by the system's design parameters.
#### 2.1.2 Establishm***
***mon classifications include:
- **Hard Faults**: Faults that significantly degrade system performance, such as open circuits or short circuits.
- **Soft Faults**: Gradually developing faults, such as component aging or wear.
- **Intermittent Faults**: Irregularly occurring faults, such as intermittent open circuits caused by poor contact or vibrations.
When establishing fault models, either physics-based or data-driven approaches can be used. Mathematically, a fault model can be represented as:
```math
f(x, u, t) = d(t)
```
Where f represents the function affecting the system state due to a fault, and d(t) represents the fault state at time t.
### 2.2 Fault Detection Algorithms
#### 2.2.1 Statistical Detection Methods
Statistical detection methods are based on historical data from the system's normal operation to build statistical models for detecting anomalies. A commonly used method is residual analysis, which generates a residual sequence by comparing the system's actual output with the expected output.
Here is a pseudocode for residual generation:
```matlab
% Input data
U = ...; % System input matrix
Y = ...; % System output matrix
% System model parameters under normal operating conditions
A = ...;
B = ...;
% State-space model simulation
X = ...; % System state estimation
Y_est = ...; % Output estimation
% Calculate residuals
residuals = Y - Y_est;
% Residual analysis
mean_res = mean(residuals);
std_dev = std(residuals);
threshold = mean_res + k * std_dev; % k is a multiple of the standard deviation
% Determine if a fault has occurred
if any(abs(residuals) > threshold)
disp('Fault detected');
else
disp('System normal');
end
```
In practical applications, choosing an appropriate multiple of the standard deviation k and ensuring that the model accurately reflects the system's normal behavior are crucial.
#### 2.2.2 Model Reference Methods
Model reference methods involve comparing the actual system to an ideal reference model. A reference model is designed to simulate the ideal behavior of the system without faults, and then the actual system is compared to this reference to detect faults.
```mermaid
graph LR
A[Actual system output] --> B[Residual generation]
C[Reference model output] --> B
B --> D[Residual analysis]
D --> E[Fault detection]
```
#### 2.2.3 Application of Machine Learning Methods in Fault Detection
With the development of machine learning technology, machine learning methods are widely applied in the field of fault detection. Data-driven methods do not require precise mathematical modeling of the system but instead learn the normal behavior patterns of the system through training data.
For example, the process of fault detection using Support Vector Machines (SVM) can be summarized as:
```matlab
% Data preprocessing
data = ...; % Collected dataset
X = data(:, 1:end-1); % Feature variables
Y = data(:, end); % Label variables (normal/fault)
% Splitting the dataset into training and testing sets
cv = cvpartition(size(data, 1), 'HoldOut', 0.2);
idx = cv.test;
X_train = X(~idx, :);
Y_train = Y(~idx, :);
X_test = X(idx, :);
Y_test = Y(idx, :);
% Training the model
svmModel = fitcsvm(X_train, Y_train);
% Prediction and evaluation
Y_pred = predict(svmModel, X_test);
accuracy = sum(Y_pred == Y_test) / length(Y_test);
% Using the model for fault detection
if accuracy > threshold
disp('Model accurate, can be used for fault detection');
else
disp('Model requires further training');
end
```
### 2.3 Evaluation of Fault Diagnosis Techniques
#### 2.3.1 Measurement of Diagnostic Accuracy
Diagnostic accuracy is typically measured by indicators such as classification accuracy, recall rate, and F1 score. These indicators are commonly used performance evaluation standards in the field of machine learning. In fault diagnosis, these indicators help us assess the diagnostic algorithm's ability to identify faults.
```math
\text{Accuracy} = \frac{TP + TN}{TP + TN + FP + FN}
```
```math
\text{Recall} = \frac{TP}{TP + FN}
```
```math
\text{F1 Score} = 2 \times \frac{\text{Accuracy} \times \text{Recall}}{\text{Accuracy} + \text{Recall}}
```
Where TP represents true positives (correctly identified faults), TN represents true negatives (correctly identified normal states), FP represents false positives (incorrectly identified faults), and FN represents false negatives (undetected faults).
#### 2.3.2 Consideration of Real-time and Reliability
In control systems, the fault diagnosis system must be not only accurate but also具备 real-time capabilities and high reliability. Real-time capability means that the fault diagnosis system can respond quickly to fault events, while high reliability refers to maintaining stable and accurate diagnostic capabilities during long-term operation.
When designing fault diagnosis systems, the following factors should be considered:
- **Computational complexity of the diagnostic algorithm**: Whether the algorithm can run efficiently without affecting the real-time capabilities of the control system.
- **Update frequency of the diagnostic model**: Whether the model can adapt to system changes and maintain diagnostic accuracy.
- **Redundancy design of the system**: Ensuring that the system can continue to operate even if some parts fail.
For example, in real-time systems, a sliding window method can be used to process data to ensure the real-time capability of fault detection.
```matlab
% Pseudocode for sliding window processing
window_size = 100; % Define window size
data = ...; % Data stream
for i = 1:length(data)
if i < window_size
window_data(i) = data(i);
else
window_data = [data(i-window_size+1:i), window_data(1:end-1)];
end
% Execute fault detection algorithm within the window
...
end
```
Through these measures, we can ensure that the fault diagnosis system has the required real-time and reliability capabilities, providing stable and secure protection for the control system.
# 3. Theories and Implementation of Fault-tolerant Control Strategies
In control systems, fault-tolerant control strategies are crucial as they ensure that the system can maintain certain performance levels or even guarantee safe operation in the event of faults. This chapter will delve into the theoretical foundations of fault-tolerant control strategies and provide detailed instructions on how to implement these strategies using MATLAB.
## 3.1 Basic Concepts of Fault-tolerant Control
#
0
0
相关推荐
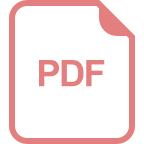
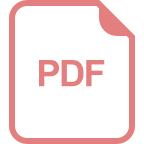
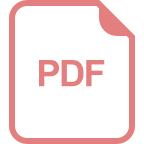





