【Stability Analysis of Control Systems in MATLAB】: Application of Eigenvalues and the Routh Criterion
发布时间: 2024-09-15 00:47:16 阅读量: 5 订阅数: 19 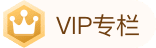
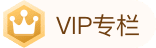
# 1. Fundamental Concepts of Control System Stability Analysis
## 1.1 The Concept of Stability in Control Systems
Stability is a central issue in control system design, determining whether the system can maintain its performance when subjected to external disturbances or changes in internal parameters. In practical applications, the stability of a control system directly affects the system's response speed, accuracy, and reliability.
## 1.2 The Importance of System Stability Analysis
Accurate analysis of system stability can predict system behavior and provide a theoretical basis for controller design. The results of stability analysis can be used to guide parameter adjustments, optimize system performance, and avoid potential risks and failures.
## 1.3 Classification and Judgment of System Stability
Control system stability is divided into three categories: static stability, dynamic stability, and marginal stability. Methods such as the Lyapunov method and the Routh criterion can be used to determine the stability state of the system. In the next chapter, we will delve into the application of MATLAB in control system stability analysis.
# 2. The Application of MATLAB in Control Systems
### 2.1 The Role of MATLAB in Control System Design
MATLAB is a high-performance numerical computing and visualization software with extensive applications in the field of control systems. MATLAB offers a wealth of toolboxes, especially the Control System Toolbox, which contains many functions for designing, analyzing, and simulating various control systems. This makes MATLAB an irreplaceable powerful tool in control system design.
### 2.2 Using MATLAB for Control System Modeling
In the design and analysis process of control systems, modeling is a crucial step. MATLAB makes it very convenient to define the system's transfer function, state-space representation, zero-pole configuration, and block diagram. Here is an example of using MATLAB for system modeling:
```matlab
% Define a transfer function
num = [1]; % Numerator polynomial coefficients
den = [1, 3, 2]; % Denominator polynomial coefficients
sys = tf(num, den); % Create a transfer function model
% Display system information
disp(sys);
```
### 2.3 System Analysis Tools in MATLAB
System analysis is another important aspect of control system design. The MATLAB Control System Toolbox provides a series of functions for time response analysis, frequency response analysis, and stability analysis, among others. For example, the `step()` function can be used to analyze the system's step response, or the `bode()` function can be used to analyze the system's frequency response. Below is a code example:
```matlab
% Perform step response analysis on the transfer function system defined above
figure;
step(sys);
title('System Step Response');
grid on;
```
### 2.4 Using MATLAB for Control System Simulation
Simulation plays a vital role in control system design, as it helps designers verify the effectiveness of their designs before actual construction and testing. Simulink, a MATLAB interactive simulation environment, allows users to build complex dynamic system models and conduct simulations. The following illustrates the process of building and simulating a simple Simulink model:
1. Open Simulink and create a new model.
2. Drag and drop the required modules from the Simulink library (e.g., input, transfer function, output, etc.).
3. Connect the modules to build the system model.
4. Configure simulation parameters and run the simulation.
5. Analyze the simulation results.
### 2.5 The Application of MATLAB in Control System Optimization
Control system design often involves optimization problems, such as finding the optimal controller parameters to meet specific performance criteria. MATLAB's Optimization Toolbox provides various optimization algorithms to solve such problems. For instance, the `fmincon()` function can be used to find the solution to a minimization problem under certain constraint conditions. Below is a simple example of setting up an optimization problem:
```matlab
% Set up an optimization problem: Minimize the cost function while meeting system performance criteria
% Define the objective function (cost function)
cost_function = @(x) (x(1) - 1)^2 + (x(2) - 2)^2;
% Define inequality constraints
A = []; b = [];
Aeq = []; beq = [];
lb = [0, 0]; % Lower bounds for parameters
ub = [10, 10]; % Upper bounds for parameters
% Use the fmincon function for optimization
x0 = [0, 0]; % Initial guess
options = optimoptions('fmincon','Display','iter','Algorithm','sqp');
[x, fval] = fmincon(cost_function, x0, A, b, Aeq, beq, lb, ub, [], options);
% Display the optimal solution
disp(['Optimal solution: x = ', num2str(x)]);
disp(['Optimal cost: f(x) = ', num2str(fval)]);
```
### 2.6 MATLAB and New Developments in Control System Research
MATLAB is constantly evolving, introducing many new tools and features to support the latest research in control systems. For example, the MATLAB R2021a version introduced the Fuzzy Logic Toolbox, used for designing and simulating fuzzy logic control systems. This provides more possibilities for advanced research and practical applications in control systems. Additionally, the active MATLAB community has promoted the development of various open-source toolboxes, such as the Robotics System Toolbox, which expands the applications of MATLAB in the field of control systems.
Through the content of the above chapters, we can see the important applications and powerful functions of MATLAB in control system design and analysis. In the subsequent content of this chapter, we will further explore some advanced applications of MATLAB in control system analysis and simulation.
# 3. Theory and Practice of the Eigenvalue Analysis Method
## 3.1 Basic Concepts of Eigenvalues
### 3.1.1 Definition of Eigenvalues
The eigenvalue analysis method is a fundamental method in control system stability analysis, primarily relying on the roots of the system characteristic equation to determine the system's stability state. The definition of eigenvalues originates from the concept of eigenvalues in linear algebra and refers to the scalar λ that, when multiplied by the system matrix A, keeps the vector x unchanged, satisfying the equation (A - λI)x = 0. Here, I is the identity matrix, and x is a non-zero vector. Expanding this equation yields an n-th order polynomial, the solutions of which are the roots of the characteristic equation.
### 3.1.2 Eigenvalues and System Stability
The stability of a system can be judged by the distribution of eigenvalues. For a continuous-time linear time-invariant system (LTI), if all eigenvalues of the system matrix A have negative real parts, the system is stable. In other words, the system can produce bounded output for any bounded input, and will not diverge or oscillate. Conversely, if at least one eigenvalue has a positive real part, the system is unstable. In practical applications, analyzing eigenvalues can help engineers understand the behavior of the system under specific conditions, such as overshoot, oscillation frequency, and damping degree.
## 3.2 Calculation Methods for Eigenvalues
### 3.2.1 Using MATLAB to Solve Eigenvalues
In the MATLAB environment, we can use the `eig` function to calculate the eigenvalues and eigenvectors of a matrix. This is very useful for analyzing the system's eigenvalues. Suppose we have a system matrix A; we can use the following MATLAB code to solve for its eigenvalues:
```matlab
A = [0 -1; 1 -2];
lambda = eig(A);
disp(lambda);
```
After executing this code, the `lambda` variable will store the eigenvalues of matrix A, which are the system's eigenvalues. When conducting system stability analysis, if the real parts of the eigenvalues are all negative, the system is stable. Otherwise, the system is unsta
0
0